ABSTRACT
Background
Breast cancer is the most commonly diagnosed cancer and the second most prominent cause of death in women. Owing to its intricate nature involving multiple cell types, preventing its onset is challenging. Tyrosine kinase plays a crucial role in cancer development and PTK6, a non-receptor kinase, is recognized as a prognostic biomarker for cancer. Consequently, inhibition of PTK6 has emerged as a potential strategy to curb breast cancer cell proliferation and survival. Dasatinib, an oral SRC-family kinase inhibitor, exhibits robust anti-proliferative, anti-metastatic, and anti-osteoclastic properties, demonstrating sensitivity in triple-negative or basal-like breast cancer cell lines.
Materials and Methods
In this context, our study aimed to identify potent lead compounds against breast cancer by leveraging dasatinib derivatives in the pursuit of robust inhibitors. Our methodology encompassed various parameters, including derivative construction by the online tool MolOpt which is freely available and widely used. Further molecular docking, ADME analysis, PASS prediction, rodent toxicity assessment, potential adverse effect evaluation, and MD with PCA calculations were investigated.
Results
Through meticulous analysis, we compiled a substantial dataset of 163 derivatives from which the top two compounds compound 1 and compound 10 emerged following rigorous parameter evaluation.
Conclusion
After filtration, the identified candidates underwent further scrutiny via MD and PCA analyses which helped to ascertain their potential as promising drug-like substances capable of inhibiting breast cancer effectively.
among women globally, with 7.8 million cases in the past five years. Breast cancer encompasses a heterogeneous group of malignancies with varying characteristics such as histological features, gene expression patterns, metastatic potential, and mortality rates.4,5 It ranks second in cancer-related mortality, presenting symptoms such as breast lumps, changes in breast size, and skin dimpling. The complexity of its developmental pathways and resistance mechanisms underscores the importance of elucidating the molecular drivers of breast tumors. Advanced age is a primary risk factor, along with gene mutations (e.g., BRCA1, BRCA2, TP53), family history, early menarche, late menopause, delayed childbirth, and hormone replacement therapy.6 Chemotherapy and radiation therapy are commonly used treatments, especially in Breast-Conserving Therapy (BCT) and surgery.7 However, their effectiveness diminishes in later stages of cancer development. These treatments also suffer from drawbacks such as adverse side effects due to the lack of selectivity of available anticancer drugs and the development of resistance in certain breast cancer variants.8–10
Protein Tyrosine Kinase 6
Protein Tyrosine Kinases (PTKs) play a crucial role in multiple cancer pathways by phosphorylating tyrosine residues in the target proteins. Abnormal PTK signaling is frequently associated with colorectal cancer.11,12 PTKs are separated into Receptor Tyrosine Kinases (RTKs) & Non-Receptor Tyrosine Kinases (NRTKs). RTKs activate various signaling pathways at the cell surface, whereas NRTKs function intracellularly.13 PTK6, also known as Breast tumor Kinase (BRK), is an intracellular signal transducer in epithelial tissues.14,15 It influences the pathogenesis of breast and prostate cancer. PTK6 was first identified in melanocytes and was subsequently cloned from metastatic breast tumors and the gut.16 It is expressed in both normal and tumorous tissues and promotes cell proliferation, survival, and carcinogenesis. Recent research suggests that PTK6 operates independently of kinases and performs context-specific functions in carcinomas. It interacts with ERBB2 (HER2) and other receptor tyrosine kinases such as EGFR and MET.17 PTK6 inhibitors have shown promise in inhibiting breast cancer cell migration and invasion.18,19
Inhibiting PTK6
Currently, various medications targeting PTK6 are commonly prescribed in clinical settings. Notably, dasatinib, a small-molecule inhibitor, is predominantly utilized for the treatment of chronic myeloid leukemia in addition to its role in inhibiting PTK6 activity.20–22 Structural-based virtual screening has emerged as a promising approach for identifying potential drug candidates for breast cancer treatment.23,24
Therefore, the investigation aimed to utilize high-throughput screening to identify small-molecule inhibitors of PTK6 using structure-based drug design. By leveraging bioinformatics tools and databases, we aimed to develop derivatives that effectively target PTK6 with minimal side effects, offering better and safer control of breast carcinoma. Our investigation utilizes the crystal structure of the PTK6 kinase domain complexed with dasatinib as a basis for this endeavor.Top of Form
MATERIALS AND METHODS
Extraction and preparation of protein
The 5H2U (PTK6) protein structure, with a resolution of 2.24 Angstroms, was obtained from the Protein Data Bank in PDB format, which is accessible through rcsb.org/.25,26 The macromolecule production procedure involved removing water particles and other heteroatoms using the BIOVIA Discovery Studio Visualizer 2021.25,26 The flow chart indicate the summary of the projected work in this manuscript (Figure 1).
Designing of dasatinib derivatives library
The first step involved the extraction of the canonical SMILE format of the principal compound dasatinib. In the subsequent building protocol phase, the primary molecule, represented by the authoritative SMILE ID, was submitted using the web-based tool MolOpt,27 which was both accessible and user-friendly. The identification of molecule replacement groups was facilitated by the bioisosteric substitution technique aimed at enhancing potency and efficacy.28 Rules from the data-mining (fast) replacement approach were employed to generate derivatives.28 The system subsequently provided output in the form of derivatives or subsidiaries along with their SMILE IDs. These SMILE IDs were subsequently converted into PDB format using the NovPro interface for further examination (https://www. novoprolabs.com/).
Library screening
To examine docking studies, the chemical structure and protein were subjected to PyRx 0.8 version, a virtual screening tool. The procedure entailed converting both the chemical compounds and proteins into ‘pdbqt’ format using the Open Babel tool provided by PyRx. A grid box was then constructed to capture the active binding site, with dimensions aligned with the binding pocket of the protein, ascertained by tracing the borders of the pertinent box.29,30 The grid box coordinates were as follows: Center X, 30.8658; Y, -2.6215; Z, 43.6183; dimension (Angstrom): X, 14.0975; Y, 15.9761; Z, 25.0604. Following docking, the results were saved in ‘CSV’ format, and energies were scrutinized with the aid of the PyMol visualization tool. Molecules with the most favorable energies were identified to filter the data.
Prediction of biological spectrum activities
The biological activity parameters for a set of compounds were predicted using the PASS prediction tool (https://www.way2drug.com/passonline/index.php).31 The aim of this process is to determine the presence or absence of specific bioactivities of the compounds, as indicated by their Pa and Pi values. To begin the analysis, compound structures were submitted in the SMILES format using the online tool NovPro. Subsequently, the mass spectrum activities and predicted values were evaluated. The assessment was conducted based on the concepts of Pa and Pi, which represent the probabilities of active and inactive compounds, respectively. To be considered worthy of further investigation, the Pa and Pi values of a compound should be between 0.000 and 1.000, with Pa exceeding Pi.
Evaluation of pharmacokinetic features and drug-likeness forecast
The ADMET profile was utilized to evaluate pharmacokinetic characteristics, such as surface area, number of hydrogen bond donors, hydrogen bond acceptors, and blood-brain barrier permeation.32 Determining this profile is considered the most critical and sensitive step in drug development.26,33 Compounds generated through profiling were identified as potential drug candidates based on their pharmacokinetic activities and drug-likeness. The pkCSM prediction tool was employed for this analysis.34,35
Estimating the toxicity and predicting the adverse side-effects
The online tools GUSAR (http://www.way2drug.com)/gusar/ acutoxpredict.html) and ADVER-Pred were used to predict severe acute rat toxicity and potential secondary complications. The General Unrestricted Structure-Activity Relationship (GUSAR)36 was employed to investigate toxicity in rodents through various routes of administration, such as intraperitoneal, intravenous, oral, and subcutaneous, to determine the dose for evaluating the analgesic activity of the compounds under examination. Conversely, ADVER-PRED (http://www.way2drug.com/adverpred/)37 was utilized to identify potential adverse effects indicated by the values of Pa and Pi.
Molecular dynamics simulation
Simulation studies were conducted on selected lead compounds to evaluate the effects of the changes and identify the most suitable drug lead compounds. Molecular Dynamics (MD) studies, which are widely considered the most effective method for understanding the atomic-level chemistry of biological macromolecules,38 was employed in this research. Using GROMACS software version 5.1.2 at 300 K, MD studies were carried out.39 The gmx grep module within the GROMACS package was used to extract and assemble the complexes and topologies. The force field CGenFF (CHARMm General Force Field) was applied to the compounds.40 The solvation step involved the use of the TIP3 model to solvate each complex within a cubic box. After the boundary conditions were applied, salts were introduced to equilibrate the charges. The framework underwent 25,000,000 steps via the steepest descent algorithm for energy minimization. Both proteins and ligands were prepared, and their interactions were examined using the mesh particle Ewald methodology following the equilibration phase.41,42 Furthermore, a gradual temperature equilibration process was performed. Following the equilibration phase, the system was prepared for simulation and directed for 5 ns to gain insights.43,44 Lastly, the MD trajectories for both systems were analyzed to estimate the Root Mean Square Deviation (RMSD), Root Mean Square Fluctuation (RMSF), and radius of gyration.
Principal component analysis
The fundamental principle of Principal Component Analysis (PCA) unambiguously illustrates the progressive development of proteins across various stages of reproduction.45 PCA reveals the underlying atomic fluctuations that govern the structure of a protein by reducing the complexity of Molecular Dynamics (MD) results.46 In galaxy research, PCA has been utilized to gain insights and investigate the conformational changes that result from intricate interactions between drugs and proteins.47 Throughout the 5 ns trajectory, the characteristics and configuration of the targeted protein and selected compounds were meticulously emphasized.48
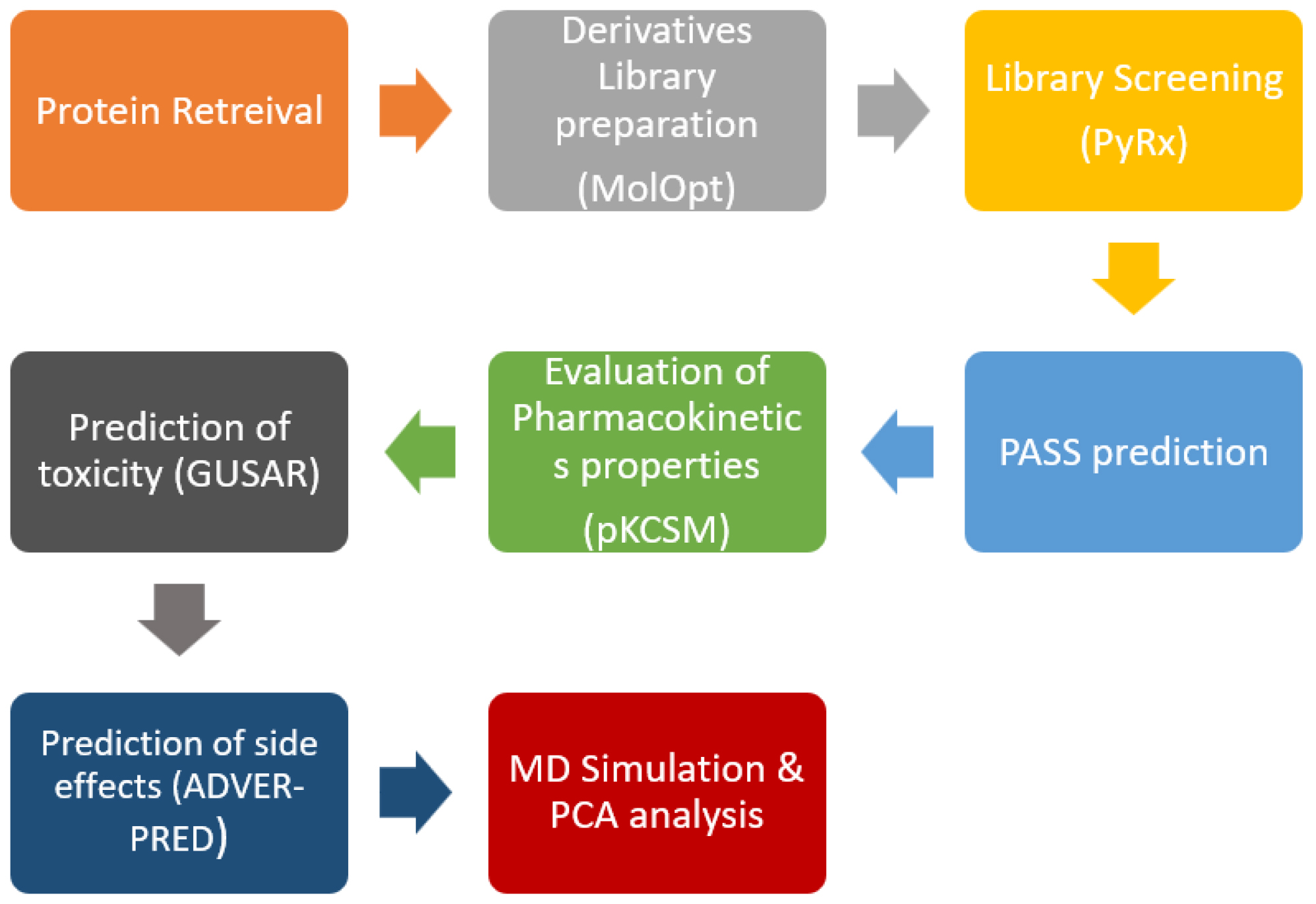
Figure 1:
Flow chart of Methodology exhibiting various steps.
RESULTS
Compounds preparation
fqImplementing the MolOpt guidelines, structural analogs of dasatinib were designed with the goal of improving the pharmacokinetic properties. A total of 29 replaceable groups were identified through various bioisosteric substitution sites. Among these, the hydroxyl group was specifically chosen as a replaceable group reasoned to obtain new interaction sites for better accuracy and efficacy (Figure 2), ultimately yielding 163 different variants.
Docking Filtration
Docking studies were performed on 163 derivatives, including dasatinib, that targeted the PTK6 receptor. The analysis demonstrated that all derivative compounds exhibited binding within the active site, with higher binding energies than the control standard drug. Specifically, the binding energies ranged from -12 kcal/mol to -8 kcal/mol, following a thorough investigation. Subsequently, derivatives exhibiting the highest binding energies and better interactions were selected, resulting in the identification of 11 compounds along with the standard drug dasatinib (Table 1). Visualization and evaluation were conducted using PyMol and Biovia Discovery Studio, respectively.
Compounds | Binding affinity (kcal/mol) |
---|---|
control.pdb | -8.0 |
mol_000.pdb | -11.1 |
mol_001.pdb | -11.0 |
mol_007.pdb | -10.5 |
mol_010.pdb | -10.4 |
mol_019.pdb | –10.8 |
mol_023.pdb | -10.2 |
mol_036.pdb | -10.3 |
mol_070.pdb | -11.5 |
mol_103.pdb | -10.6 |
mol_109.pdb | -10.9 |
mol_143.pdb | -12.0 |
Binding affinities comparison of dasatinib control drug and top analog compounds.
PASS prediction spectrum
To systematically evaluate the biological activities of substances, we employed the online tool way2 drug for PASS prediction.49 This analysis provided various chemical descriptors with a focus on specific parameters, such as aurora kinase inhibitor, fibroblast growth factor antagonist, Erbb antagonist, CDK6, and antineoplastic activity against solid tumors, all tailored to our target disease, breast cancer. Several studies have highlighted the overexpression of aurora kinase in various cancers, including breast cancer, thus emphasizing the importance of AK inhibition.50 Similarly, FGF and Erbb have been identified as potential therapeutic targets for breast cancer treatment.51,52 Additionally, cyclin-dependent kinases have emerged as crucial targets in cancer therapeutics, particularly in breast cancer treatment.53 Therefore, these parameters are of particular concern which can not only support the current study but will also help in estimating better therapeutic results. The primary goal of the PASS prediction was to comprehensively assess these parameters, ensuring that each compound exhibited a Pa value greater than Pi (Table 2).
Compounds | Aurora kinase inhibitor | Erbb antagonist | Erbb 2 antagonist | Antineoplastic | FGF antagonist | CDK-6 inhibitor | ||||||
---|---|---|---|---|---|---|---|---|---|---|---|---|
Pa | Pi | Pa | Pi | Pa | Pi | Pa | Pi | Pa | Pi | Pa | Pi | |
control.pdb | 0.198 | 0.014 | 0.098 | 0.022 | 0.093 | 0.022 | 0.251 | 0.137 | 0.105 | 0.048 | ||
mol_000.pdb | 0.271 | 0.008 | 0.145 | 0.012 | 0.141 | 0.012 | 0.272 | 0.115 | 0.079 | 0.057 | 0.102 | 0.051 |
mol_001.pdb | 0.197 | 0.014 | 0.348 | 0.004 | 0.286 | 0.005 | 0.311 | 0.081 | 0.135 | 0.022 | 0.130 | 0.025 |
mol_007.pdb | 0.173 | 0.018 | 0.090 | 0.026 | 0.075 | 0.033 | 0.222 | 0.178 | 0.112 | 0.039 | ||
mol_010.pdb | 0.208 | 0.012 | 0.151 | 0.012 | 0.107 | 0.017 | 0.375 | 0.114 | 0.117 | 0.029 | 0.156 | 0.016 |
mol_019.pdb | 0.168 | 0.019 | 0.221 | 0.007 | 0.158 | 0.010 | 0.258 | 0.130 | 0.115 | 0.030 | 0.142 | 0.020 |
mol_023.pdb | 0.187 | 0.015 | 0.106 | 0.020 | 0.101 | 0.019 | 0.230 | 0.166 | 0.077 | 0.061 | 0.077 | 0.061 |
mol_036.pdb | 0.165 | 0.020 | 0.199 | 0.008 | 0.142 | 0.011 | 0.253 | 0.135 | 0.111 | 0.031 | 0.141 | 0.020 |
mol_070.pdb | 0.127 | 0.032 | 0.089 | 0.079 | ||||||||
mol_103.pdb | 0.162 | 0.021 | 0.185 | 0.009 | 0.122 | 0.014 | 0.297 | 0.093 | 0.081 | 0.054 | 0.147 | 0.018 |
mol_109.pdb | 0.200 | 0.013 | 0.167 | 0.010 | 0.162 | 0.010 | 0.260 | 0.127 | 0.097 | 0.060 | ||
mol_143.pdb | 0.173 | 0.018 | 0.137 | 0.013 | 0.087 | 0.024 | 0.278 | 0.109 | 0.074 | 0.066 | 0.178 | 0.012 |
PASS prediction of dasatinib control drug and top analog compounds.
ADMET screening
In the field of drug development, the assessment of ADMET properties is of utmost importance as it provides information on key factors such as absorption, distribution, metabolism, excretion, & toxicity.54,55 All compounds were evaluated for their ADME profiles, and it was determined that they met the criteria and adhered to the Lipinski rule (Table 3). Notably, the bioavailability score was consistently 0.55 across all compounds, including the control. Further examination was carried out using the Swiss ADME.56 Furthermore, the pkCSM approach uncovered additional molecular characteristics beyond drug-likeness metrics, including skin permeability, CYC inhibitors, AMES, hERG inhibitors, and hepatotoxicity,57 as detailed in Table 4.
Compounds | Formula | MW* | RB* | HBA* | HBD* | BBB* | BS* | RO5 | TPSA |
---|---|---|---|---|---|---|---|---|---|
control.pdb | C22H26ClN7O2S | 488.01 | 8 | 6 | 3 | No | 0.55 | 0 | 134.75 |
mol_000.pdb | C22H24ClN7O2S | 485.99 | 7 | 5 | 2 | No | 0.55 | 0 | 131.59 |
mol_001.pdb | C22H24ClN7O2S | 485.99 | 7 | 5 | 2 | No | 0.55 | 0 | 131.59 |
mol_007.pdb | C23H28ClN7OS | 486.03 | 7 | 5 | 2 | No | 0.55 | 0 | 114.52 |
mol_010.pdb | C24H23ClN6OS | 479 | 9 | 4 | 3 | No | 0.55 | 0 | 120.07 |
mol_019.pdb | C22H26ClN7OS | 472.01 | 9 | 5 | 3 | No | 0.55 | 0 | 123.31 |
mol_023.pdb | C22H26ClN7OS | 472.01 | 9 | 5 | 3 | No | 0.55 | 0 | 123.31 |
mol_036.pdb | C23H28ClN7OS | 486.03 | 9 | 5 | 3 | No | 0.55 | 0 | 123.31 |
mol_070.pdb | C23H25ClN6O3S | 501 | 8 | 6 | 3 | No | 0.56 | 1 | 148.58 |
mol_103.pdb | C22H26ClN7OS | 472.01 | 7 | 5 | 4 | No | 0.55 | 0 | 146.09 |
mol_109.pdb | C22H26ClN7OS | 472.01 | 7 | 5 | 4 | No | 0.55 | 0 | 146.09 |
mol_146.pdb | C25H23ClN6OS | 491.01 | 7 | 4 | 3 | No | 0.55 | 0 | 120.07 |
ADME properties of control and selected analogs.
Attributes | Analogs | |||||||||||
---|---|---|---|---|---|---|---|---|---|---|---|---|
Control | 000 | 001 | 007 | 010 | 019 | 023 | 036 | 070 | 103 | 109 | 146 | |
Absorption | ||||||||||||
Skin perm. (log mol/l) | -3.087 | -3.153 | -3.046 | -3.146 | -2.948 | -3.085 | -3.116 | -3.063 | -2.835 | -3.12 | -3.124 | -2.997 |
Water sol. (log kp) | -4.573 | -5.411 | -4.706 | -5.309 | -5.699 | -5 | -5.251 | -5.054 | -5.1 | -5.004 | -5.196 | -5.455 |
Distribution | ||||||||||||
CNS perm. | -2.745 | -2.386 | -2.771 | -2.212 | -1.975 | -2.475 | -2.117 | -2.384 | -2.956 | -2.261 | -3.086 | -0.183 |
BBB perm. | -1.438 | -1.433 | -1.55 | -1.354 | -1.321 | -1.455 | -1.364 | -1.478 | -1.708 | -1.46 | -1.534 | -1.239 |
Metabolism | ||||||||||||
CYP2D6 subs. | No | No | No | No | No | No | No | No | No | No | No | No |
CYP3A4 subs. | Yes | Yes | Yes | Yes | Yes | Yes | Yes | Yes | Yes | Yes | Yes | Yes |
CYP1A2 inh. | No | No | No | No | No | No | No | No | No | No | No | No |
CYP2C19 inh. | No | No | No | No | Yes | No | No | No | No | No | No | Yes |
CYP2C9 inh. | No | No | No | No | Yes | No | No | No | No | No | No | Yes |
CYP2D6 inh. | No | No | No | No | No | No | No | No | No | No | No | No |
CYP3A4 inh. | Yes | Yes | Yes | Yes | Yes | Yes | Yes | Yes | Yes | Yes | Yes | Yes |
Excretion | ||||||||||||
Total clearance | 0.515 | 0.26 | 0.698 | 0.444 | 0.039 | 0.704 | 0.568 | 0.566 | -0.017 | 0.634 | 0.599 | -0.139 |
RenalOCT2 subs. | No | No | No | No | No | No | No | No | No | No | No | No |
Toxicity | ||||||||||||
AMES | No | No | No | No | No | No | No | No | No | No | No | No |
hERG I inhibitor | No | No | No | No | No | No | No | No | No | No | No | No |
hERG II inhibitor | Yes | Yes | Yes | Yes | Yes | Yes | Yes | No | No | Yes | Yes | Yes |
Hepatotoxicity | Yes | Yes | Yes | Yes | Yes | Yes | Yes | Yes | Yes | Yes | Yes | Yes |
Drug-likeness and toxicity profiling of control and selected analogs.
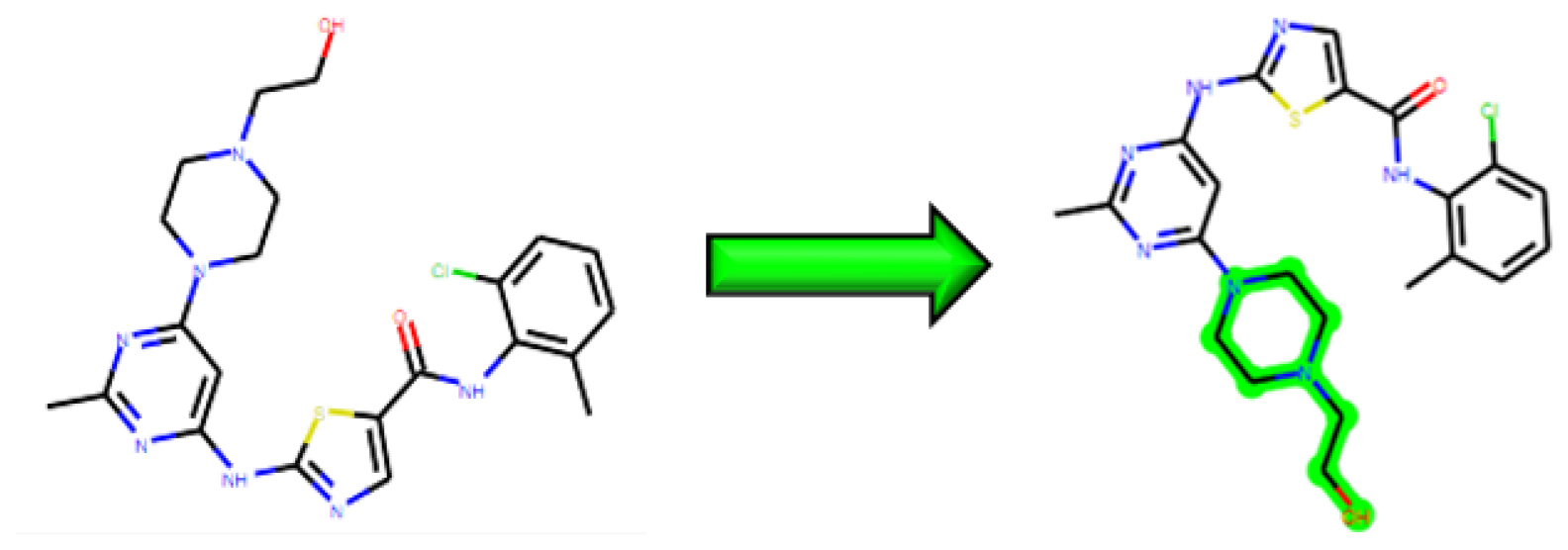
Figure 2:
2D representation of dasatinib, with the green highlighted portion indicating a replaceable group.
Toxicity prediction
The toxicity of various derivatives was assessed using the GUSAR acute rat toxicity database, which categorizes all derivatives as Class III for all administration routes, including Intraperitoneal (IP), Intravenous (IV), oral, & Subcutaneous (SC). In comparison, the standard drugs fell into the Class IV category. Dasatinib demonstrated toxicity predictions, including an IP LD50 of 423,800 mg/kg, an IV LD50 of 137,400 mg/kg, an oral LD50 of 735,100 mg/kg, and an SC LD50 of 976,300 mg/kg. However, no toxicity was observed for the derivatives.
Evaluation of side effects
Identifying potential adverse effects is an essential component of the lead candidate discovery process. By employing the Adver-pred web service, we were able to effectively uncover possible adverse effects, which are represented by the values of Pa and Pi (Table 5). The data showed that all derivatives, as well as the control drug, displayed similar adverse effects, such as cardiac failure, arrhythmia, and myocardial infarction.
Compounds | Pa | Pi | Side Effects |
---|---|---|---|
Control | 0.967 | 0.003 | Arrhythmia |
0.932 | 0.004 | Myocardial infarction | |
0.927 | 0.003 | Cardiac failure | |
mol_00.pdb | 0.939 | 0.004 | Myocardial infarction |
0.921 | 0.004 | Cardiac failure | |
0.891 | 0.005 | Arrhythmia | |
mol_003.pdb | 0.934 | 0.003 | Cardiac failure |
0.919 | 0.004 | Myocardial infarction | |
0.767 | 0.017 | Arrhythmia | |
mol_007.pdb | 0.96 | 0.003 | Arrhythmia |
0.927 | 0.004 | Myocardial infarction | |
0.898 | 0.004 | Cardiac failure | |
mol_010.pdb | 0.953 | 0.004 | Myocardial infarction |
0.952 | 0.003 | Cardiac failure | |
0.887 | 0.005 | Arrhythmia | |
mol_019.pdb | 0.932 | 0.003 | Cardiac failure |
0.931 | 0.004 | Myocardial infarction | |
0.924 | 0.004 | Arrhythmia | |
mol_023.pdb | 0.972 | 0.002 | Arrhythmia |
0.936 | 0.004 | Myocardial infarction | |
0.911 | 0.004 | Cardiac failure | |
mol_036.pdb | 0.928 | 0.004 | Myocardial infarction |
0.927 | 0.004 | Arrhythmia | |
0.926 | 0.003 | Cardiac failure | |
mol_070.pdb | 0.935 | 0.004 | Myocardial infarction |
0.916 | 0.004 | Cardiac failure | |
0.844 | 0.009 | Arrhythmia | |
mol_103.pdb | 0.95 | 0.004 | Myocardial infarction |
0.899 | 0.004 | Cardiac failure | |
0.859 | 0.008 | Arrhythmia | |
mol_109.pdb | 0.903 | 0.005 | Myocardial infarction |
0.891 | 0.004 | Cardiac failure | |
0.657 | 0.032 | Arrhythmia | |
mol_l46.pdb | 0.966 | 0.003 | Myocardial infarction |
0.903 | 0.005 | Arrhythmia | |
0.902 | 0.004 | Cardiac failure |
Evaluation of possible adverse effects of control and selected analogs.
Following extensive evaluation of all parameters, compounds mol_00 and mol_010 were identified as the most promising candidates because of their nontoxic nature, negative AMES results, and lack of inhibition of non-hERG channels, as well as various PASS prediction parameters indicative of minimal side effects. Further investigations, including molecular dynamics studies and principal component analysis, are necessary to determine the most suitable derivative inhibitor for the treatment of PTK6-associated breast cancer. The 3D illustrations in Figure 3A-C provide visual representations of the interactions and residues involved.
Compound A displayed a total of 10 van der Waals interactions, five conventional hydrogen bonds, one carbon-hydrogen bond, and two Pi-sigma, Pi-alkyl, and alkyl interactions. In contrast, Compound B exhibited four conventional hydrogen bonds: carbon-hydrogen bond, Pi-sigma, Pi-alkyl, and alkyl interactions. The control compound was characterized by one halogen and covalent bond as well as other interactions.
Molecular dynamic simulation
A comprehensive examination was implemented to assess the stability of the requisite compounds within the ligands and complexes.58 An assortment of trajectory plots, such as RMSD, RMSF, SASA, & Rg, were scrutinized to assess the interaction stability of ligands & protein comes.59 The simulation study was carried out over a 5 ns period.
Analysis of stability and compactness
Root-Mean-Square Deviation (RMSD) has long been a perilous component in stability assessment, providing a vital statistic for evaluating protein stability relative to the experimental structure.60 A lower RMSD value indicated greater stability. In this context, the mean RMSD values of 0.9 nm & 1.2 nm were calculated for the PTK6 protein backbone and ligand, respectively. The RMSD graph shows the superior stability of compound 01, with a value of 0.9 nm, surpassing that of the control at 1.03 nm.61 The Root-MeanSquare Fluctuation (RMSF) captures residue fluctuations upon binding.62 In the RMS-fluctuation analysis, structural deviations were observed for both compounds compared with the control.
Compound 01 exhibited a slightly higher degree of fluctuation. RMSF analysis offers insights into how protein residues fluctuate upon ligand binding throughout the progression.63 Detailed plots of RMSF and RMSD are presented in Figures 4A-B.
Determination of compactness: Radius of gyration
Radius of gyration (Rg) is an essential metric for various aspects of a chain molecule. It provides insight into the extent of structural shifts a protein undergoes during molecular dynamics simulations. Rg typically evaluates compactness and flexibility within a biological context by tracking changes in protein structure over time. This study revealed the relative compactness of the macromolecule, as depicted in Figure 4C.64 Both compounds showed average Rg values, with readings of 3.1 nm and 3.2 nm, respectively. The control compound had an Rg value of 3.13 nm.
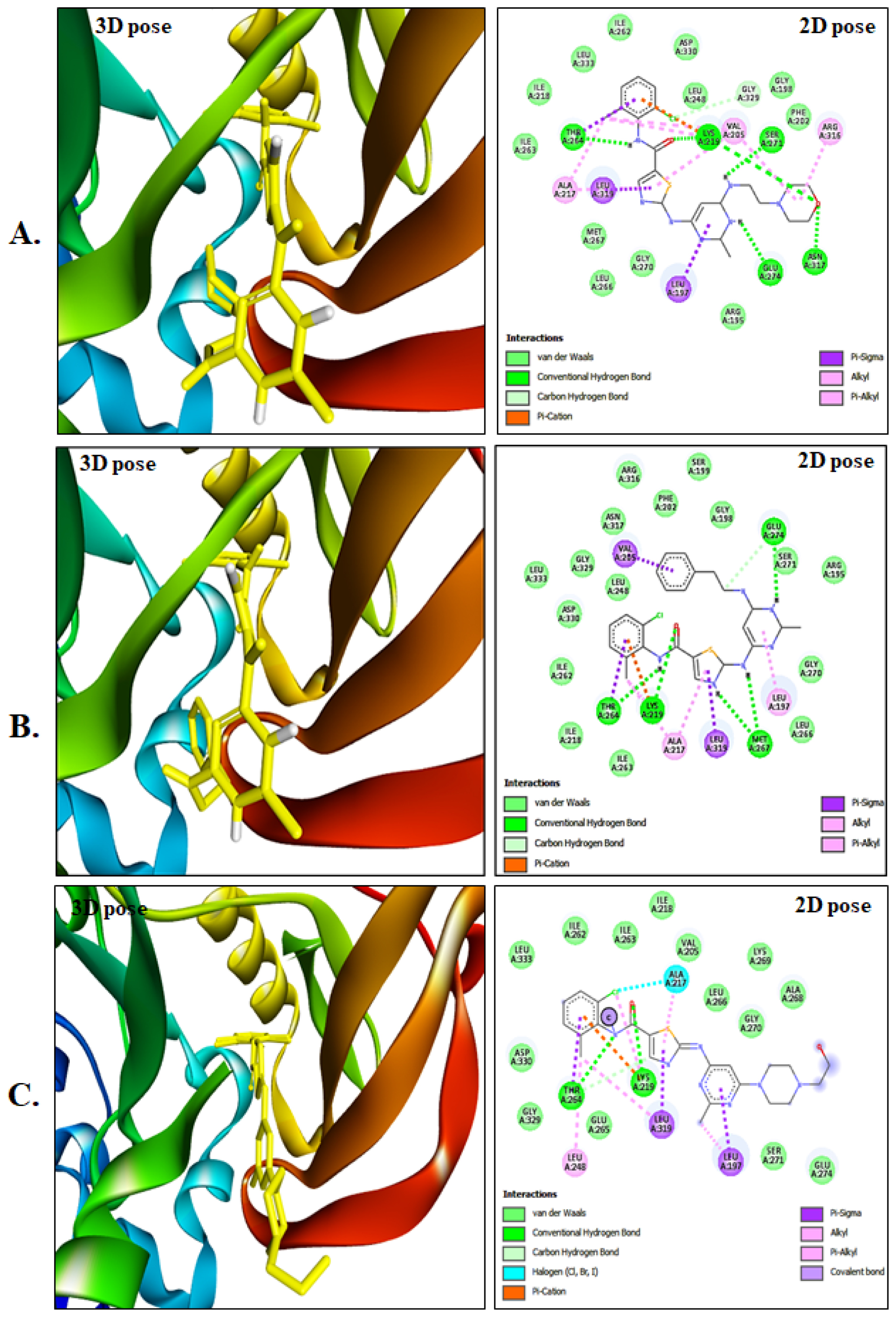
Figure 3:
3D representation of bound derivatives, accompanied by 2D diagrams highlighting interacting amino acid residues. (A) Depicts Compound 01, while (B) Showcases Compound 10, and (C) Portrays the Control compound.
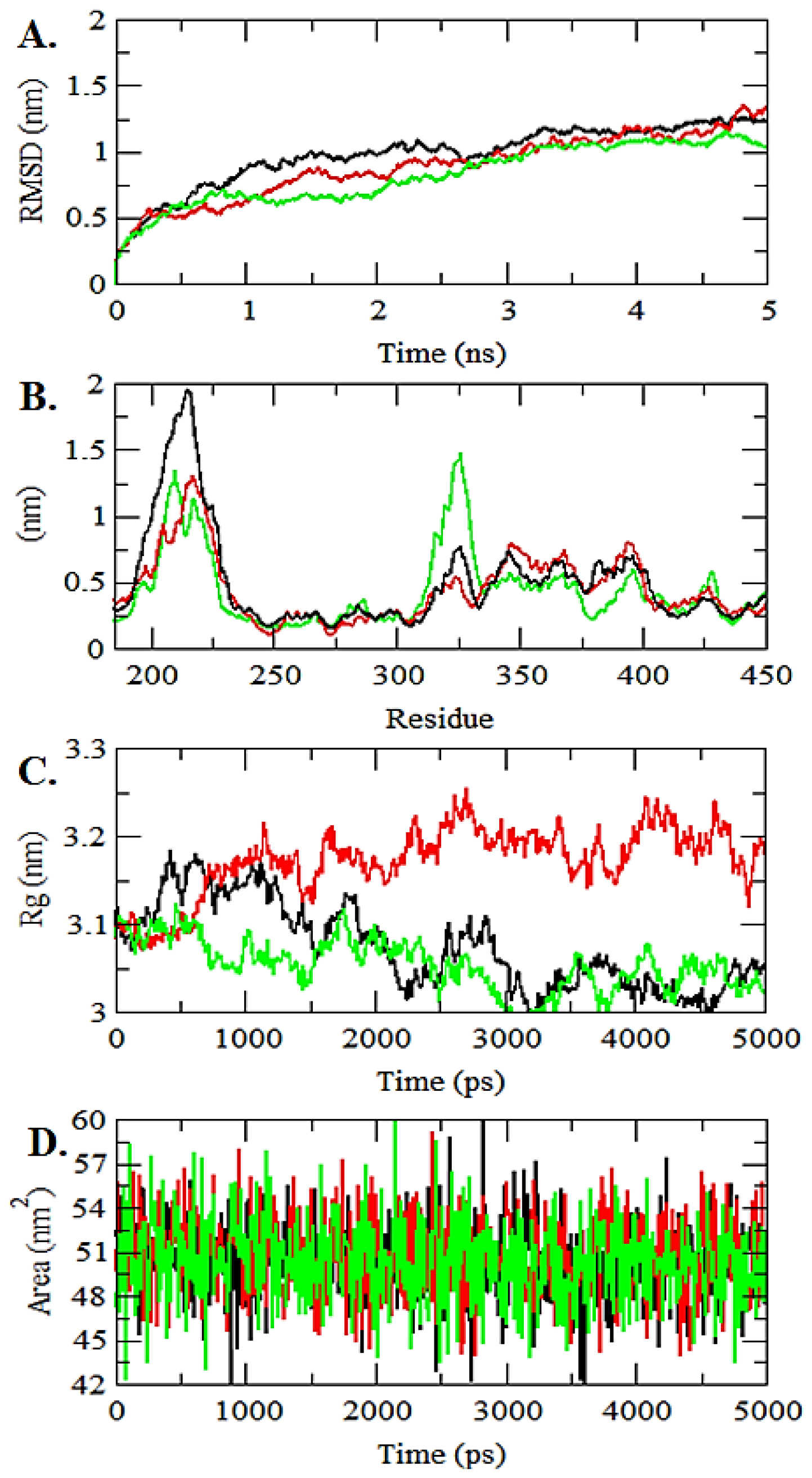
Figure 4:
MD trajectories of compounds 01 and 10 alongside the control drug, depicting: (A) RMSD plot, (B) RMSF plot, (C) Radius of gyration graph plot, and (D) SASA plot representation. In the legend, red represents compound 10, green represents compound 01, and black indicates the standard control drug.
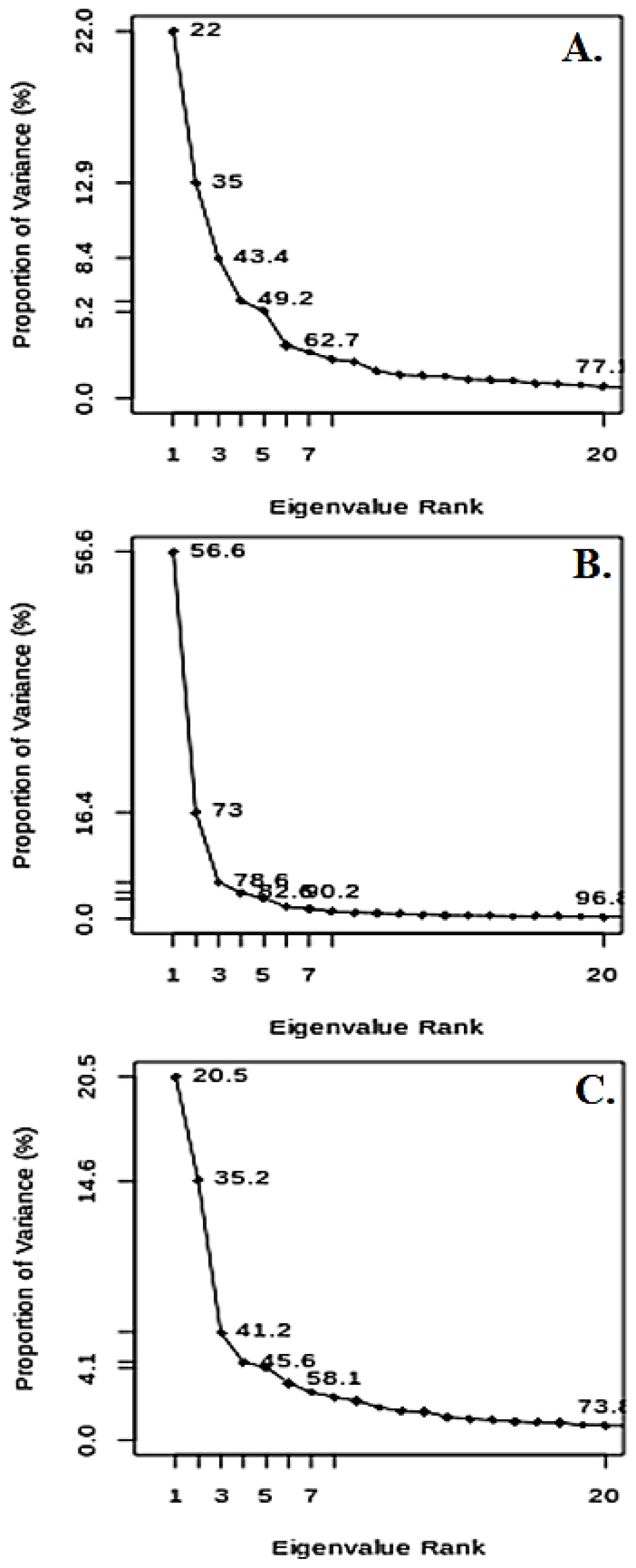
Figure 5:
The graph shows how much the variance of (A) Compound 01, (B) Compound 10, and (C) Control differ from each other.
SASA determination
The Solvent-Accessible Surface Area (SASA) is a measure of the surface area of a biomolecule and represents the extent to which the atoms of the protein on the surface can interact with the solvent. SASA has been utilized to better understand the continuity and conformational changes following ligand-binding interactions.65 A higher SASA value indicates expansion of the protein structure, facilitating solvent access to a larger surface area. As illustrated in Figure 4D, compound 01 displayed a higher SASA value, indicating a larger exposed area.
The trajectories of Molecular Dynamics simulation detailed analysis revealed compound 1 as the best compound.
Principal Component Analysis (PCA)
PCA is a multivariate investigation procedure that simplifies complex data while retaining variance. In this study, PCA was applied to the top two selected compounds and the standard drug to reveal variations, while minimizing complexity. The gmx analog module was used to extract eigenvalues.
The fractions of diversity measured by PCI for compounds 1 and 2 were 22% and 56.6%, respectively, indicating significant variations. For the control group, a variance of 20.5% was observed. Lower eigenvalues indicate greater stability which is also found as a supporting pillar for compound 01. The detailed eigenvalues are illustrated in Figure 5A-C. PCA variance arises from its capability to generate principal components, which function as effective variables for estimating variance.
DISCUSSION
Breast cancer is the prominent cause of fatalities among women, primarily because of its poor prognosis and persistent nature. Treatment of breast cancer that is resistant to conventional treatments presents significant therapeutic challenges. Cancer cells possess inherent or acquired mechanisms that reduce the lethal effects of cytotoxic drugs, which lead to the recurrence of breast cancer and account for the majority of morbidity rates.
Dasatinib has been shown to inhibit PTK6 activity with an IC50 in the nm range and is a promiscuous kinase inhibitor that inhibits both Src and ABL tyrosine kinases. Therefore, it is crucial to develop effective inhibitors that can specifically target PTK6 and induce apoptosis and growth arrest in breast cancer cells without harming healthy cells.12,15 In the present study, we demonstrated that inhibiting PTK6 leads to apoptosis in dasatinib-resistant breast cancer cells.22
Recently, there has been renewed interest in the discovery of small molecules for the treatment of breast cancer. Bioinformatic methods have been widely employed in the development and discovery of new compounds.30 In this study, computational approaches were used to conduct the research. The MolOpt database was utilized to construct the derivatives, resulting in the generation of 163 compounds. From these, 11 compounds were selected on the basis of their binding energies. The docking energy for the parent compound was -8 kcal/mol, while the other compounds exhibited energies greater than -8 kcal/mol. These compounds were tested under various conditions to identify hits. Additionally, other computational methods were employed, including pharmacokinetic profiling, acute rat toxicity prediction, pass prediction, and assessment of potential side effects following virtual screening. The drug likelihood and ADME determination profiles are depicted in Table 3, and Table 4 provides detailed information on compounds with various characteristics, such as AMES and hERG inhibitor activity.
The outcomes of all compounds were compared with those of the control substance, dasatinib. The analysis suggested that the compounds mol_01 and mol_10 performed better than the others and met all ADME criteria while adhering to the Lipinski rule without any violations. Following the assessment of these parameters, molecular dynamics simulations and Principal Component Analysis (PCA) were conducted on the top-filtered compounds. According to our analysis, the MD study revealed that compound_01 outperformed all other MD trajectories, such as RMSD, RMSF, Rg, and SASA, compared to the other compounds as well as the parent compound. Detailed results of the MD analysis are shown in Figure 4. Furthermore, a lower variance of 22% was observed for mol_01 through PCA, indicating its favorable properties such as fibroblast growth factor, CDK-6, and antineoplastic activities to be a lead compound. However, it is important to note that despite our comprehensive computational analysis, there may be limitations to our research, including the absence of experimental validation or survey analysis to support our predictions.
CONCLUSION
In summary, our results indicate that PTK6 inhibition has the possible to be a valuable strategy for inducing apoptosis through the development of dasatinib derivatives. By utilizing modern computational methodologies, we designed and analyzed novel PTK6 inhibitors, which have the potential to be promising therapeutic candidates for the management of breast cancer. Our research identified mol_01 as a compound with significant inhibitory effects on various biological characteristics, including aurora kinase, fibroblast growth factor, CDK-6, and antineoplastic activities, with superior binding affinities compared to other compounds. This compound possesses all the promising therapeutic that could help in treating the ailment of breast cancer. Importantly, mol_01 demonstrated no toxicity and had only three side effects, similar to those associated with the commercial drug dasatinib. Furthermore, molecular dynamics studies support our findings, showing a decrease in the primary component and reinforcing the efficacy of mol_01 as a potential therapeutic agent. However, further optimization is necessary during the drug discovery phase. In light of these findings, it is crucial to conduct experimental validation to support our data and pave the way for proper clinical trials of the identified drug. Ensuring the safety and efficacy of mol_01 through rigorous experimentation is essential before its practical application in clinical settings.
Cite this article:
Asiri A, Ali AA, Abu-Alghayth MH. Targeting Breast Cancer with Dasatinib Derivatives: A Multi-Parameter Strategy to Uncover Potent Lead Compounds. Int. J. Pharm. Investigation. 2024;14(4):1260-72.
ACKNOWLEDGEMENT
The authors extend their appreciation to the Deanship of Graduate Studies and Scientific Research at University of Bisha for funding this research through the general research project under grant number (UB-GRP- 39 -1444).
Grant number (UB-GRP- 39 -1444).
FUNDING
The authors extend their appreciation to the Deanship of Graduate Studies and Scientific Research at University of Bisha for funding this research through the general research project under grant number (UB-GRP- 39 -1444).
Grant number (UB-GRP- 39 -1444).
ABBREVIATIONS
PTKs | Protein Tyrosine Kinases |
---|---|
NRTKs | Non-receptor Tyrosine Kinases |
BRK | Breast Tumor Kinase |
PDB | Protein Data Bank |
GUSAR | General Unrestricted Structure-Activity Relationship |
MD | Molecular dynamics |
RMSD | Root Mean Square Deviation |
RMSF | Root Mean Square Fluctuation |
PCA | Principal Component Analysis |
IP | Intraperitoneal |
IV | Intravenous |
SC | Subcutaneous |
Rg | Radius of Gyration |
SASA | Solvent-Accessible Surface Area |
References
- Ahmad K, Khan MK, Baig MH, Imran M, Gupta GK. Role of azoles in cancer prevention and treatment: present and future perspectives. Anti-Cancer Agents Med Chem. 2018;18(1):46-56. [PubMed] | [CrossRef] | [Google Scholar]
- Chaurasia V, Pal S, Tiwari B. Prediction of benign and malignant breast cancer using data mining techniques. J Algorithm Comput Technol. 2018;12(2):119-26. [CrossRef] | [Google Scholar]
- Solanki YS, Chakrabarti P, Jasinski M, Leonowicz Z, Bolshev V, Vinogradov A, et al. A hybrid supervised machine learning classifier system for breast cancer prognosis using feature selection and data imbalance handling approaches. Electronics. 2021;10(6):699 [CrossRef] | [Google Scholar]
- Khan MS, Khan MKA, Ansari I, Arif J. Dietary phytochemicals as potent chemotherapeutic agents against breast cancer: inhibition of NF-κB pathway via molecular interactions in rel homology domain of its precursor protein p105. Pharmacogn Mag. 2014;9(33):51-7. [PubMed] | [CrossRef] | [Google Scholar]
- Testa U, Castelli G, Pelosi E. Breast cancer: A molecularly heterogenous disease needing subtype-specific treatments. Med Sci (Basel). 2020;8(1):18 [PubMed] | [CrossRef] | [Google Scholar]
- Sun YS, Zhao Z, Yang ZN, Xu F, Lu HJ, Zhu ZY, et al. Risk factors and preventions of breast cancer. Int J Biol Sci. 2017;13(11):1387-97. [PubMed] | [CrossRef] | [Google Scholar]
- Moo TA, Sanford R, Dang C, Morrow M. Overview of breast cancer therapy. PET Clin. 2018;13(3):339-54. [PubMed] | [CrossRef] | [Google Scholar]
- Baig MH, Ahmad K, Hasan Q, Khan MK, Rao NS, Kamal MA, et al. Interaction of glucagon G-protein coupled receptor with known natural antidiabetic compounds: multiscoring approach. Evid Based Complement Alternat Med. 2015;2015:497253 [PubMed] | [CrossRef] | [Google Scholar]
- Loprinzi PD, Cardinal BJ. Effects of physical activity on common side effects of breast cancer treatment. Breast Cancer. 2012;19(1):4-10. [PubMed] | [CrossRef] | [Google Scholar]
- Hale MJ, Howell A, Dowsett M, Cuzick J, Sestak I. Tamoxifen related side effects and their impact on breast cancer incidence: A retrospective analysis of the randomised IBIS-I trial. Breast. 2020;54:216-21. [PubMed] | [CrossRef] | [Google Scholar]
- Arslan MA, Kutuk O, Basaga H. Protein kinases as drug targets in cancer. Curr Cancer Drug Targets. 2006;6(7):623-34. [PubMed] | [CrossRef] | [Google Scholar]
- Paul MK, Mukhopadhyay AK. Tyrosine kinase – role and significance in Cancer. Int J Med Sci. 2004;1(2):101-15. [PubMed] | [CrossRef] | [Google Scholar]
- Gocek E, Moulas AN, Studzinski GP. Non-receptor protein tyrosine kinases signaling pathways in normal and cancer cells. Crit Rev Clin Lab Sci. 2014;51(3):125-37. [PubMed] | [CrossRef] | [Google Scholar]
- Zhou Y, Wong MW. Array. Pharmaceuticals (Basel). 2023;17(1):60 [PubMed] | [CrossRef] | [Google Scholar]
- Jerin S, Harvey AJ, Lewis A. Therapeutic potential of protein tyrosine kinase 6 in colorectal cancer. Cancers. 2023;15(14):3703 [PubMed] | [CrossRef] | [Google Scholar]
- Derry JJ, Prins GS, Ray V, Tyner AL. Altered localization and activity of the intracellular tyrosine kinase BRK/Sik in prostate tumor cells. Oncogene. 2003;22(27):4212-20. [PubMed] | [CrossRef] | [Google Scholar]
- Aubele M, Walch AK, Ludyga N, Braselmann H, Atkinson MJ, Luber B, et al. Prognostic value of protein tyrosine kinase 6 (PTK6) for long-term survival of breast cancer patients. Br J Cancer. 2008;99(7):1089-95. [PubMed] | [CrossRef] | [Google Scholar]
- Patel P, Tsiperson V, Gottesman SR, Somma J, Blain SW. Dual inhibition of CDK4 and CDK2 via targeting p27 tyrosine phosphorylation induces a potent and durable response in breast cancer cells. Mol Cancer Res. 2018;16(3):361-77. [PubMed] | [CrossRef] | [Google Scholar]
- Mitchell PJ, Sara EA, Crompton MR. A novel adaptor-like protein which is a substrate for the non-receptor tyrosine kinase, BRK. Oncogene. 2000;19(37):4273-82. [PubMed] | [CrossRef] | [Google Scholar]
- Rix U, Hantschel O, Dürnberger G, Remsing Rix LL, Planyavsky M, Fernbach NV, et al. Chemical proteomic profiles of the BCR-ABL inhibitors imatinib, nilotinib, and dasatinib reveal novel kinase and nonkinase targets. Blood. 2007;110(12):4055-63. [PubMed] | [CrossRef] | [Google Scholar]
- Wozniak DJ, Hitchinson B, Gilic MB, Bie W, Gaponenko V, Tyner AL, et al. Vemurafenib Inhibits Active PTK6 in PTEN-null Prostate Tumor Cells. Mol Cancer Ther. 2019;18(5):937-46. [PubMed] | [CrossRef] | [Google Scholar]
- Thakur MK, Birudukota S, Swaminathan S, Battula SK, Vadivelu S, Tyagi R, et al. Co-crystal structures of PTK6: with dasatinib at 2.24 Å, with novel imidazo[1,2-a]pyrazin-8-amine derivative inhibitor at 1.70 Å resolution. Biochem Biophys Res Commun. 2017;482(4):1289-95. [PubMed] | [CrossRef] | [Google Scholar]
- Khan MK, Akhtar S. Novel drug design and bioinformatics: an introduction. Phys Sci Rev. 2023;8(8):1571-91. [CrossRef] | [Google Scholar]
- Baig MH, Ahmad K, Hasan Q, Khan MK, Rao NS, Kamal MA, et al. Interaction of glucagon G-protein coupled receptor with known natural antidiabetic compounds: multiscoring approach. Evid Based Complement Alternat Med. 2015;2015:497253 [PubMed] | [CrossRef] | [Google Scholar]
- Sarkar M, NA , KA , MC , AF , AM, et al. Synthesis, molecular docking screening, ADMET and dynamics studies of synthesized 4-(4-methoxyphenyl)-8-methyl-3,4. J Mol Struct. 2021; 1244; 5(6): 7, 8-Hexahydroquinazolin-2 (1H)-one and quinazolinone derivatives. [PubMed] | [CrossRef] | [Google Scholar]
- Singh R, Bhardwaj VK, Sharma J, Das P, Purohit R. Discovery and evaluation of aminoarylbenzosuberene molecules as novel checkpoint kinase 1 inhibitor determinants. Genomics. 2021;113(1 Pt 2):707-15. [PubMed] | [CrossRef] | [Google Scholar]
- Modee R, Mehta S, Laghuvarapu S, Priyakumar UD. MolOpt: autonomous molecular geometry optimization using multiagent reinforcement learning. J Phys Chem B. 2023;127(48):10295-303. [PubMed] | [CrossRef] | [Google Scholar]
- Dick A, Cocklin S. Bioisosteric replacement as a tool in anti-HIV drug design. Pharmaceuticals (Basel). 2020;13(3):36 [PubMed] | [CrossRef] | [Google Scholar]
- Langdon SR, Ertl P, Brown N. Bioisosteric replacement and scaffold hopping in lead generation and optimization. Mol Inform. 2010;17;29(5):366-85. [PubMed] | [CrossRef] | [Google Scholar]
- Singh K, Sharma A, Upadhyay TK, Hayat-ul-Islam M, Khan MK, Dwivedi UN, et al. Structure-based and Analysis Reveals Asiatic Acid as Novel Potential Inhibitor of Mycobacterium tuberculosis Maltosyl transferase. Curr Comput Aided Drug Des. 2022;18(3):213-27. [PubMed] | [CrossRef] | [Google Scholar]
- Dmitriev AV, Filimonov DA, Rudik AV, Pogodin PV, Karasev DA, Lagunin AA, et al. Drug-drug interaction prediction using pass. SAR QSAR Environ Res. 2019;30(9):655-64. [PubMed] | [CrossRef] | [Google Scholar]
- Lipinski CA. Lead- and drug-like compounds: the rule-of-five revolution. Drug Discov Today Technol. 2004;1(4):337-41. [PubMed] | [CrossRef] | [Google Scholar]
- Azzam K, Swiss AL. ADME and pkCSM Webservers Predictors: an integrated Online Platform for Accurate and Comprehensive Predictions for ADME/T Properties of artemisinin and its Derivatives. Kompleksnoe Ispol’zovanie Mineral Syr’â/Complex Use of Mineral Resources/Mineraldik Shikisattardy Keshendi Paidalanu. 2003;325:14-21. [CrossRef] | [Google Scholar]
- Domínguez-Villa FX, Durán-Iturbide NA, Ávila-Zárraga JG. Synthesis, molecular docking, and ADME/Tox profiling studies of new 1-aryl-5-(3-azidopropyl) indol-4-ones: potential inhibitors of SARS CoV-2 main protease. Bioorg Chem. 2021;106:104497 [PubMed] | [CrossRef] | [Google Scholar]
- Pires DE, Blundell TL, Ascher DB. pkCSM: Predicting small-molecule pharmacokinetic and toxicity properties using graph-based signatures. J Med Chem. 2015;58(9):4066-72. [PubMed] | [CrossRef] | [Google Scholar]
- Lagunin A, Zakharov A, Filimonov D, Poroikov V. QSAR modelling of rat acute toxicity on the basis of PASS prediction. Mol Inform. 2011;30(2-3):241-50. [PubMed] | [CrossRef] | [Google Scholar]
- Ivanov SM, Lagunin AA, Rudik AV, Filimonov DA, Poroikov VV. ADVERPred-Web service for prediction of adverse effects of drugs. J Chem Inf Model. 2018;58(1):8-11. [PubMed] | [CrossRef] | [Google Scholar]
- Khan S, Khan FI, Mohammad T, Khan P, Hasan GM, Lobb KA, et al. Exploring molecular insights into the interaction mechanism of cholesterol derivatives with the Mce4A: A combined spectroscopic and molecular dynamic simulation studies. Int J Biol Macromol. 2018;111:548-60. [PubMed] | [CrossRef] | [Google Scholar]
- Stenberg S, Stenqvist B. An exact Ewald summation method in theory and practice. J Phys Chem A. 2020;124(19):3943-6. [PubMed] | [CrossRef] | [Google Scholar]
- Fischer NM, van Maaren PJ, Ditz JC, Yildirim A, van der Spoel D. Properties of organic liquids when simulated with long-range Lennard-Jones interactions. J Chem Theor Comput. 2015;11(7):2938-44. [PubMed] | [CrossRef] | [Google Scholar]
- Grant BJ, Rodrigues AP, ElSawy KM, McCammon JA, Caves LS. Bio3d: an R package for the comparative analysis of protein structures. Bioinformatics. 2006;22(21):2695-6. [PubMed] | [CrossRef] | [Google Scholar]
- Petersen HG. Accuracy and efficiency of the particle mesh Ewald method. J Chem Phys. 1995;103(9):3668-79. [CrossRef] | [Google Scholar]
- Yu H, Dalby PA. A beginner’s guide to molecular dynamics simulations and the identification of cross-correlation networks for enzyme engineering. 2020:15-49. [PubMed] | [CrossRef] | [Google Scholar]
- Zhang Y, Zhang TJ, Tu S, Zhang ZH, Meng FH. Identification of novel Src inhibitors: pharmacophore-based virtual screening, molecular docking and Molecular Dynamics simulations. Molecules. 2020;25(18):4094 [PubMed] | [CrossRef] | [Google Scholar]
- Maisuradze GG, Liwo A, Scheraga HA. Principal component analysis for protein folding dynamics. J Mol Biol. 2009;385(1):312-29. [PubMed] | [CrossRef] | [Google Scholar]
- David CC, Jacobs DJ. Principal component analysis: A method for determining the essential dynamics of proteins. 2014:193-226. [PubMed] | [CrossRef] | [Google Scholar]
- Khan MK, Alouffi S, Ahmad S. Identifying potential inhibitors of C-X-C motif chemokine ligand10 against vitiligo: structure-based virtual screening, molecular dynamics simulation, and principal component analysis. J Biomol Struct Dyn. 2023:1-18. [PubMed] | [CrossRef] | [Google Scholar]
- Khan MK, Ahmad S, Rabbani G, Shahab U, Khan MS. Target-based virtual screening, computational multiscoring docking and molecular dynamics simulation of small molecules as promising drug candidate affecting kinesin-like protein KIFC1. Cell Biochem Funct. 2022;40(5):451-72. [PubMed] | [CrossRef] | [Google Scholar]
- Filimonov DA, Lagunin AA, Gloriozova TA, Rudik AV, Druzhilovskii DS, Pogodin PV, et al. Prediction of the biological activity spectra of organic compounds using the pass online web resource. Chem Heterocycl Compd. 2014;50(3):444-57. [CrossRef] | [Google Scholar]
- Tang A, Gao K, Chu L, Zhang R, Yang J, Zheng J, et al. Aurora kinases: novel therapy targets in cancers. Oncotarget. 2017;8(14):23937-54. [PubMed] | [CrossRef] | [Google Scholar]
- Schroeder RL, Stevens CL, Sridhar J. Small molecule tyrosine kinase inhibitors of ErbB2/HER2/Neu in the treatment of aggressive breast cancer. Molecules. 2014;19(9):15196-212. [PubMed] | [CrossRef] | [Google Scholar]
- Jafarzadeh M, Mousavizadeh K, Joghataei MT, Bahremani MH, Safa M, Asghari SM, et al. A fibroblast growth factor antagonist peptide inhibits breast cancer in BALB/c mice. Open Life Sci. 2018;13:348-54. [PubMed] | [CrossRef] | [Google Scholar]
- Grinshpun A, Tolaney SM, Burstein HJ, Jeselsohn R, Mayer EL. The dilemma of selecting a first line CDK4/6 inhibitor for hormone receptor-positive/HER2-negative metastatic breast cancer. npj Breast Cancer. 2023;9(1):15 [PubMed] | [CrossRef] | [Google Scholar]
- Islam S, Hosen MA, Ahmad S, ul Qamar MT, Dey S, Hasan I, et al. Synthesis, antimicrobial, anticancer activities, PASS prediction, molecular docking, molecular dynamics and pharmacokinetic studies of designed methyl α-D-glucopyranoside esters. J Mol Struct. 2022;1260:132761 [CrossRef] | [Google Scholar]
- Vardhan S, Sahoo SK. Array. Comput Biol Med. 2020;124:103936 [PubMed] | [CrossRef] | [Google Scholar]
- Hanee U, Rahman MR, Matin MM. Synthesis, PASS, ADMET and thermodynamic studies of some galactopyranoside esters. Phys Chem Res. 2021:9 [CrossRef] | [Google Scholar]
- Chen J, Swamidass SJ, Dou Y, Bruand J, Baldi P. ChemDB: a public database of small molecules and related chemoinformatics resources. Bioinformatics. 2005;21(22):4133-9. [PubMed] | [CrossRef] | [Google Scholar]
- Khan MK, Ahmad S, Rabbani G, Shahab U, Khan MS. Target-based virtual screening, computational multiscoring docking and molecular dynamics simulation of small molecules as promising drug candidate affecting kinesin-like protein KIFC1. Cell Biochem Funct. 2022;40(5):451-72. [PubMed] | [CrossRef] | [Google Scholar]
- Khan MK, Ahmad S, Rabbani G, Shahab U, Khan MS. Target-based virtual screening, computational multiscoring docking and molecular dynamics simulation of small molecules as promising drug candidate affecting kinesin-like protein KIFC1. Cell Biochem Funct. 2022;40(5):451-72. [PubMed] | [CrossRef] | [Google Scholar]
- Khan FI, Lai D, Anwer R, Azim I, Khan MK. Identifying novel sphingosine kinase 1 inhibitors as therapeutics against breast cancer. J Enzyme Inhib Med Chem. 2020;35(1):172-86. [PubMed] | [CrossRef] | [Google Scholar]
- Kausar MA, Shahid S, Anwar S, Kuddus M, Khan MK, Alotaibi AD, et al. Identifying natural therapeutics against diabetes via inhibition of dipeptidyl peptidase 4: molecular docking and MD simulation study. Indian J Pharm Educ Res. 2022;56(1):21-31. [CrossRef] | [Google Scholar]
- Kausar MA, Shahid S, Anwar S, Kuddus M, Khan MK, Khalifa AM, et al. Identifying the alpha-glucosidase inhibitory potential of dietary phytochemicals against diabetes mellitus type 2 via molecular interactions and dynamics simulation. Cell Mol Biol (Noisy-le-grand). 2022;67(5):16-26. [PubMed] | [CrossRef] | [Google Scholar]
- Ghahremanian S, Rashidi MM, Raeisi K, Toghraie D. Molecular dynamics simulation approach for discovering potential inhibitors against SARS-CoV-2: A structural review. J Mol Liq. 2022;354:118901 [PubMed] | [CrossRef] | [Google Scholar]
- Ahmad Khan MK, Almarshad F. Computational approaches for lead discovery against SARS-CoV-2 3C-like protease: virtual screening and Molecular Dynamics studies. J Med Health Stud. 2023:49-62. [CrossRef] | [Google Scholar]
- Rathod SB. Identification of PICK1 PDZ-Domain antagonists: pharmacophore-based virtual screening, molecular docking, and molecular dynamic simulation analyses. [CrossRef] | [Google Scholar]