ABSTRACT
Background
Lung cancer is the most reported cancer worldwide and therapeutic resistance poses a major challenge. This in silico molecular docking and molecular dynamic simulation study was carried out to evaluate the binding affinity and stability of molecular interactions between the phytochemical Matairesinol and the molecular targets in Non-Small Cell Lung Carcinoma (NSCLC) which are implicated in the pathogenesis and development of therapeutic resistance, thereby exploring its potential anticancer activity in NSCLC.
Materials and Methods
The three-dimensional structure of Matairesinol was obtained from the PubChem database. The three-dimensional protein structures of target proteins were obtained from Protein Data Bank. DOCK6 software package was used for the preparation of ligands and proteins. For the molecular dynamics study, the OPLS-2005 force field implemented in Desmond routine of Schrödinger software suite was used and the study was performed till 100 ns. Binding free energy (MM/ GBSA), Root Mean Square Deviation (RMSD) and Root Mean Square Fluctuation (RMSF) values of all complexes were determined. Principal component analysis and Detailed Cross-Correlation Maps were carried out.
Results and Discussion
Matairesinol showed good binding energies and docking scores with all the targets tested in this study with relatively stable molecular interactions, with the highest affinity shown towards ALK. The interactions with EGFR wild type, KRAS G12C mutant protein, VEGFR2, C-MET and ALK were especially of high stability.
Conclusion
The results of this study reveal the potential anti-cancer activity of Matairesinol in NSCLC with the possible added benefit of treating therapeutic resistance. Further studies are required to fully evaluate the target profile of Matairesinol in NSCLC and its therapeutic efficacy.
INTRODUCTION
Lung Cancer is the most reported cancer worldwide and is the primary cause of death related to cancer globally.1 It is of two types histologically- Small Cell and Non-Small Cell Lung Carcinoma (NSCLC) types. NSCLC represents around 85% of all cases worldwide.2 Somatic “driver mutations” (oncogenes) such as those of EGFR, KRAS, MET, BRAF, ALK and ROS1 have been found to contribute to the unchecked cellular proliferation and survival of tumour cells in NSCLC.3
The treatment options for advanced stages of NSCLC include Chemotherapy, radiotherapy, targeted therapy and immunotherapy.4 However, despite the leaps of advancement in the treatment of NSCLC, resistance to treatment has hindered therapeutic efficacy. Possible mechanisms of development of therapeutic resistance to chemotherapy agents include upregulation of EGFR, SPhk1 and PI3K- Akt pathways which may contribute to increased survival of tumour cells.5 Alarmingly, acquired resistance to targeted therapy too has been observed, placing therapeutic benefit in jeopardy.6 The on-target mechanisms for resistance to EGFR-TKIs include exon 19 deletions, L858R mutation, T790M mutation (for the first two generations)7 and acquired C797S mutation (for third-generation EGFR-TKIs).8 The Off-target mechanisms include Hypoxia Inducible Factor-1 pathway and MET amplification.9,10 Resistance to KRAS inhibitors such as Sotarasib can also be due to MET amplification.11 Resistance to immunotherapy has also developed, with causative factors including gene mutations (of ALK, EGFR and KRAS) and Neo-angiogenesis due to HIF1-α / VEGF pathways.12
The dibenzylbutyrolactone lignan, Matairesinol, sourced from theForsythia suspensa fruit and the marine seagrassHalophila stipulacea13,14 has shown anti-cancer activity against other cancers in vitro through mechanisms involving downregulation of Akt/ MAPK & ERK 1/2 pathways and inhibition of neo-angiogenesis.15–18 Akt/MAPK pathways are involved downstream of EGFR and KRAS pathways, while inhibition of angiogenesis may correlate with the ability of Matairesinol to modulate VEGF pathways. These very pathways are implicated in both pathogenesis and development of resistance to therapy in NSCLC. This in silico molecular docking and molecular dynamic simulation study was thus conducted to evaluate the binding affinity and stability of molecular interactions between Matairesinol and the molecular targets in NSCLC, thereby exploring its potential anticancer activity in NSCLC.
MATERIALS AND METHODS
The three-dimensional structure of Matairesinol was retrieved from the PubChem database. The three-dimensional protein structure of chosen targets- EGFR tyrosine kinase, VEGF, KRAS (wild), KRAS G12C mutant, AKT1, ALK, C-MET, EGFRC797S and EGFR- T790M, HIF1-α were retrieved from Protein Data Bank (PDB ID: 1M17, 6XVJ, 8AZV, 8AZX, 3O96, 3AOX, 5HTI, 8A27, 5XGM, 2WA3). DOCK6 software package was used for the preparation of ligands and proteins. MD simulation was done using the OPLS-2005 force field implemented in Desmond routine of the Schrödinger software suite.19,20 The orthorhombic periodic boxes with 10 Å3 were constructed using the TIP3P water association system as a buffer. The charge was neutralized by placing the charged ions (Na+/Cl–) isotopically. The system was minimized and the MD production was carried out up to 100 ns for the protein-ligand complexes and was performed in a 2 fs time step with the isothermal-isobaric ensemble (NPT), constant temperature (300 K) and pressure (1 bar). RMSD and RMSF of all the complexes were observed throughout the MD simulation. The PCA and the DCCM plots were created with the CPPTRAJ and R-package software.21,22 Binding free energy (MM/GBSA) was calculated from the fractions of simulation trajectories by the Prime application available in the Schrodinger software package.23–25
RESULTS
Matairesinol showed good corresponding docking scores with all the targets tested (Table 1) and corresponding highly negative binding energies – with the most negative binding energy seen with ALK. (Table 3).
Protein | Dock Score |
---|---|
EGFR tyrosine kinase | -40.8 |
VEGFR2 | -44.2 |
KRAS (wild-type) | -45.2 |
KRAS (G12C) | -45.8 |
AKT1 | -51.8 |
ALK | -40.8 |
C-MET | -44.2 |
EGFRC797S | -42.6 |
EGFRT790M | -41.1 |
HIF1-α | -30.9 |
Hydrogen bonding interactions (distances in Å) | Hydrophobic interactions (distances in Å) | |||
---|---|---|---|---|
EGFR tyrosine kinase | Dock | MD | Dock | MD |
Phe699 (3.1), Cys751 (2.9), Thr766 (3.1), Met769 (3.0), Gly772 (3.3), Cys773 (2.4), Asp776 (2.9), Leu820 (3.6), Thr830 (2.9), Asp831 (2.6) | Leu694 (2.7), Phe699 (3.5), Gly772 (2.9), Arg817 (2.5) | Cys751 (4.5), Ala719 (3.8), Met769 (5.5), Leu820 (4.7) | Leu694 (5.2), Phe699(5.7), Val702 (4.6), Cys773 (4.0), Leu820 (5.3) | |
VEGFR2 | Asp814 (2.8), Ala881 (2.8), Ser884 (3.5), Glu885 (3.0), Ile1025 (2.5), His1026 (2.7), Asp1046 (2.1), Gly1048 (2.8), Leu1049 (3.0) | Asp814 (2.3), Cys817 (2.8), Val899 (2.6), His1026 (3.4), Arg1027 (2.7), Ile1044 (2.6), Cys1045 (2.8), Asp1046 (3.1) | Ile888 (4.4) | Cys817 (4.1), Ile892 (4.3), Val899 (3.8), Leu1019 (4.5), His1026 (3.9), Arg1027 (4.4), Ile1044 (4.3) |
KRAS | Gly10 (3.5), Lys16 (2.3), Thr58 (3.3), Ala59 (3.2), Gly60 (2.9), Glu62 (2.6), Tyr64 (3.0), His95 (3.2) | Gly10 (2.0), Lys16 (1.9), Thr58 (2.7), Gly60 (2.6), Gln61 (з.0), Glu62 (2.0), Met72 (2.5), GDP201 (2.2) | Gln61 (4.9), Glu62 (4.9), Tyr64 (4.9), His95 (4.6) | — |
KRAS G12C | Gly10 (3.0), Cys12 (1.8), Lys16 (2.2), Pro34 (2.4), Thr58 (2.8), His95 (2.7), Tyr96 (2.6), | Gly10 (2.2), Thr58 (2.5), Gly60 (2.8), Gln61 (з.2), Glu63 (2.5), Met72 (3.3), Tyr96 (2.6), Gln99 (3.3) | Tyr64 (5.2), His95 (4.6), Tyr96 (4.7) | Tyr96 (4.6) |
AKT1 | Gln79 (2.9), Thr82 (3.5), Leu210 (2.8), Val271 (2.8), Tyr272 (2.8), Asp274 (3.3), Thr291 (3.5), Asp292 (2.9), Phe293 (3.0), Gly294 (3.3), Glu298 (3.2) | Asn53 (3.4), Asn54 (2.7), Gln79 (2.9), Trp80 (2.6), Thr82 (2.4), Lys268 (3.0), Val271 (2.6), Tyr272 (2.6), Arg273 (2.4), Asp292 (1.6), Leu295 (3.2), Cys296 (3.1) | Trp80 (4.0), Leu264 (5.0), Tyr272 (4.8), Asp274 (4.3), Asp292 (4.1) | Trp80 (5.2), Leu264 (5.0), Val270 (2.7), Tyr272 (4.6) |
ALK | Arg1120 (2.6), Leu1122 (2.8), Gly1123 (2.9), Lys1150 (3.2), Glu1197 (2.2), Met1199 (2.7), Ala1200 (2.7), Asp1203 (3.3), Leu1256 (2.8) | Leu1122 (2.3), Glu1123 (2.8), Ala1148 (2.5), Leu1196 (3.1), Glu1197 (2.3), Met1199 (2.3), Gly1202 (2.8) | Leu1122 (4.8), Val1130 (4.7), Ala1148 (4.0), Leu1256 (4.6) | Val1130 (4.5), Ala1148 (4.3), Val1180 (4.3), Leu1196 (4.1) |
C-MET | Lys1110 (3.4), Gln1123 (2.9), Glu1127 (3.4), Met1137 (2.8), Arg1203 (3.3), Asp1222 (2.8), Arg1227 (2.8) | Lys110 (3.4), Gln1123 (2.2), Met1131 (3.1), Leu1140 (3.3), Phe1200 (2.6), His1202 (2.5), Asp1222 (2.4), Leu1225 (2.8) | Met1131 (4.2), Arg1203 (4.1) | — |
EGFR C790S | Leu218 (2.6), Val726 (3.4), Glu762 (2.9), Met766 (2.5), Thr790 (2.7), Gln791 (2.8), Met793 (2.6), Pro794 (2.4), Leu844 (2.8), Thr854 (3.2) | Leu718 (2.5), Ser720 (3.5), Gln791 (3.6), Met793 (2.0), Gly796 (3.2), Ser797 (2.6), Leu799 (3.2), Arg841 (3.5), Thr854 (2.6) | Leu718 (4.1), Cys775 (3.7), Met793 (4.8) | Leu718 (4.1), Arg841 (3.9), Leu844 (4.3) |
EGFR C790M | Leu718 (2.7), Lys745 (2.9), Met79O (2.9), Gln791 (2.0), Met793 (3.0), Arg841 (2.7), Asn842 (2.6), Asp855 (2.6) | Leu718 (4.9), Ala743 (4.1), Met79O (5.0), Arg841 (4.4), Leu844 (5.0) | Pro741 (2.8), Leu792 (3.0), Phe795 (3.3), Lys846 (2.7), Thr847 (2.0), Val1011 (2.4), Asp1012 (3.0) | Pro794 (4.6), Val1010 (5.2) |
HIF1-α | Tyr102 (3.5), Thr183 (2.9), Serl84 (2.8), Asp201 (3.1), Gln2O3 (2.8), Asp237 (3.1), Arg238 (2.9), Gln239 (3.0), Trp296 (2.8) | Tyr102 (2.1), Tyr103 (1.8), Arg120 (3.0), Gln147 (2.5), Leu186 (2.5), Leu188 (3.5), Pro197 (2.5), His199 (3.0), Gln239 (2.1) | Tyr102 (4.5), Trp296 (4.3) | Tyr102 (4.5), His199 (4.0) |
Proteins | ΔG Bind | Coulomb | Covalent | H_bond | Lipo | vdW |
---|---|---|---|---|---|---|
(kcal/mol) | ||||||
EGFR (Tyrosine kinase) | -52.2 | -7.7 | 0.39 | -0.8 | -19.2 | -41.2 |
VEGFR2 | -42.9 | -8.7 | -0.18 | -0.62 | -15.6 | -43.5 |
KRASKRAS (G12C) | -38.9-47.2 | -19.7-11.8 | 14.03.5 | -0.4-1.2 | -35.2-24.2 | -25.9-37.1 |
AKT1 | -60.1 | -19.6 | 4.9 | -1.6 | -20.0 | -54.4 |
ALK | -167.7 | -124.0 | 2.6 | -9.2 | -25.5 | -89.3 |
C-MET | -55.1 | -8.3 | 2.9 | -0.6 | -19.7 | -48.4 |
EGFRC797S | -70.6 | -115.1 | 2.5 | -7.4 | -16.2 | -55.7 |
EGFRC797M | -67.7 | -185.0 | 3.7 | -8.4 | -13.4 | -52.5 |
HIF1-α | -42.0 | -13.6 | 3.0 | -0.96 | -20.2 | -39.1 |
Matairesinol-EGFR tyrosine kinase complex
The molecular docking analysis revealed that Matairesinol formed strong interactions with the active site amino acids of the EGFR tyrosine kinase enzyme (Figure 1B). Matairesinol was able to form strong interactions with ATP binding site loop amino acids including Leu694, Phe699, Gly772, Cys773, Arg817 and Leu820 (Figure 1C). The RMSD and RMSF values were below 4 Å, confirming the relative stability of the complex structure over MD simulation (Figure 1D and 1F). The DCCM plots indicated the presence of pair pairwise correlation between Matairesinol and EGFR tyrosine kinase enzyme (Figure 1G). The corresponding PC3 value (Figure S1A) was 8.31% which denotes the low mobility and high stability of the complex structure. The intermolecular interactions revealed that Matairesinol is highly stable in the active site of EGFR for up to 100 ns in MD simulation (Table 2). The respective Coloumb, hydrophobic and van der Waals interactions energy values were very low and contributed to the low binding energy values (-52.2 kcal/mol) (Table 3).
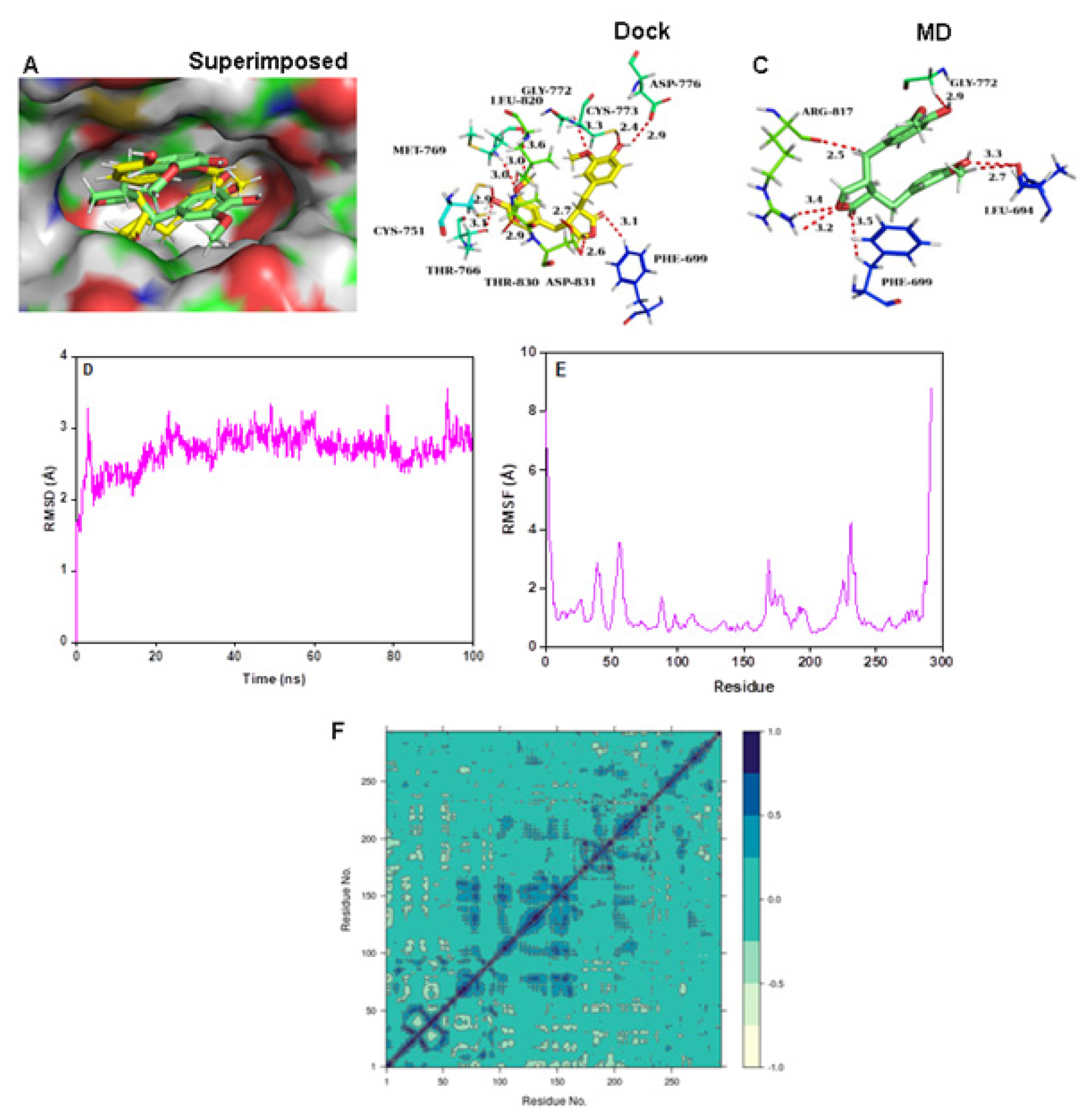
Figure 1:
(A) Connolly representations of Matairesinol-EGFR tyrosine kinase, Intermolecular interactions between Matairesinol and EGFR tyrosine kinase after docking (B) and 100 ns MD simulation (C) RMSD (D) RMSF (E) and DCCM plot (F) of Matairesinol-EGFR tyrosine kinase complex throughout MD simulation.
Matairesinol-EGFR (C797S and T790M) mutant complexes
Matairesinol formed strong interactions with the active site amino acids of mutant EGFRC797S protein (Figure 2A-2C) while it shifted from the active site of EGFRCT790M enzyme (Figure 2D-2F). Further studies showed that Matairesinol exhibited instability at the active site of the EGFR T790M mutant protein (Figure 2G-2H). DCCM plots reveal the distribution of pairwise cross-correlating residues over the Matairesinol-EGFR C797S complex and anti-pairwise cross-correlating residues in the case of Matairesinol-EGFR T790M complex (Figure 2I and 3J). The PCA plots showed the high solidity of the Matairesinol-EGFRC797S mutant complex over the Matairesinol-EGFRT790M complex (Figure S1B and S1C). EGFRC797S mutation has been known to mediate resistance to third-generation TKIs26 and the affinity and stable interactions shown by Matairesinol to this target are especially encouraging.
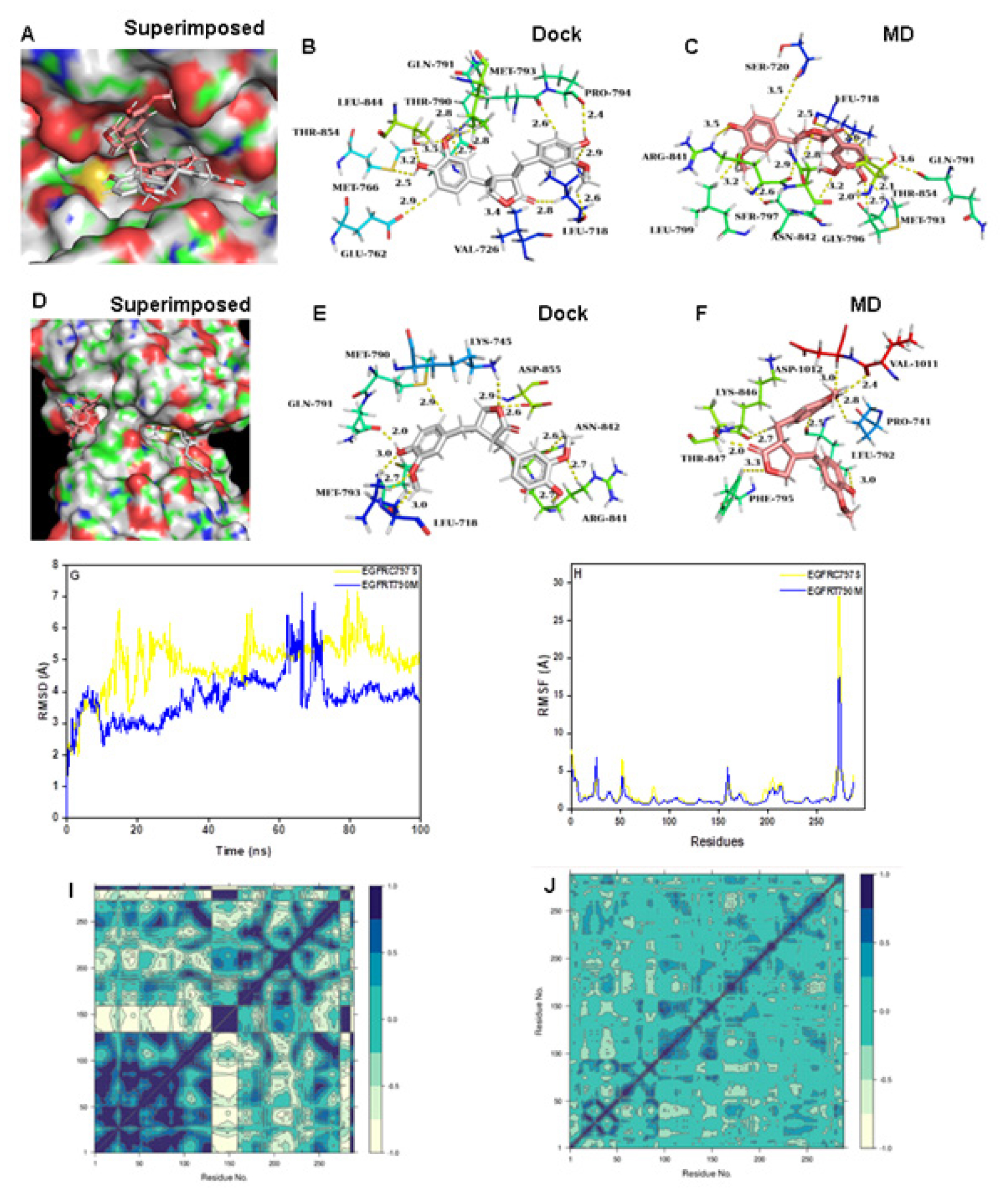
Figure 2:
(A) Connolly representations of Matairesinol-EGFRC797S, Intermolecular interactions between Matairesinol and EGFRC797S after docking (B) and 100 ns MD simulation (C). Connolly representations (D) and intermolecular interactions between Matairesinol and EGFRT790M after docking (E) and 100 ns MD simulation (F) RMSD (G) RMSF (H) and DCCM plot (I) of Matairesinol-EGFRC797S and Matairesinol-EGFRT790M complexes throughout MD simulation.
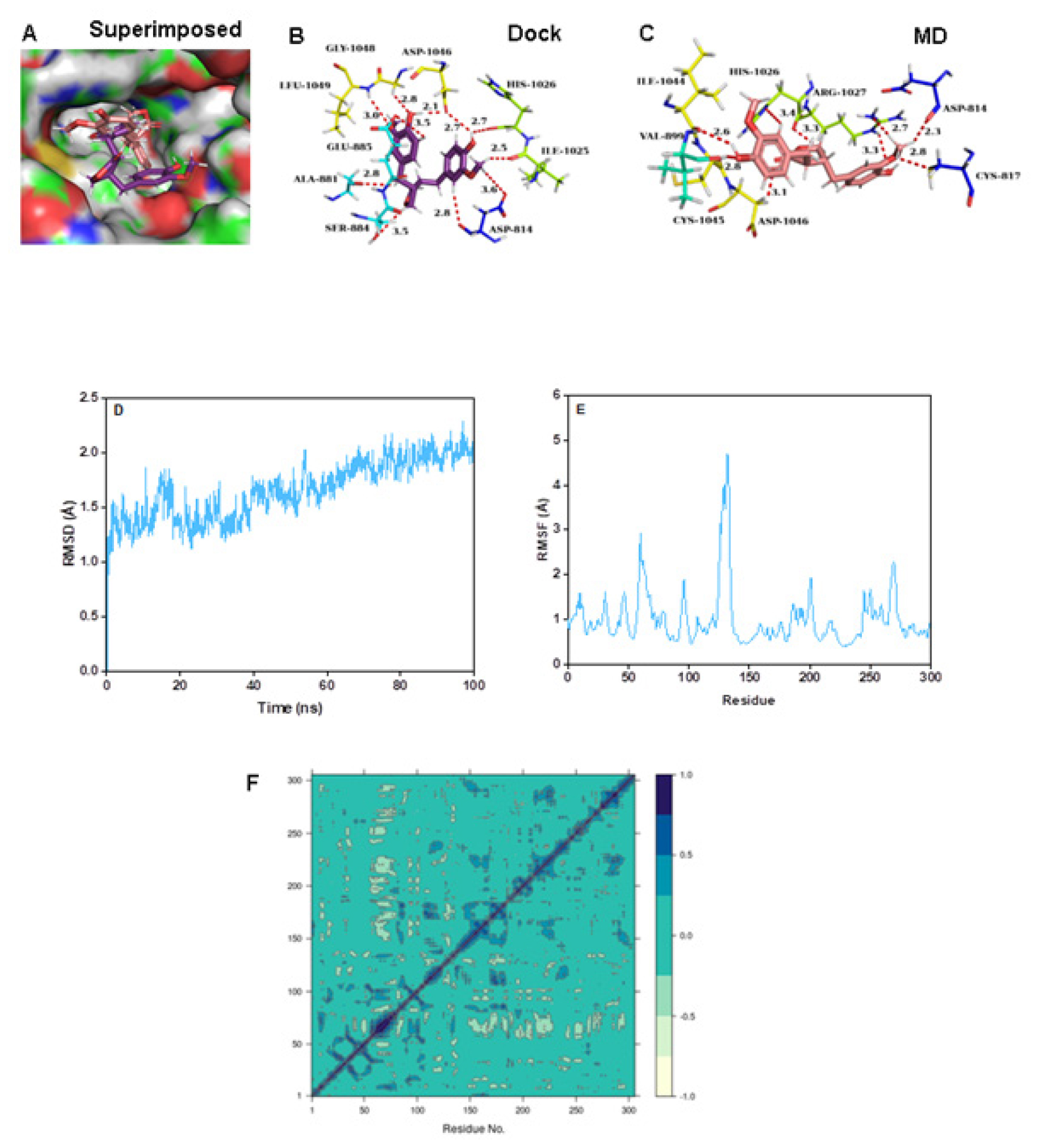
Figure 3:
(A) Connolly representations of Matairesinol-VEGFR2, Intermolecular interactions between Matairesinol and VEGFR2 after docking (B) and 100 ns MD simulation (C) RMSD (D) RMSF (E) and DCCM plot (F) of Matairesinol- VEGFR2 complex throughout MD simulation.
Matairesinol-KRAS (wild and G12C) mutant complexes
Matairesinol formed strong interactions with P-loop and switch II residues of the wild and G12C mutant enzymes and the intermolecular interactions are almost similar for both cases (Figure 4A-4F). Further studies showed that Matairesinol exhibits high firmness at the active site of the G12C mutant protein (Figure 4G and 4H). Especially, the DCCM plot reveals the distribution of anti-pairwise cross-correlating residues over wild-type complex and pairwise cross-correlating residues over Matairesinol G12C mutant KRAS enzyme complex (Figure 4I and 4J). The PCA plots show the high solidity of the mutant complex over the wild type (FigureS1E and S1F). This interaction is especially important, since KRAS G12C has historically been heralded as an “undruggable target”,28 until the development of drugs like Sotarasib which bind to the switch II domain. The good binding interactions shown by Matairesinol to the same domain are promising.
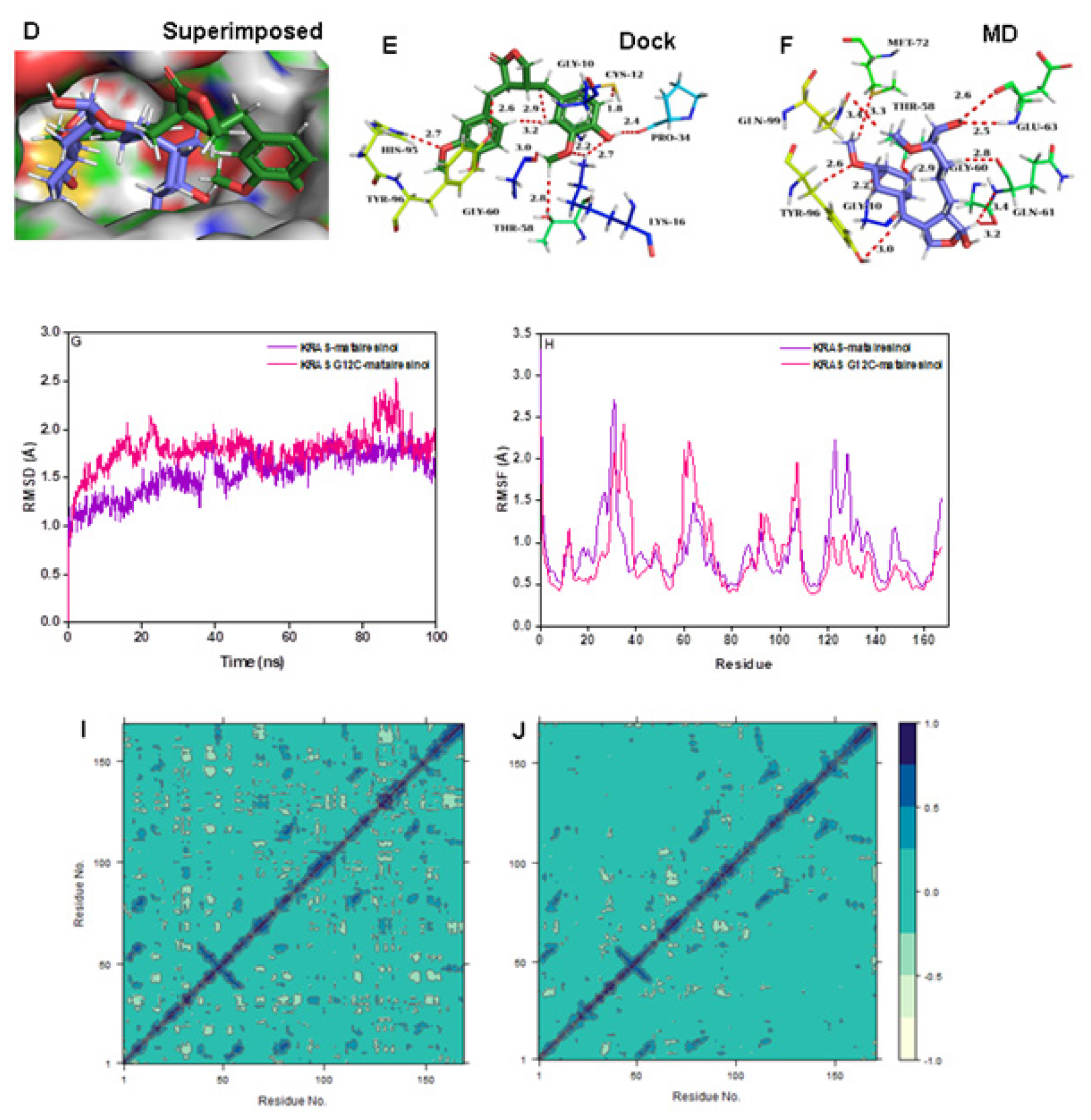
Figure 4:
(A) Connolly representations of Matairesinol-wild-type KRAS, Intermolecular interactions between Matairesinol and wild-type KRAS after docking (B) and 100 ns MD simulation (C) Connolly representations (D) and intermolecular interactions between Matairesinol and KRAS G12C after docking (E) and 100 ns MD simulation (F) RMSD (G) RMSF (H) and DCCM plot (I, J) of Matairesinol-wild-type KRAS and Matairesinol- KRAS G12C complexes throughout MD simulation.
Matairesinol-AKT1 complex
Matairesinol formed intermolecular contacts with the active site amino acids of AKT1 enzyme with MD simulation revealing strong interaction over the active site (Figure 5A-5C). The intermolecular interactions of Matairesinol with the active site residues during MD simulation showed the inhibition properties of the molecule against the AKT1 enzyme (Table 2). The corresponding RMSD (below 5 Å) and RMSF values are quite low specifying the stability of the complex structure (Figure 5D and 5E). In addition, the DCCM plot shows the presence of pairwise correlating residues is dominant during MD simulation (Figure 5F). The PC3 value is 8.36% which reveals the high steadiness of the complex system and the PCA plot of the complex is shown in Figure S1G.
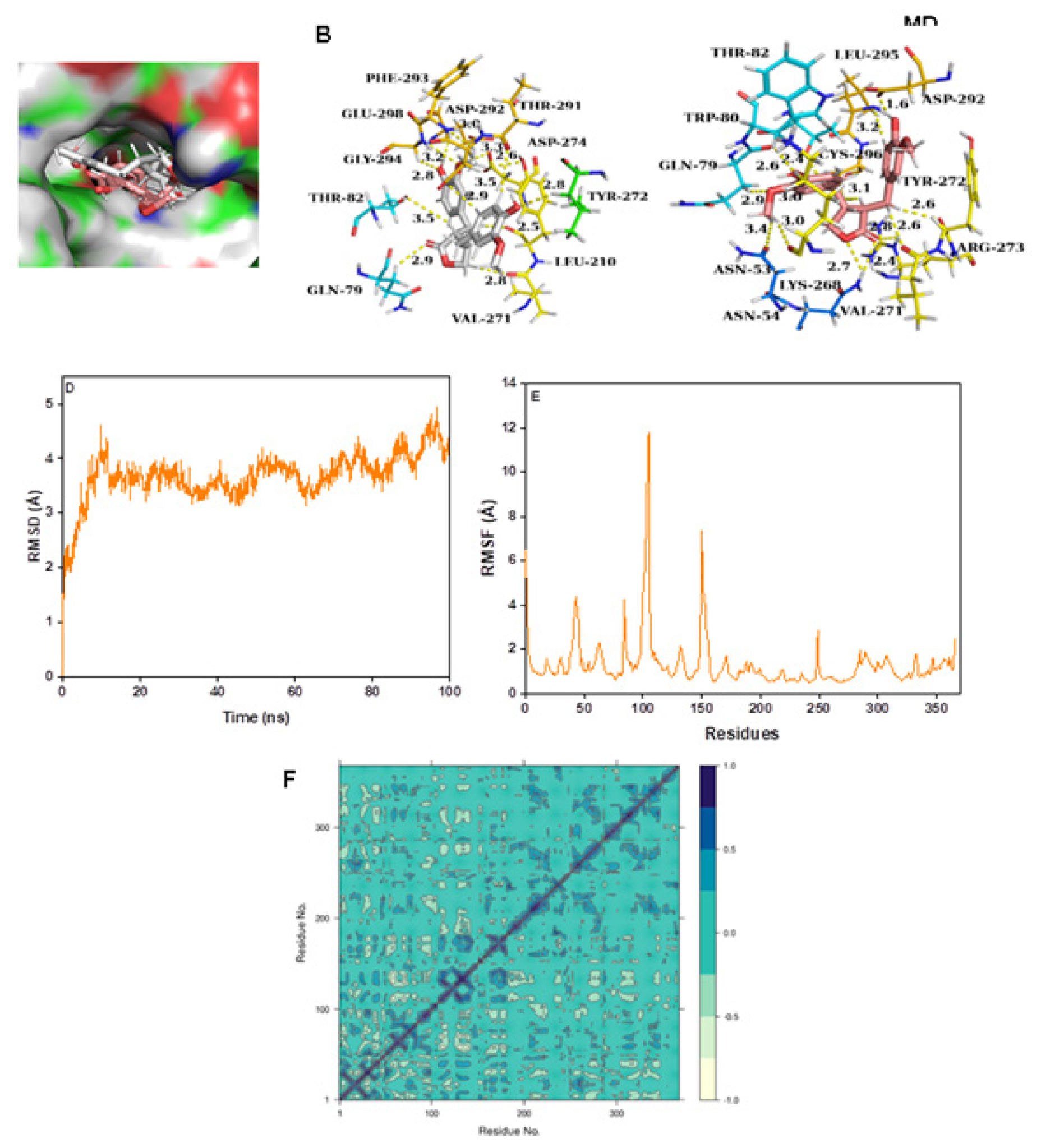
Figure 5:
(A) Connolly representations of Matairesinol-AKT1, Intermolecular interactions between Matairesinol and AKT1 after docking (B) and 100 ns MD simulation (C) RMSD (D) RMSF (E) and DCCM plot (F) of Matairesinol-AKTM complex throughout MD simulation.
Matairesinol-ALK complex
Matairesinol interacted in the active site of ALK after docking and MD simulation (Figure 6A-6C). The RMSD value of the complex was in the range of 2.5 Å and the corresponding RMSF values were quite low for the active site amino acids (Figure 6D and 6E). Hence the complex was stable during the MD simulation. The presence of more pairwise correlating residues than anti-pairwise correlation was seen (Figure 6F) with a low PCA value (8.25%) (Figure S1H). The complex also exhibited the lowest binding energy of all the complexes in the present study (Table 3).
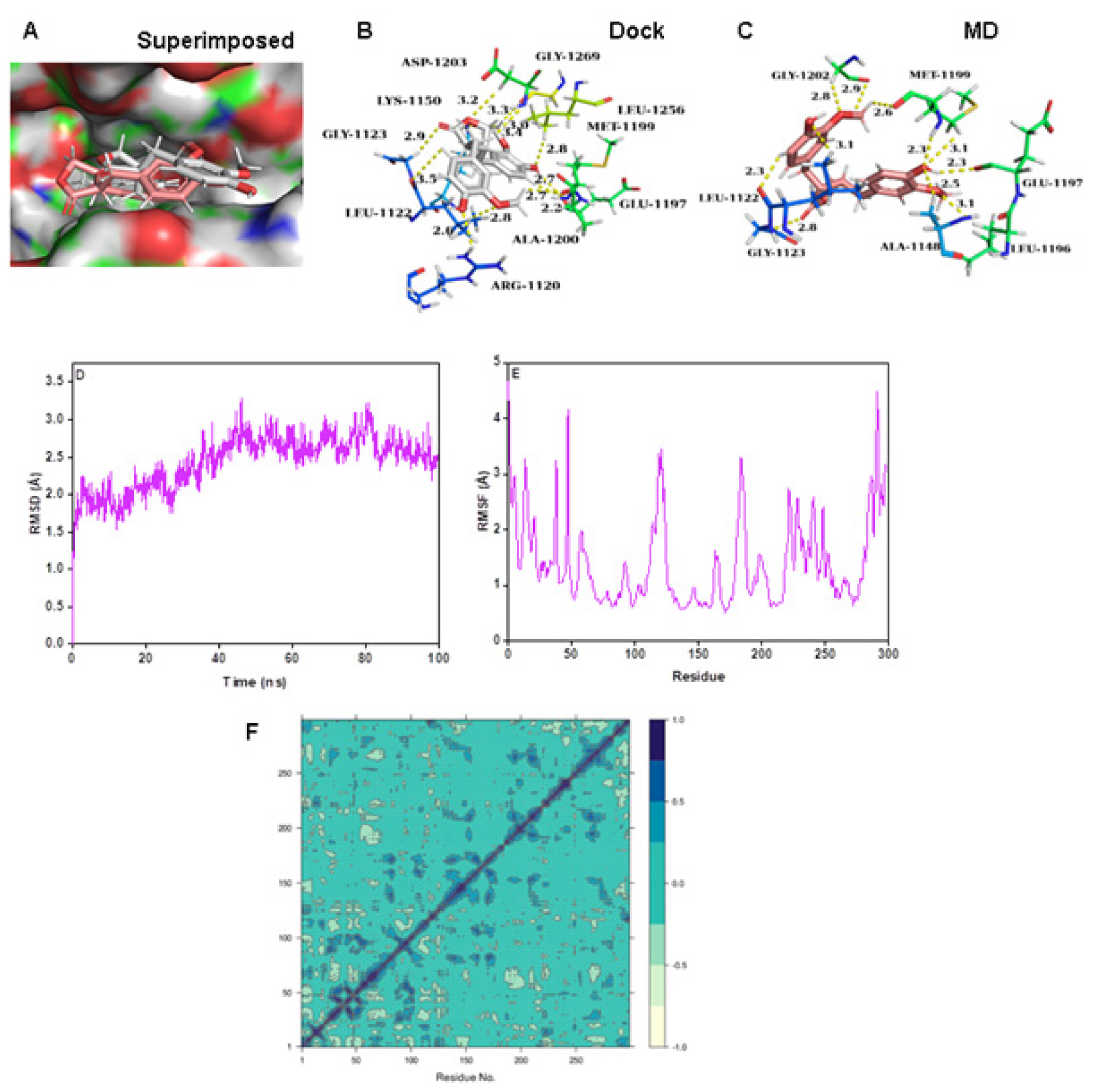
Figure 6:
(A) Connolly representations of Matairesinol-ALK, Intermolecular interactions between Matairesinol and ALK after docking (B) and 100 ns MD simulation (C) RMSD (D) RMSF (E) and DCCM plot (F) of Matairesinol-ALK complex throughout MD simulation.
Matairesinol-C-MET complex
The RMSD and RMSF values were below 3 Å, confirming the stability of the complex structure over MD simulation (Figure 7D and 7E). The DCCM plots indicated the presence of a large pairwise correlation between Matairesinol and the C-MET enzyme (Figure 7F). The corresponding PC3 value was 7.27% which denoted the low mobility and high stability of the complex structure (Figure S1I). The respective coulomb, hydrophobic and van der Waals interactions energy values were very low and contributed to the low binding energy values (-55.1 kcal/mol), respectively denoting the stability of the complex (Table 3).
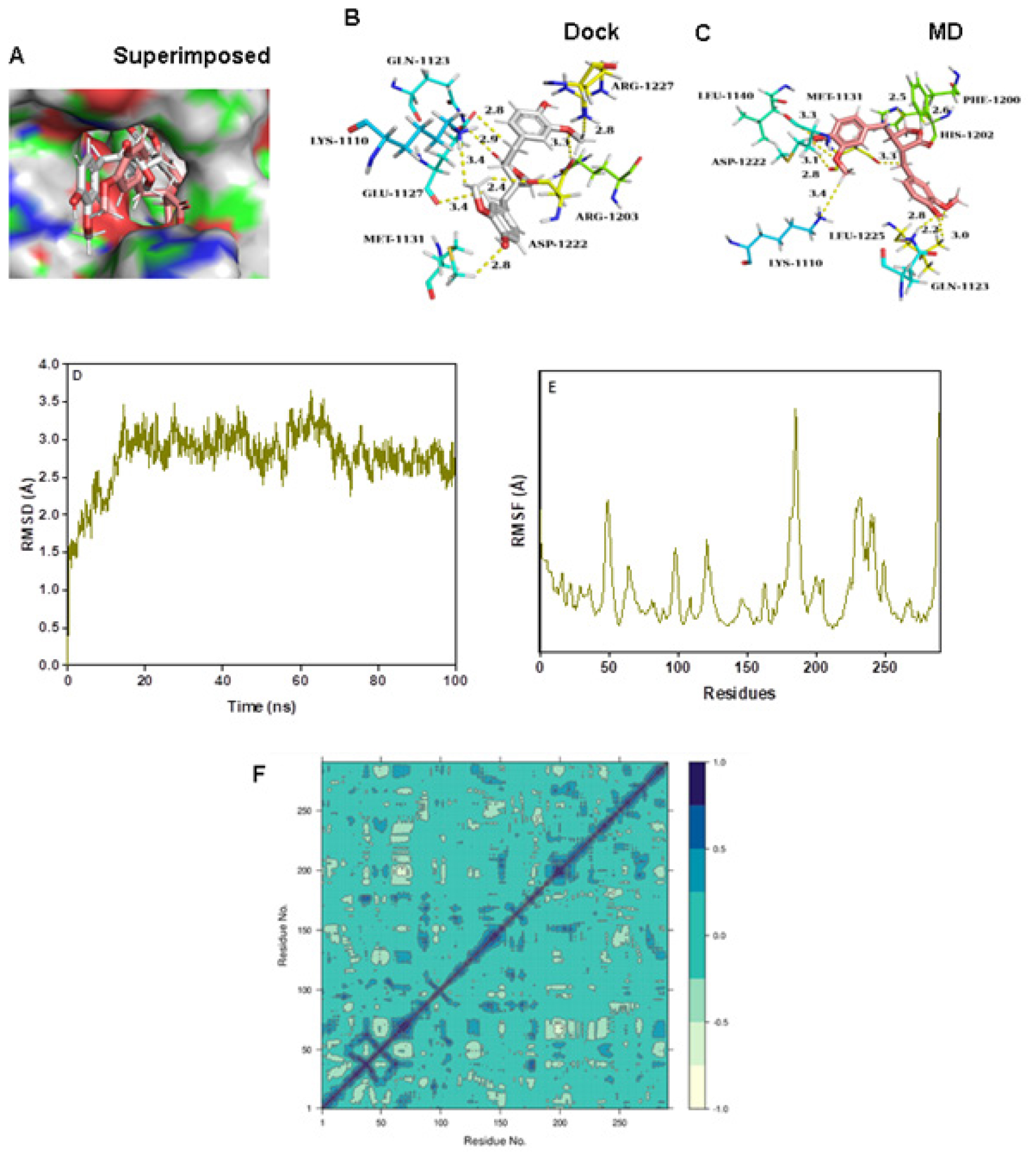
Figure 7:
(A) Connolly representations of Matairesinol-C-MET, Intermolecular interactions between Matairesinol and C-MET after docking (B) and 100 ns MD simulation (C) RMSD (D, RMSF (E) and DCCM plot (F) of Matairesinol- C-MET complex throughout MD simulation.
Matairesinol-HIF-α complex
The molecular docking analysis revealed that Matiresinol forms intermolecular contacts with the active site amino acids of the HIF-α enzyme (Figure 8A and 8B). Further MD simulation revealed that the molecule forms a strong interaction with the active site at the end of 100 ns MD simulation (Figure 8C). The corresponding RMSD (below 3.5 Å) and RMSF values are quite low specifying the stability of the complex structure (Figure 8D and 8E). In addition, the DCCM plot denotes the presence of pairwise correlating residues is dominant during MD simulation (Figure 8F). The PC3 value is 6.83% which reveals the high steadiness of the complex system (Figure S1J). The corresponding MMPBSA free energy value was also quite low at -42.0 kcal/mol (Table 3).
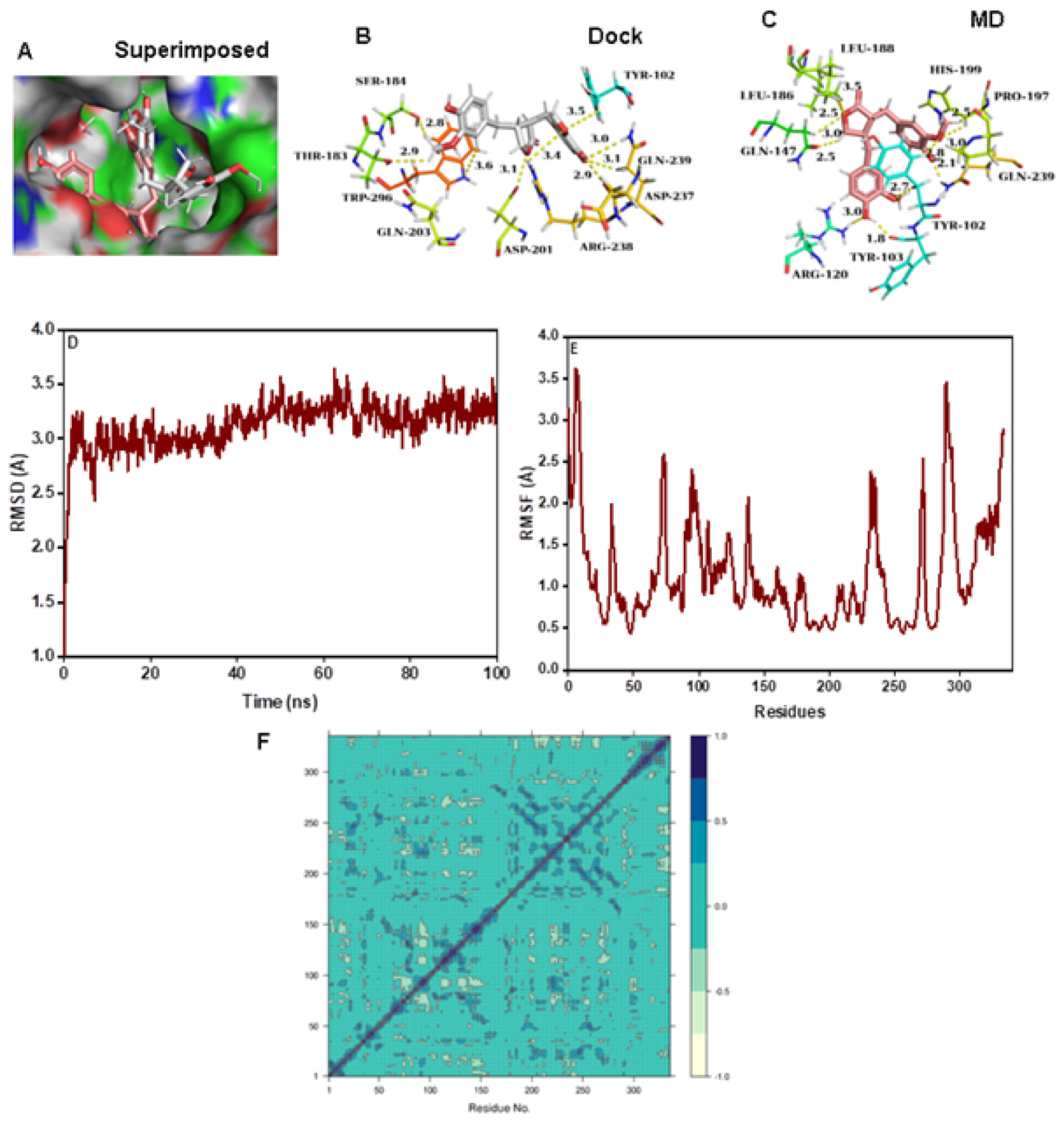
Figure 8:
(A) Connolly representations of Matairesinol-HIF-1α, Intermolecular interactions between Matairesinol and HIF-1α after docking (B) and 100 ns MD simulation (C). RMSD (D) RMSF (E) and DCCM plot (F) of Matairesinol- HIF-1α complex throughout MD simulation.
DISCUSSION
In toto, Matairesinol showed good docking scores and binding energies with all the targets, with maximum affinity to ALK. ALK-positive cases account for 3-7% of all NSCLC cases.29 Although the mutant fusion protein complexes of ALK such as EMLA4- ALK were not taken as targets in the study potential action of Matairesinol on the ALK pathway does open doors to further studies with specific proteins in this pathway. The molecular dynamics simulation showed that the interactions of Matairesinol with EGFR wild type, KRAS G12C mutant protein, VEGFR, C-MET and ALK were especially stable, showing lower RMSD and RMSF values. Matairesinol has previously shown anti-cancer activity through inhibition of PI3-AKT and ERK 1/2 signaling-this study revealed the affinity to the targets upstream to these pathways namely EGFR, KRAS and MET. The interesting finding in this study was the affinity shown by Matairesinol to the mutant KRAS G12C and EGFR -C797S proteins-both of which are implicated in therapeutic resistance to targeted therapies in NSCLC. The good binding energy as well as stable interactions shown by Matairesinol with VEGFR2 and HIF1-α may also contribute towards its potential role in treating resistance to immunotherapy in NSCLC. The limitations of this study included not using standard drugs against each target as controls. Also, the targets in this study were chosen based on previous in-vitro anti-cancer studies using Matairesinol-a more relevant and comprehensive method of establishing targets, such as Network Pharmacology can be used in further studies.
CONCLUSION
Matairesinol showed good binding energies and docking scores with all the targets tested in this study with relatively stable molecular interactions. The interactions with EGFR wild type, KRAS G12C mutant protein, VEGFR2, C-MET and ALK were especially of high stability. All the targets tested in this study are implicated either directly in the pathogenesis of NSCLC or in the development of therapeutic resistance-thus underlining the potential role of Matairesinol as an anti-cancer agent in NSCLC with the additional benefit of curbing treatment resistance. Further in silico studies are required to fully explore the target profile of Matairesinol in NSCLC and further in-vitro and in-vivo studies are needed to explore efficacy against the targets tested in this study.
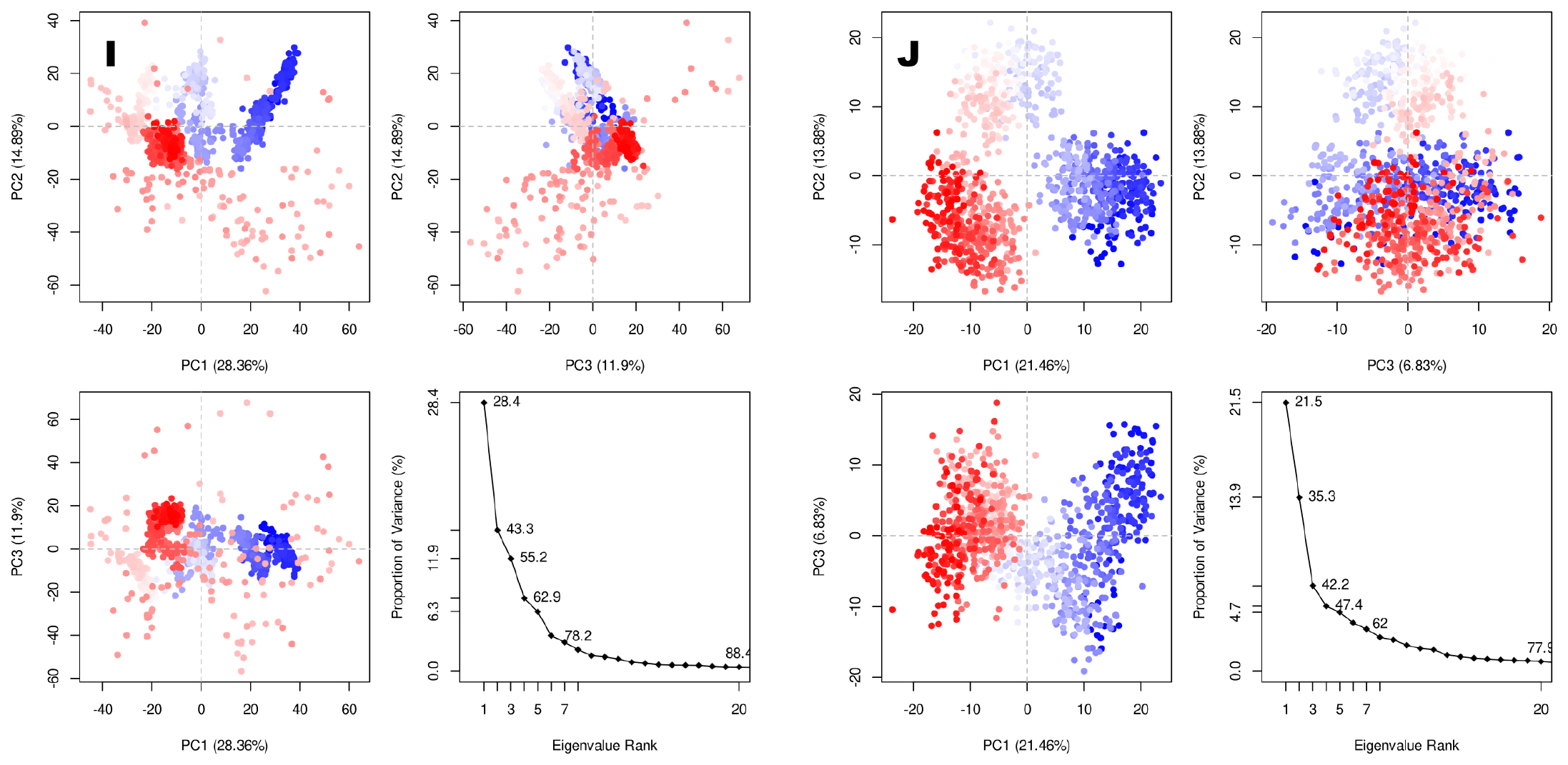
Figure S1:
PCA plots of (A) matairesinol-EGFR tyrosine kinase, (B) matairesinol-VEGFR2, (C) matairesinol-KRAS and (D) matairesinol-KRAS G12C (mutant) ) matairesinol-AKT1 (F) matairesinol-ALK (G) matairesinol-C-MET (H) matairesinol-EGFRC790S (I) matairesinol-EGFRC790M and (J) matairesinol-HIF1-α enzyme complexes.
Cite this article:
Kamalarathnam BR, Karthik VP, Sowmya P, Ramasamy K. In silico Molecular Docking and Molecular Dynamics Simulation Analysis of Matairesinol with Molecular Targets in Non-Small Cell Lung Carcinoma. Int. J. Pharm. Investigation. 2024;14(3):857-72.
ACKNOWLEDGEMENT
The authors would like to express heartfelt gratitude to the Department of Pharmacology, SRMC & RI for the support and motivation provided
The authors would like to express heartfelt gratitude to the Department of Pharmacology, SRMC & RI for the support and motivation provided
ABBREVIATIONS
EGFR | Epidermal Growth Factor Receptor |
---|---|
KRAS | Kirsten Rat Sarcoma virus oncogene |
cMET | Mesenchymal Epithelial Transition factor |
ALK | Anaplastic Lymphoma Kinase protein |
ROS1 | Proto-oncogene tyrosine-protein kinase ROS |
SPhk1 | Sphingosine kinase 1 |
PI3K | Phosphoinositide 3-kinase |
VEGFR2 | Vascular Endothelial Growth Factor Receptor 2 |
ERK ½ | Extracellular Signal-Regulated Kinases |
MAPK | Mitogen-activated protein kinase |
MD | Molecular Dynamics |
RMSD | Root Mean Square Deviation |
RMSF | Root Mean Square Fluctuation |
PCA | Principal Component Analysis |
DCCM | Detailed Cross-Correlation Map |
MMGBSA | Molecular Mechanics-Generalized Born Surface Area |
ΔG bind | free energy of the binding |
H bond | Hydrogen Bond |
Lipo | Lipophilic energy |
kcal/mol | Kilocalorie/mole |
ns | Nanosecond |
Coulomb | Coulomb Energy |
Covalent | Covalent Binding Energy |
vdW | Van der Waals Energy |
References
- Leiter A, Veluswamy RR, Wisnivesky JP. The global burden of lung cancer: current status and future trends. Nat Rev Clin Oncol. 2023;20(9):624-39. Epub 2023 Jul 21 [PubMed] | [CrossRef] | [Google Scholar]
- Alduais Y, Zhang H, Fan F, Chen J, Chen B. Non-small cell lung cancer (NSCLC): A review of risk factors, diagnosis and treatment. Medicine (Baltimore). 2023;102(8):e32899 [PubMed] | [CrossRef] | [Google Scholar]
- McLaughlin J, Berkman J, Nana-Sinkam P. Targeted therapies in non-small cell lung cancer: present and future. Fac Rev. 2023;12:22 [PubMed] | [CrossRef] | [Google Scholar]
- Alexander M, Kim SY, Cheng H. Update 2020: Management of Non-Small Cell Lung Cancer. Lung. 2020;198(6):897-907. Epub 2020 Nov 11 [PubMed] | [CrossRef] | [Google Scholar]
- Stewart DJ. Mechanisms of resistance to cisplatin and carboplatin. Crit Rev Oncol Hematol. 2007;63(1):12-31. Epub 2007 Mar 1 [PubMed] | [CrossRef] | [Google Scholar]
- Wu SG, Shih JY. Management of acquired resistance to EGFR TKI-targeted therapy in advanced non-small cell lung cancer. Mol Cancer. 2018;17(1):38 [PubMed] | [CrossRef] | [Google Scholar]
- Yu HA, Arcila ME, Rekhtman N, Sima CS, Zakowski MF, Pao W, et al. Analysis of tumor specimens at the time of acquired resistance to EGFR-TKI therapy in 155 patients with EGFR-mutant lung cancers. Clin Cancer Res. 2013;19(8):2240-7. Epub 2013 Mar 7 [PubMed] | [CrossRef] | [Google Scholar]
- Oxnard GR, Hu Y, Mileham KF, Husain H, Costa DB, Tracy P, et al. Assessment of Resistance Mechanisms and Clinical Implications in Patients With EGFR T790M-Positive Lung Cancer and Acquired Resistance to Osimertinib. JAMA Oncol. 2018;4(11):1527-34. [PubMed] | [CrossRef] | [Google Scholar]
- Passaro A, Jänne PA, Mok T, Peters S. Overcoming therapy resistance in EGFR-mutant lung cancer. Nat Cancer. 2021;2(4):377-91. Epub 2021 Apr 15 [PubMed] | [CrossRef] | [Google Scholar]
- Jin Q, Zheng J, Chen M, Jiang N, Xu X, Huang F, et al. HIF-1 Inhibitor YC-1 Reverses the Acquired Resistance of EGFR-Mutant HCC827 Cell Line with MET Amplification to Gefitinib. Oxid Med Cell Longev. 2021;2021:6633867 [PubMed] | [CrossRef] | [Google Scholar]
- Koga T, Suda K, Fujino T, Ohara S, Hamada A, Nishino M, et al. KRAS Secondary Mutations That Confer Acquired Resistance to KRAS G12C Inhibitors, Sotorasib and Adagrasib and Overcoming Strategies: Insights From In Vitro Experiments. J Thorac Oncol. 2021;16(8):1321-32. Epub 2021 May 7 [PubMed] | [CrossRef] | [Google Scholar]
- Ashrafi A, Akter Z, Modareszadeh P, Modareszadeh P, Berisha E, Alemi PS, et al. Current Landscape of Therapeutic Resistance in Lung Cancer and Promising Strategies to Overcome Resistance. Cancers (Basel). 2022;14(19):4562 [PubMed] | [CrossRef] | [Google Scholar]
- Sung YY, Lee AY, Kim HK. Forsythia suspensa fruit extracts and the constituent matairesinol confer anti-allergic effects in an allergic dermatitis mouse model. J Ethnopharmacol. 2016;187:49-56. Epub 2016 Apr 13 [PubMed] | [CrossRef] | [Google Scholar]
- Bel Mabrouk S, Reis M, Sousa ML, Ribeiro T, Almeida JR, Pereira S, et al. The Marine Seagrass as a Source of Bioactive Metabolites against Obesity and Biofouling. Mar Drugs. 2020;18(2):88 [PubMed] | [CrossRef] | [Google Scholar]
- Lee W, Song G, Bae H. Matairesinol Induces Mitochondrial Dysfunction and Exerts Synergistic Anticancer Effects with 5-Fluorouracil in Pancreatic Cancer Cells. Mar Drugs. 2022;20(8):473 [PubMed] | [CrossRef] | [Google Scholar]
- Wu S, Wang J, Fu Z, Familiari G, Relucenti M, Aschner M, et al. Matairesinol Nanoparticles Restore Chemosensitivity and Suppress Colorectal Cancer Progression in Preclinical Models: Role of Lipid Metabolism Reprogramming. Nano Lett. 2023;23(5):1970-80. Epub 2023 Feb 20 [PubMed] | [CrossRef] | [Google Scholar]
- Peuhu E, Rivero-Müller A, Stykki H, Torvaldson E, Holmbom T, Eklund P, et al. Inhibition of Akt signaling by the lignan matairesinol sensitizes prostate cancer cells to TRAIL-induced apoptosis. Oncogene. 2010;29(6):898-908. Epub 2009 Nov 23 [PubMed] | [CrossRef] | [Google Scholar]
- Chang H, Wang Y, Gao X, Song Z, Awale S, Han N, et al. Lignans from the root of Wikstroemia indica and their cytotoxic activity against PANC-1 human pancreatic cancer cells. Fitoterapia. 2017;121:31-7. Epub 2017 Jun 16 [PubMed] | [CrossRef] | [Google Scholar]
- Vijayan S, Loganathan C, Sakayanathan P, Thayumanavan P. Array. J Biomol Struct Dyn. 2022;40(22):11511-25. Epub 2021 Aug 3 [PubMed] | [CrossRef] | [Google Scholar]
- Bowers KJ, Chow E, Xu H, Dror RO, Eastwood MP, Gregersen BA, Klepeis JL, Kolossvary I, Moraes MA, Sacerdoti FD, Salmon JK, et al. Scalable algorithms for molecular dynamics simulations on commodity clusters. InProceedings of the 2006 ACM/IEEE Conference on Supercomputing. 2006:84-es. [CrossRef] | [Google Scholar]
- Ashraf N, Asari A, Yousaf N, Ahmad M, Ahmed M, Faisal A, Saleem M, Muddassar M, et al. Combined 3D-QSAR, molecular docking and dynamics simulations studies to model and design TTK inhibitors. Front Chem. 2022;10:1003816 [PubMed] | [CrossRef] | [Google Scholar]
- Roe DR, Cheatham TE. PTRAJ and CPPTRAJ: Software for Processing and Analysis of Molecular Dynamics Trajectory Data. J Chem Theory Comput. 2013;9(7):3084-95. Epub 2013 Jun 25 [PubMed] | [CrossRef] | [Google Scholar]
- Li J, Abel R, Zhu K, Cao Y, Zhao S, Friesner RA, et al. The VSGB 2.0 model: a next generation energy model for high resolution protein structure modeling. Proteins. 2011;79(10):2794-812. Epub 2011 Aug 22 [PubMed] | [CrossRef] | [Google Scholar]
- Wang E, Sun H, Wang J, Wang Z, Liu H, Zhang JZH, et al. End-Point Binding Free Energy Calculation with MM/PBSA and MM/GBSA: Strategies and Applications in Drug Design. Chem Rev. 2019;119(16):9478-508. Epub 2019 Jun 24 [PubMed] | [CrossRef] | [Google Scholar]
- Genheden S, Ryde U. The MM/PBSA and MM/GBSA methods to estimate ligand-binding affinities. Expert Opin Drug Discov. 2015;10(5):449-61. Epub 2015 Apr 2 [PubMed] | [CrossRef] | [Google Scholar]
- Wang S, Tsui ST, Liu C, Song Y, Liu D. EGFR C797S mutation mediates resistance to third-generation inhibitors in T790M-positive non-small cell lung cancer. J Hematol Oncol. 2016;9(1):59 [PubMed] | [CrossRef] | [Google Scholar]
- Rampogu S, Baek A, Zeb A, Lee KW. Exploration for novel inhibitors showing back-to-front approach against VEGFR-2 kinase domain (4AG8) employing molecular docking mechanism and molecular dynamics simulations. BMC Cancer. 2018;18(1):264 Erratum in: BMC Cancer. 2019; 19(1):1249 [PubMed] | [CrossRef] | [Google Scholar]
- Huang L, Guo Z, Wang F, Fu L. KRAS mutation: from undruggable to druggable in cancer. Signal Transduct Target Ther. 2021;6(1):386 [PubMed] | [CrossRef] | [Google Scholar]
- Peng L, Zhu L, Sun Y, Stebbing J, Selvaggi G, Zhang Y, et al. Targeting ALK Rearrangements in NSCLC: Current State of the Art. Front Oncol. 2022;12:863461 [PubMed] | [CrossRef] | [Google Scholar]