ABSTRACT
Precision medicine in diabetes management is a groundbreaking approach that tailors treatment strategies to individual patients, moving beyond the one-size-fits-all model. It leverages genetic, molecular and lifestyle data to personalize care. The complex interactions between genetics, the environment and lifestyle decisions that lead to diabetes growth and progression are understood through the theoretical framework. Numerous susceptibility genes have been identified thanks to genomic research, which facilitates risk assessment and customized therapies. Early disease identification has been made easier to detect biomarker. The integration of many data sources for accurate patient profile is possible by cutting-edge technologies like machine learning and data analytics. A thorough understanding of disease mechanisms has been made possible by the inclusion of omics data, genomics and metabolomics, paving the way for the creation of tailored treatments. This involves identifying the precise diabetes subtype of a patient, predicting how they will react to certain drugs and creating a specialized diet and exercise regimen. Wearable technologies and continuous glucose monitoring improve real-time data collecting, enabling quick modifications. Pharmacogenomics also aids in selecting the best treatments while reducing adverse effects. Precision medicine gives medical professionals the ability to improve outcomes and avoid problems, eventually raising the quality of life for diabetics. It represents a paradigm change in the treatment of diabetes, allowing for more focused therapies and effective control.
INTRODUCTION
The classification of patients into categories based on clinical criteria, molecular and hereditary biomarkers and other factors in order to optimize therapeutic results is known as precision medicine. The focus of precision medicine has been expanded to encompass environmental and behavioural elements in addition to genetics, with suggestions focusing on populations instead of single patients (Gloyn and Drucker, 2018). The use of data-driven healthcare can be seen as the future of medicine; however, the practice of individualized medicine is not new. Precision medicine is a movement that takes account of each person’s unique genetic makeup when determining the best course of treatment and/or prevention. Pharmaceutical specialists can forecast a person’s response to a specific drug and/or treatment through the study of the human genome and its environmental factors, enhancing the standard of care given to patients. Diabetes is notoriously difficult to prevent and cure because of its complexity (Ni Kiet al., 2022). The specialized and intensive treatment that helps diabetic expecting mothers achieve glycaemic objectives and optimize pregnancy outcomes may be jeopardized by COVID-19-induced restrictions that prevent face-to-face patient-provider conversation. Maintaining an emphasis on patient-centered patient-provider communication therefore becomes crucial during this period of transition from face-to-face ambulatory care to virtual care (Sushkoet al., 2023). Dealing with data in medicine presents many difficult problems, privacy being one of them. Another important challenge and usually the biggest one in the situation is data collection. Privacy is a serious issue that arises with the adoption of big data techniques or systems. Medical data are much more sensitive than data from other sources. The accessibility of information is a key factor in machine learning algorithms. Without openly accessible data, it is difficult for researchers to conduct analysis to boost the effectiveness of the present and future healthcare systems (Ni Kiet al., 2022). Individuals have particular physical qualities, emotional demands and problems and environmental factors that affect their capacity for and desire to carry out a variety of everyday chores to regulate their blood sugar levels and enhance their general health. When there were few medications and tools available, it was acceptable for practitioners to use a “one size fits all” approach to patient care. This strategy has long since lost its value. In addition to improving glycemia management and multiple complications of diabetes, new drugs and cutting-edge technology also offer chances to tailor treatment to the unique demands and circumstances of each patient (Midyett, 2023). Different people present with T2D in different ways; some present with the disease for a short period, some for a long time and some present with additional comorbidities. Therefore, “a single size does not fit all” when it comes to therapy. More recently, the American Diabetes Association (ADA) and European Association for the Study of Diabetes (EASD) have suggested creating treatment to be more or less strict depending on the mindsets of individuals, the risk of hypoglycemia, the length of the illness, lifespan, complications and resources. The development of a therapeutic plan that is specific to the patient is the basis of individualized diabetes care. Along with social, medical (including comorbidities), clinical and phenotypic factors, they also included biochemical, genetic and phenotypic elements (Williamset al., 2022).
Precision Medicine Foundations
A report by the US National Research Council that attempted to establish an innovative taxonomy for illness identification using a knowledge network is where the phrase “precision medicine” first gained notoriety (Ashley, 2016). Subgroups and categorisation have long been fundamental to the study of medicine. The ancient Greeks believed that certain factors, including gender, location, social status, humour’s, nutrition, trauma, beliefs and attitude, had an impact on both health (“a gift from the gods”) and disease (“divine punishment”) (Merino and Florez, 2018). Medical information, genomic information and environmental data are the three main types of data used in PM. On the other hand, the following is a short and straightforward explanation of how an IoT-based healthcare system functions. First, the patient’s body of interest is directly connected to the IoT health sensors and gadgets. Sensors gather a range of physiological information including signs of life (Afzalet al., 2020). Genome-guided medical treatment places an emphasis on a patient’s unique genomic characteristics to determine if they are likely to benefit from a particular treatment, avoid harsh adverse reactions from drugs that are unlikely to work and change prescription dosages to maximize their efficacy and safety. Drug reaction variable in humans is known to be significantly influenced by genetic variants (Tremblay and Hamet, 2013). The most popular genetic testing in the USA are prenatal screenings, which include sequencing paternal blood-derived neonatal fragments of DNA for genetic disorders. Another exciting new subject that is essential to modern genomics is pharmacogenomics, which optimizes drug response in relation to genetics (Maret al., 2019). These innovations will thus be a component of the omics pipelines, which also includes methods for supporting PM such as microbiomics, transcriptomics, interactomics, transcriptomics, proteomics and peptidomics. Numerous current studies have shown that such a PM strategy is both feasible and efficient. The integrated Private Omics Profile, an extensive individual omics characterization that combined an individual person’s genomic, transcriptomic, proteomic, metabolomic and autoantibody profiles over a 14-month period, revealed dynamic molecular and medical phenotypes including a risk for type 2 diabetes (Nice, 2018).
Genetics and Genomics in Diabetes
Biomedical research has a primary focus on identifying and characterizing the genetic variations that either cause or predispose to diabetes. A variety of monogenic and syndromic types of diabetes have been developed during the last fifteen years and the utilization of standard directional cloning and candidate gene techniques has been successful in generating increasingly thorough inventories of the genes that are causally linked in these developments (Owen and McCarthy, 2007). The potential of personalized medicine is being realized by scientific and technological advancements in genomics, which are transforming our approaches to genetic counselling and examination, specific therapy and cancer diagnosis and prevention (Weitzelet al., 2011). In the pathophysiology of T2D, concurrent or interdependent abnormalities of peripheral tissues’ (liver, muscle in the skeleton and adipose tissue) insulin sensitivity and pancreatic β-cells’ insulin secretion led to the decompensation of β-cell functioning and persistent hyperglycemia. T2D is recognized as a medically heterogeneous metabolic condition made up of numerous subtypes, including common polygenic forms of T2D in adults where genetic susceptibility strongly correlates with environmental factors and monogenic diabetes, which has greater penetrant genetic forms in young people (Vaxillaire and Froguel, 2008). We have made significant progress over the past ten years in understanding the genetic causes of diabetes. The clinical community has come to assume that with this advancement, our capacity to identify, classify and treat individuals with diabetes will change. This notion is understandable given the well-documented success in the quick transformation of gene finding efforts for monogenic variants of diabetes into modifications in clinical care pathways. Monogenic diabetes can account for up to 3% of cases in children, with alterations in the transcription factors gene HNF1A being the most frequent culprit. Rare penetrant HNF1A mutant carriers generally develop non-insulin-dependent diabetes before the age of 25, are thin and have a longstanding family history of the disease. Clinical evaluation typically indicates that these people are C-peptide positive but autoantibody negative (Gloyn and Drucker, 2018). In genomic epidemiology, study of associations looks at whether certain genetic variations (polymorphisms) in the studied population are linked to a particular phenotype. This trait might be quantitative (such as blood glucose levels) or qualitative (such as diabetes mellitus). The foundation of numerous approaches used in the investigation of diseases involving multiple, genetically and environmentally influenced components is the polygenic additive model. Genome-Wide Association Studies (GWAS) which examine hundreds of thousands of gene variants throughout the whole human genome to see if they are related with phenotype are a novel and effective method. The main issue with a GWAS looking at, say, 500,000 SNPs is that 25,000 false positive associations will be found if the standard significance level of p 0.05 is used.
Type 2 diabetes mellitus GWAS
The geneticist’s worst nightmare is Type 2 Diabetes Mellitus (T2DM). Yet, current GWAS developments revealed some unexpected findings that led to a significant advancement. Six GWAS found six additional gene regions in addition to the five already known gene regions, bringing the total number of proven areas to 11 (Kronenberg, 2008).
Biomarkers for Personalized Diabetes Care
“A characteristic that is objectively measured and evaluated as an indicator of normal biological processes, pathogenic processes, or pharmacological responses to a therapeutic intervention” is the definition of a biomarker. The decision-making process involving drug discovery, preclinical pharmacological and toxicological investigations, clinical trials and even after marketing investigations is significantly influenced by biomarkers. Knowledge gains in the fields of molecular biology, genomics, transcriptomics, lipid omics, glycomics and proteomics have resulted in the discovery of a number of biomarkers that may one day be used in therapeutic settings. The treatment should be based on clinical observations in addition to the study of the biomarkers and the biomarkers should not be misconstrued for clinical end points. Genetics, proteomics, metabolomics and imaging can all be used to predict biomarkers and some of these methods are already being used in clinical settings (Figure 1) (Pichuet al., 2017). Many biological indicators or biomarkers, including C-reactive protein, gamma-glutamyl transpeptidase, or adiponectin, have been linked to the likelihood of developing T2D, according to a substantial body of observational research conducted over the past ten years. Due to the chronic and variable character of diabetes, the utilization using biomarkers may aid in better defining diabetes risk and guiding medical judgment. The usefulness of a novel biomarker in this situation depends on whether it enhances prediction beyond straightforward clinical data. Yet, biomarkers can also be useful in identifying the underlying mechanisms that increase the risk of diabetes, which might afterwards help design new pharmacological targets for curative or preventive therapies (Abbasiet al., 2016).
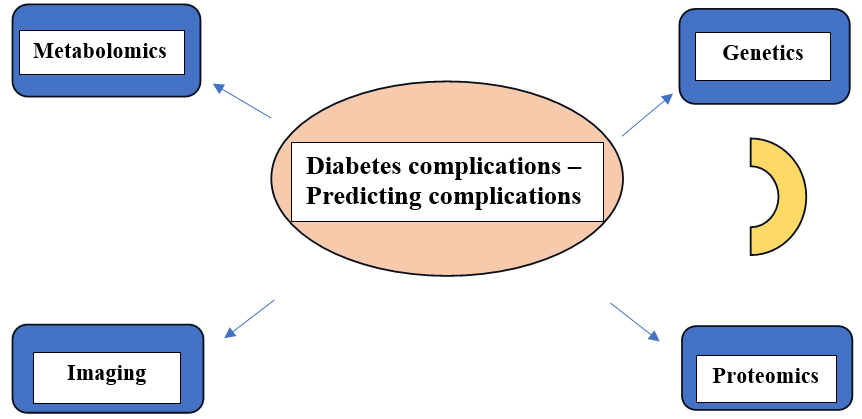
Figure 1:
Identification of biomarkers for diabetics and its complication using advanced techniques.
miRNAs as biomarkers in diabetic complications
A recently identified class of non-coding RNAs called miRNAs is essential for controlling the expression of genes. They alter the post-transcriptional pathways, which in turn suppress the expression of the target genes which play a variety of roles in cellular function. miRNAs play a variety of roles in numerous biological processes including division, proliferation, cell death and growth, which are all supported by a large body of research. miRNAs can be targeted for many diseases, including diabetes and its consequences, because they have unique properties that allow them to regulate important pathways in a variety of physiological and pathophysiological circumstances. Dysregulation of miRNAs has been linked to an increasing number of illnesses. miRNA expression changes that occur as diabetes progresses have been linked to a number of diabetic problems, including cardiomyopathy, nephropathy, retinopathy, neuropathy and several. New research has established a direct connection between changed miRNA expression and its effects on diabetic complications (Pichuet al., 2017).
Genetic Biomarkers
Studies of large cohorts have identified over 50 loci that influence the chance of developing type 1 diabetes and genetic indicators are used to determine type 1 diabetes propensity. But routine HLA typing is used to test for genetic risk for type 1 diabetes, similar to the way it is carried out for other autoimmune conditions. There is currently no widely acknowledged link with any risk variants despite estimates of the heritability of DR and proliferative DR being as high as 27 and 52%, respectively. Despite a sizable number of genes and genetic variations that have been demonstrated to be significantly linked with DR, none of these relationships have been repeated consistently across numerous populations (Tinget al., 2016).
Emerging biomarkers: omics
It has become possible to track the changes that take place when type 1 diabetes develops using a variety of omics technologies. Changes linked with the onset of type 1 diabetes can be identified early in the course of the disease, according to the study of long-term data from people at elevated risk for the condition and samples from properly matched controls. Additionally, recent research has shown that serum proteomes can be used to track the development of the disease in people with type 1 diabetes at several phases, including early childhood, seroconversion and diagnosis. When it comes to the pathophysiology of type 1 diabetes, metabolomic and lipidomic markers are likely to indicate alterations in immunological and metabolic state. They’ll probably produce a brand-new class of immunological indicators as well. Awareness on how surroundings and antibodies may influence one another may be gained from metabolomic investigations, which are also just beginning to take off. The recognition of relevant indicators, such as those for patient classification and inquiries for patients to gauge their reaction to therapy, may then result from tailored analysis of particular analytes (Mathieuet al., 2018). The accessibility of big data with the growth of technologies to mine these data are two significant factors in the recent explosive growth of precision medicine. Traditional medical data sources like patient history, physical exam and lab workup are thought to be more accurate when combined with deep phenotypic data mining, genomic analyses, epigenetic, metabolomic, proteomic and transcriptomic data to help individual patients receive precision medicine as showed in Figure 2 (Mohan and Radha, 2019).
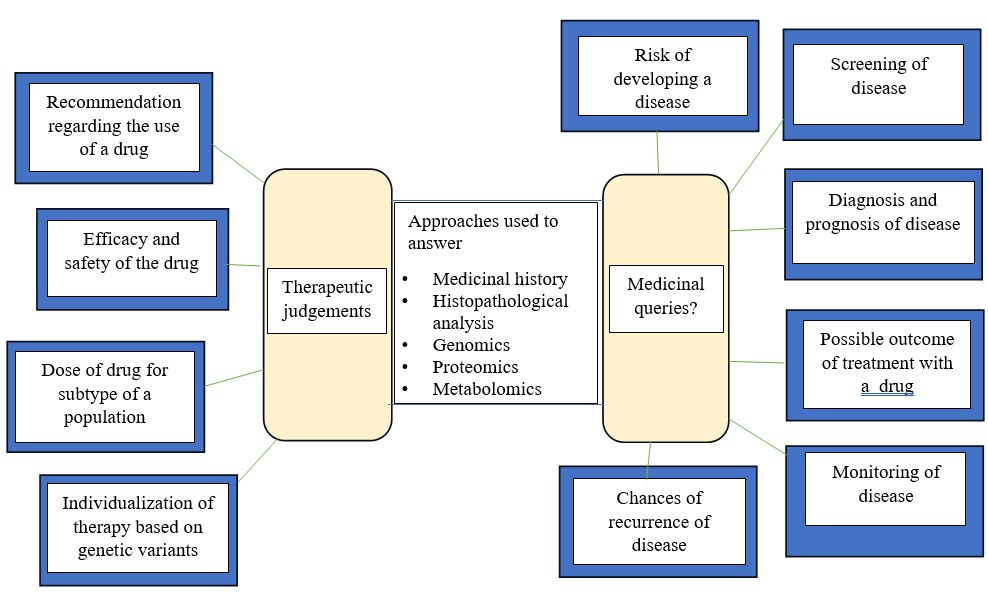
Figure 2:
For improved therapeutic judgments and individualization of therapy, medical queries and the use of omics and other techniques are also used.
Diabetes Subtyping: Moving Beyond Type 1 and Type 2
More accurate categorizations can be made using genotypic and phenotypic data, with the goal of illuminating the various biological pathways that contribute to the development of hyperglycemia in a particular individual. A precise medical approach to managing diabetes may be made possible by such patient stratification, which would highlight subgroups of clients who are: (1) most at threat for progression of the disease and/or (2) more likely to benefit from specific management regimens. While they were recognized as separate entities long before these words were developed, in the past, a large percentage (>95%) of people who developed hyperglycemia outside of pregnancies have been divided into two subtypes known as type 1 and type 2. Clinical traits have been used to categorize these subtypes, which have been revised throughout time to reflect new information, such as the 1970s discovery of autoantibodies against islet cells in the pancreas in type 1 diabetes. The error of the present medical classifications of diabetes has led to the development of a number of algorithms to more objectively divide the disease into subtype based on phenotypic characteristics. These algorithms take into account the presence or absence of self-antibodies along with unaltered β cell function in diabetes involving ketoacidosis (the “AB classification”), blood-based predictors of insulin secretion ability and diabetes-related insulin resistance, enormous scale study of networks of phenotypes from electronic health records and more. All of these methods have shown that there is variation within the various diabetes subtypes, but they only applied to a tiny portion of all diabetes cases or haven’t been widely duplicated (Deutschet al., 2022).
Tailoring Pharmacotherapy
The avoidance of DM, early identification and slowing the disease’s course are essential steps to take in order to prevent potentially fatal consequences. The use of patient-specific pharmacological methods to treat is encouraged by current guidelines, which emphasize the value of individualized care for individuals with DM. Personalized therapy for diabetes management also includes treating cardiovascular disease complications and educating patients on adopting a healthy lifestyle. The notion of patient-specific therapy can be expanded in light of genetic information in DM risk evaluation, pathogenesis, disposition and therapeutic efficacy (Elk and Iwuchukwu, 2017). Pharmacogenomics is an investigation of the genetic underpinnings of individual medication response variations. It is a young and difficult therapeutic discipline with little clinical value and application. There are a lot of factors that might affect a medicine’s efficacy, such as the environment, weight, age, gender and digestion. Patient’s privacy must be protected and everyone should be given the same chance to benefit from individualized therapy. Pharmacogenomics has the potential to significantly solve problems with medication safety and effectiveness. Pharmacogenomics’ long-term objective is to assist physicians in making diagnoses and prescribing medications and dosages depending on the genetic composition of the patient. Pharmacogenomics’ main goal is to investigate and compile all the genetic and epigenetic variations that influence medication response. For medications used for the avoidance and treatment of diseases like cancer, diabetes, mood disorders, vaccinations, anticonvulsant, anti-infective, cardiovascular and psychiatric treatments and also for various other therapies, studies relating to pharmacogenomics have been carried out. Several people pass away as a result of the drug’s side effects (Singh, 2020).
The Impact of Pharmacogenomics in Personalized
Personalized Nutritional Interventions
According to current nutritional suggestions aimed at enhancing the general quality of food are crucial for the avoidance and management of diabetes. These recommendations include eating a diet high in veggies, whole grains, fruits, nuts, legumes and marine while consuming fewer refined and highly processed foods. Particularly regarding the health benefits of dairy, meat and drinks, as well as dietary trends such as ketogenic eating habits, there is less agreement among experts. The disparity about the health benefits of particular nutrients, food items, or diets also stems from inter-individual variances. Previous research has demonstrated that there is a significant interindividual variation in how people react to the same meals due to variations in demographic, medical, genetic, gut microbiota and lifestyle factors (Singh, 2020).
Nutrigenomics investigates the interactions between genetics and foodstuffs or food bioactive and their impact on human health. It tries to determine the effects of various nutrients, especially micronutrients as well as macronutrients, on the genome. The term “Nutrigenomics” also refers to the study of how nutrients affect gene expression, transcriptional activity and the diverse responses of gene variations. The relationship between diet and the human body is extremely complex, encompassing the physiology of several organs as well as molecular mechanisms at every regulatory level, including genes, gene expression, proteins and metabolites. To investigate the intricate relationship between dietary phytochemical consumption and human health, metabolomics methods are applied. The term “nutrigenomics” also refers to the study of biological systems using functional genomic methods to understand how dietary components influence metabolic processes and homeostatic regulation. Human behaviour and metabolic functions are influenced by the regulation and gene expression brought on by eating. The power of precision nutrition to improve human health is great. Technologies used in nutrigenomics research include transcriptomics, proteomics and metabolomics (Farhudet al., 2010).
Wearable Devices and Continuous Monitoring
In the last 10 years, customers have spent a lot more time dealing with technology and machine learning. In four crucial areas of diabetes care, including computerized retinal testing, support for clinical decisions, predictive risk population stratification and self-management for patients’ tools, Artificial Intelligence (AI) is widely used. Artificial Intelligence (AI), a branch of computer science, works to create systems or procedures that can analyse data and control complexity in a range of applications. The application of AI is both desirable and practicable for effective data analysis and the development of tools and technology for treating diabetes. To produce safer technology utilizing AI, it is suggested that there should be safer designs, safe reservations and production safeguards with all hazards outlined for all potential technological systems. Wearables, cell phones and other devices have been made possible by technological advancements and they can help with ongoing symptom monitoring and illness status tracking. To treat diabetes effectively, doctors and other healthcare providers should let patients select AI-assisted care. The three main components of diabetes treatment that AI may influence and improve patients with the disease, medical professionals and healthcare systems (Ellahham, 2020). The current technological revolution includes consumer acceptance of wearable technologies. Wearable electronics, also known as wearables, are defined by Wright et al. as smart computers incorporated into a range of accessories, such as clothing, trendy accessories, smart watches and other common consumer goods. There are more and more ways than ever before that these advances in technology are being applied in healthcare. This is brought on by the numerous sensors that have been incorporated into these gadgets, including those that detect sound, images, human body gestures and light levels. The development and roll out of better chronic illness management services may be made possible by the fusion of cutting-edge technologies, such as sensor technologies, wearable technology and artificial intelligence approaches. The body of research on managing diabetes with various AI-based solutions is extensive and difficult to understand (Makroumet al., 2022).
Data Analytics and Machine Learning: Enhancing Precision
Several investigators are carrying out experiments for identifying illnesses using various algorithms for classifying artificial intelligence techniques like J48, SVM, Naive Bayes, Decision Tree, Decisions Table, etc., as showed in the Figure 3. Since research studies indicate that machine-learning algorithms perform better in diagnosing a variety of diseases. The power of information gathering and learning algorithms comes from their ability to handle enormous amounts of data, combine data from multiple sources and incorporate background knowledge into the research (Makroumet al., 2022).
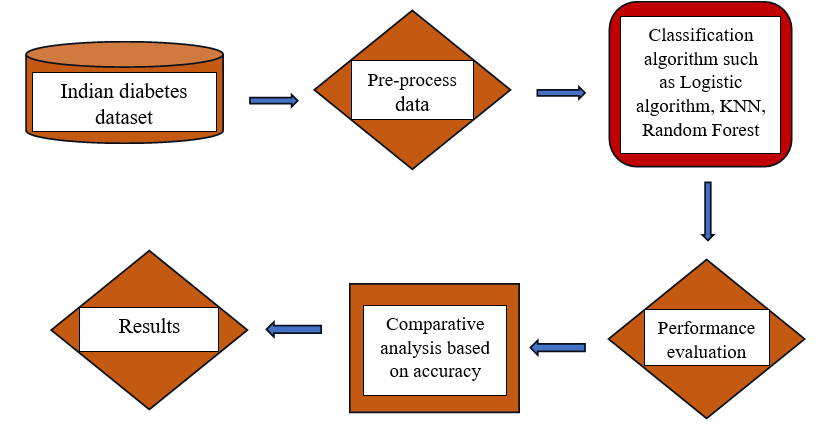
Figure 3:
Framework of Monitor Learning techniques.
Challenges and Ethical Considerations
The implementation of personalized medicine in diabetes management gives rise to ethical considerations concerning the acquisition of informed consent from patients. It is imperative for patients to possess a comprehensive understanding of the advantages, drawbacks and potential ramifications associated with the disclosure of their genetic and personal information for making personalized treatment decisions (Ellahham, 2020). The use of precision medicine in the management of diabetes raises ethical questions about how to obtain patients’ informed permission. In order to make informed decisions about their own care, patients must have a thorough awareness of the benefits, risks and other implications of disclosing their family history and personal information. In the area of personalized medicine, the gathering and storage of patient genetic and medical data may pose possible privacy problems. To protect confidential information from unauthorized access and breaches, it is essential to put into place strong data security measures and rigorously adhere to patient privacy requirements. Patients may raise worries about who will hold their data, who will use it for research and how it will be shared with outside parties (Sugandhet al., 2023; Golledgeet al., 2020). The collection and storage of patients’ genetic and health-related data in the field of personalized medicine may present potential privacy risks. It is imperative to implement robust data security measures and strictly adhere to patient privacy standards to safeguard sensitive information from unauthorized access and breaches. Patients may express concerns regarding the ownership of their data and the manner in which their information will be utilized in research or disclosed to third parties. Research, healthcare and regulatory science have entered a new frontier as a result of recent advancements in digital technology (such as sensors, software and algorithms) and their increasing pervasiveness in daily life (Coravoset al., 2019; Sim, 2019). Patient participation in research, the creation of new types of scientific data to support regulatory conclusions and the potential fulfilment of prior “bigdata” objectives in areas like post-approval surveillance of medical goods are all examples of these unique techniques. More generally, it’s possible that we’re only beginning to see a paradigm change in the divisions and connections between biomedical research, health care and regular activities. Technologies that will enable continuous tracking of health markers from diverse sources are being developed for the initial time in human history. This has the great potential to improve precision medicine at both population- and individual-levels. Additionally, this offers chances to improve healthcare delivery models for patients, providers and systems (Adamoet al., 2020). The sources, categories and amount of data that may be gathered on health are all changing quickly as a result of technological advancements. These data may contain important information about a person’s health condition, needs, illness load, relative value of outcomes and outcomes in a longitudinal picture of a person’s health and the efficacy of interventions or treatments. Such information and insights can then support a variety of stages in the life cycle of developing a medical device. As a result, these data have the potential to be a substantial and crucial source of knowledge that not only helps assess the effectiveness of medications but also increases their value by managing care pathways. However, the quality, quantity and type of data generated and continuously gathered in real-world scenarios (such as at-home monitoring, wearable technology and other IoT-connected devices) may differ significantly from those generated in conventional healthcare or controlled research settings (Beesonet al., 2020).
Clinical Implementation and Translational Research
Similar significant growth is being seen in the area of precision medicine. The National Research Council at first referred to its establishment of “a New Taxonomy of diseases in humans based on cellular biology” as precision medicine. Another way to put it is that precision medicine is a movement in health care that was sparked by information learned from arranging the human genome. Since then, the discipline has advanced to understand how the convergence of multiomic data with medical history, social and behavioural variables and environmental knowledge properly describes medical conditions, disease states and treatment alternatives for afflicted individuals. With the help of precise medicine, healthcare professionals now have the opportunity to collect and disseminate information that either supports or alters a clinical decision, changing from one that is based upon the evidence for the typical patient to a choice that is dependent on the individual’s specific features. It makes it simpler for physicians to give each patient tailored care. Early illness identification and the creation of individualized therapies are just a couple of the practical advantages that precision medicine advancements have to offer. Several data collecting and analytics technologies provide the ability of personalized medicine to tailor care (Johnsonet al., 2021).
Barack Obama, who was president at the time, unveiled a brand-new healthcare project in 2015 called precision medicine. The project uses genetic diversity to categorize people and target illnesses and preventative therapy. This effort uses the constantly expanding library of human genetic sequences in conjunction with improved computer intelligence to target oncology as its initial area of focus. This results in an educated basis for treatment choices. Since then, several organizations, like Intermountain Health Care (Levit et al., 2019), have adopted precision medicine. An integrative healthcare delivery organization called Intermountain Health Care provides patients with genetic testing as part of their program. Informed treatment plans may be given to patients thanks to the physicians’ prior understanding of the patient’s needs. The outcomes of this initiative have given patients better clinical benefits for comparable, if not less, money. Intermountain has changed its own policy in favor of this new endeavour due to the program’s results, putting greater faith in it as its success increases (Ni Kiet al., 2022).
Patient Empowerment and Shared Decision-Making
An individual gains more control over their choices and self-care practices via the process of empowerment. Having enough knowledge about diabetes is crucial for DM patients in order to improve patient empowerment. According to several researches, empowerment can improve psychological well-being, encourage behavioural changes, reduce HbA1c levels and increase understanding of health issues, as well as self-care and self-control. The self-determination method, which attempts to enhance a person’s ability for autonomous care decision-making and critical thought, mainly focuses on shared engagement, awareness-raising, the provision of required information, taking into consideration linguistic and cultural variations and open communication. The World Health Organization, also known as the WHO, says that treating chronic diseases and preserving or improving the individual’s quality of life depend on doing this. People with poorly managed DM may see an improvement in their quality of life through a patient-centered empowerment-based approach. According to Chan et al.’s study, those who participated in the person-centered empowerment-based program showed a decrease in blood sugar level of 0.476%, which is on the edge of becoming a clinically significant improvement in glycaemic control (Lambrinouet al., 2019). The ability of everyone to decide if there are hazards to their own health or well-being has long been acknowledged by biomedical ethics. Patient preferences are an individual’s assessment of the relative importance of things, such as health conditions, treatments, treatment outcomes, or other elements of health or medical care. The idea of patient preferences takes advantage of the requirement to adapt therapy and care to the unique personality and beliefs of the person. Patient safety is related to the ideas of patient preference and joint decision-making. These three ideas centre on obtaining the best outcomes for patients with the fewest harms. Shared decision-making and patient preferences give a greater emphasis on attaining desired results from the standpoint of patients, whereas research on patient safety places a greater emphasis on eliminating medical mistakes. A failure to attain an anticipated consequence in an anticipated order of operations that is not attributable to chance is referred to as an error, according to reason. According to the evidence, a lack of pertinent data, incorrect data interpretation and inefficient communication are some of the main causes of inaccuracy. Patient preferences are significant pieces of information for decision-making with unclear, value-sensitive outcomes and doctors’ failure to gather this information may compromise their ability to provide the optimal results (Ruland, 2004).
Future Directions
The delivery of healthcare services, notably in the area of diabetes management, has been profoundly changed by the use of telemedicine and remote patient surveillance, which have emerged as important tools in modern medicine. Telemedicine enables healthcare professionals to undertake remote evaluations, diagnoses and treatments with the aid of technology, providing personalised care and improving patient outcomes. By enabling people to take an active role in their own diabetes selfcare, the combination of remote patient surveillance and virtual consultations significantly improves patient engagement. Nevertheless, telemedicine offers considerable potential for managing diabetes, but in order to fully realize these advantages, it is imperative to recognize and address the difficulties it faces. By removing accessibility barriers and helping patients to get quick, convenient medical help, the deployment of telemedicine has significantly impacted the provision of tailored diabetic care. Healthcare professionals can remotely check blood sugar levels, modify treatment plans as needed and send prompt feedback thanks to telemedicine, which allows them to tailor interventions to each patient’s particular needs. Data privacy and security concerns are raised by the transfer and preservation of patient data on telemedicine platforms. To ensure the confidentiality and safeguarding of patient information, it is crucial to employ strong data protection mechanisms. To effectively use telemedicine tools and provide excellent virtual care services, healthcare providers must undergo in-depth training (Whiteet al., 2000). Due to the various laws and reimbursement practices that exist in various regions, telemedicine faces regulatory and reimbursement problems. To encourage widespread adoption of telemedicine services, it is essential to simplify the current rules and create fair reimbursement procedures (Tesfaye and Selvarajah, 2012; Golledgeet al., 2020).
CONCLUSION
In conclusion, the transition of precision medicine in the management of diabetes from theory to clinical application has enormous promise. Personalized techniques, cutting-edge technologies and the incorporation of genetic markers have made diagnosis and therapy more precise. Personalized treatment plans have been made possible by theoretical underpinnings, including developments in genomes, metabolomics and artificial intelligence. Clinicians may now customize therapies to match the particular needs of each patient because of the discovery of genetic variations, biomarkers and lifestyle variables. A major focus is on empowering patients via individualized therapies and lifestyle changes. But resolving difficulties with pricing and accessibility is essential for fair implementation. The use of precision medicine has an opportunity to transform diabetes care as research advances, lessening the financial burden of this chronic illness and enhancing patient outcomes. This development is a key step in providing people with diabetes with healthcare that is more effective and individualized.
Cite this article:
Pooja K N, Bharathi D R, Ahmed SS, Jayanth B M, Chandan K. Precision Medicine in Diabetes Management: From Theory to Clinical Practice. Int. J. Pharm. Investigation. 2025;15(2):10-8.
ABBREVIATIONS
DM | Diabetes mellitus |
---|---|
T2DM | Type 2 Diabetes mellitus |
GWAS | Genome Wide Association Studies |
AI | Artificial Intelligence |
SVM | Support Vector Machine |
References
- Abbasi A., Sahlqvist A.-S., Lotta L., Brosnan J. M., Vollenweider P., Giabbanelli P., Nunez D. J., Waterworth D., Scott R. A., Langenberg C., Wareham N. J., et al. (2016) A systematic review of biomarkers and risk of incident type 2 diabetes: An overview of epidemiological, prediction and aetiological research literature. PLOS One. Public Library of Science 11: Article e0163721 Google Scholar
- Abhari S., Niakan Kalhori S. R. N., Ebrahimi M., Hasannejadasl H., Garavand A.. (2019) Artificial intelligence applications in type 2 diabetes mellitus care: Focus on machine learning methods. Healthcare Informatics Research 25: 248-261 Google Scholar
- Adamo J. E., Bienvenu R. V., Dolz F., Liebman M., Nilsen W., Steele S. J., et al. (2020) Translation of Digital Health Technologies to Advance Precision Medicine: Informing Regulatory Science. Digital Biomarkers 4: 1-12 Google Scholar
- Afzal M., Riazul Islam S. M., Hussain M., Lee S.. (2020) Precision medicine informatics: Principles, prospects and challenges. IEEE Access 8: 13593-13612 Google Scholar
- Ashley E. A.. (2016) Towards precision medicine. Nature Reviews. Genetics. Nature Publishing Group 17: 507-522 Google Scholar
- Beeson J., Bergmeier W., Best S. M., Blazar B., Bluestone J., Boyman O., et al. (2020) Scientific Advisory Board Chief Scientific Advisors [Internet] Google Scholar
- Coravos A., Goldsack J. C., Karlin D. R., Nebeker C., Perakslis E., Zimmerman N., Erb M. K., et al. (2019) Digital medicine: A primer on measurement. Digital Biomarkers 3: 31-71 Google Scholar
- Deutsch A. J., Ahlqvist E., Udler M. S.. (2022) Phenotypic and genetic classification of diabetes. Diabetologia. Springer Science+Business Media Deutschland GmbH 65: 1758-1769 Google Scholar
- Elk N., Iwuchukwu O. F.. (2017) Using personalized medicine in the management of diabetes mellitus. Pharmacotherapy 37: 1131-1149 Google Scholar
- Ellahham S.. (2020) Artificial intelligence: The future for diabetes care. The American Journal of Medicine. Elsevier, Incorporated 133: 895-900 Google Scholar
- Farhud D., Zarif Yeganeh M., Zarif Yeganeh M.. (2010) Nutrigenomics and nutrigenetics. Iranian Journal of Public Health 39: 1-14 Google Scholar
- Gloyn A. L., Drucker D. J.. (2018) Precision medicine in the management of type 2 diabetes. The Lancet. Diabetes and Endocrinology. Lancet Publishing Group 6: 891-900 Google Scholar
- Golledge J., Fernando M., Lazzarini P., Najafi B., G Armstrong D. G.. (2020) The potential role of sensors, wearables and telehealth in the remote management of diabetes-related foot disease. Sensors. MDPI 20: Article 4527 Google Scholar
- Johnson K. B., Wei W.-Q., Weeraratne D., Frisse M. E., Misulis K., Rhee K., Zhao J., Snowdon J. L., et al. (2021) Precision medicine, AI and the future of personalized health care. Clinical and Translational Science. Blackwell Publishing Limited 14: 86-93 Google Scholar
- Kronenberg F.. (2008) Genome-wide association studies in aging-related processes such as diabetes mellitus, atherosclerosis and cancer. Experimental Gerontology 43: 39-43 Google Scholar
- Lambrinou E., Hansen T. B., Beulens J. W. J.. (2019) Lifestyle factors, self-management and patient empowerment in diabetes care. European Journal of Preventive Cardiology 26: 55-63 Google Scholar
- Makroum M. A., Adda M., Bouzouane A., Ibrahim H.. (2022) Machine learning and smart devices for diabetes management: Systematic review. Sensors. MDPI 22: Article 1843 Google Scholar
- Mar C., Sam J., Mcgahan C. E., Devries K., Coldman A. J., Mo M., et al. (2019) Clinical 376 The influence of breast density on breast cancer diagnosis: A study of participants in the BC Cancer Breast Screening Program, 385. Drug-induced psychosis and neurological effects following nitrous oxide misuse: A case report, 372 Editorials 375 President’s Comment Strength in numbers: The power of cooperation [Internet]. BC Medical Journal 61: 370 Google Scholar
- Mathieu C., Lahesmaa R., Bonifacio E., Achenbach P., Tree T.. (2018) Immunological biomarkers for the development and progression of type 1 diabetes. Diabetologia. Springer Verlag 61: 2252-2258 Google Scholar
- Merino J., Florez J. C.. (2018) Precision medicine in diabetes: An opportunity for clinical translation. Annals of the New York Academy of Sciences 1411: 140-152 Google Scholar
- Midyett L. K.. (2023) One size fits all versus individualized medicine in type 1 diabetes management. Diabetes Technology and Therapeutics 25: S42-S47 Google Scholar
- Mohan V., Radha V.. (2019) Precision diabetes is slowly becoming a reality. Medical Principles and Practice. S. Karger AG 28: 1-9 Google Scholar
- Ni Ki C., Hosseinian-Far A., Daneshkhah A., Salari N.. (2022) Topic modelling in precision medicine with its applications in personalized diabetes management. Expert Systems 39 Google Scholar
- Nice E. C.. (2018) Challenges for omics technologies in the implementation of personalized medicine. Expert Review of Precision Medicine and Drug Development. Taylor & Francis Limited 3: 229-231 Google Scholar
- Owen K. R., McCarthy M. I.. (2007) Genetics of type 2 diabetes. Current Opinion in Genetics and Development 17: 239-244 Google Scholar
- Pichu S., Patel B. M., Apparsundaram S., Goyal R. K.. (2017) Role of biomarkers in predicting diabetes complications with special reference to diabetic foot ulcers. Biomarkers in Medicine 11: 377-388 Google Scholar
- Ruland C. M.. (2004) Improving patient safety through informatics tools for shared decision making and risk communication. In International Journal of Medical Informatics 73: 551-557 Google Scholar
- Sim I.. (2019) Mobile devices and health. The New England Journal of Medicine 381: 956-968 Google Scholar
- Singh D. B.. (2020) In Advances in Biochemical Engineering/Biotechnology 171: 369-394 Google Scholar
- Sugandh F., Chandio M., Raveena F., Kumar L., Karishma F., Khuwaja S., Memon U. A., Bai K., Kashif M., Varrassi G., Khatri M., Kumar S., et al. (2023) Advances in the management of diabetes mellitus: A focus on personalized medicine. Cureus 15: Article e43697 Google Scholar
- Sushko K., Menezes H. T., Wang Q. R., Nerenberg K., Fitzpatrick-Lewis D., Sherifali D., et al. (2023) Patient-reported benefits and limitations of mobile health technologies for diabetes in pregnancy: A scoping review. Canadian Journal of Diabetes. Elsevier 47: 102-113 Google Scholar
- Tesfaye S., Selvarajah D.. (2012) Advances in the epidemiology, pathogenesis and management of diabetic peripheral neuropathy. Diabetes/Metabolism Research and Reviews 28: 8-14 Google Scholar
- Ting D. S. W., Tan K.-A., Phua V., Tan G. S. W., Wong C. W., Wong T. Y., et al. (2016) Biomarkers of diabetic retinopathy. Current Diabetes Reports 16: 125 Google Scholar
- Tremblay J., Hamet P.. (2013) Role of genomics on the path to personalized medicine. Metabolism: Clinical and Experimental 62: S2-S5 Google Scholar
- Vaxillaire M., Froguel P.. (2008) Monogenic diabetes in the young, pharmacogenetics and relevance to multifactorial forms of type 2 diabetes. Endocrine Reviews 29: 254-264 Google Scholar
- Weitzel J. N., Blazer K. R., MacDonald D. J., Culver J. O., Offit K.. (2011) Genetics, genomics and cancer risk assessment. CA A Cancer Journal for Clinicians, NA–NA Google Scholar
- White W. B., Prisant L. M., Wright J. T.. (2000) Management of patients with hypertension and diabetes mellitus: Advances in the evidence for intensive treatment. The American Journal of Medicine 108: 238-245 Google Scholar
- Williams D. M., Jones H., Stephens J. W.. (2022) Diabetes, Metabolic Syndrome and Obesity: Targets and Therapy 15: 281-295 Google Scholar