ABSTRACT
Background
Nebivolol hydrochloride (NBV) is a highly selective beta-adrenoceptor antagonist belonging to the third generation of beta-blockers. It is primarily utilized in the management of cardiovascular conditions. Since NBV is marketed in the tablet dosage form, ensuring its quality control and evaluation is important.
Objectives
Analytical Quality by Design (AQbD) is a novel tool for the optimization of chromatographic methods. The present study explores the AQbD approach for optimization of RP-HPLC method for analysis of Nebivolol (NBV).
Materials and Methods
In method development, Box-Behnken Design was used wherein mobile phase, wavelength and flow rate were considered as independent variables while retention time, peak area and tailing factor were responses. The optimized set of parameters was a mobile phase (MeOH:0.1% aqueous acetic acid in 71:29%v/v), flow rate of (0.8 mL/min) and wavelength (282 nm).
Results and Discussion
The method was found to be linear in a concentration range of 10 to 50 μg/mL with regression value (r2) of 0.999. The LOD and LOQ were obtained at concentration of 0.54 μg/mL and 1.64 μg/mL. The %RSD values for precision and robustness were found to be <2%. The % recovery was found to be 102.49% to 103.44% during accuracy study. Ruggedness of the method was confirmed by different analysts.
Conclusion
The optimized and validated RP-HPLC method, for NBV analysis, is robust and precise, making it suitable for routine use in pharmaceutical analysis.
INTRODUCTION
Nebivolol hydrochloride (NBV) is a highly selective beta-adrenoceptor antagonist of the third generation of beta-blockers. It possesses a unique property, activating 3-adrenergic receptors to increase nitric oxide release, thereby improving endothelial function, inducing vasodilation, increasing arterial compliance and decreasing peripheral vascular resistance. Nebivolol possesses the molecular formula C22H25F2NO4 and has a molecular weight of 405.4 g/mol. It is denoted chemically as 1-(6-fluorochroman-2-yl)-[2- (6-fluorochroman-2-yl)-2-hydroxyethyl) amino] ethanol (Figure 1). The therapeutic efficacy of drug depends on its quality. Thus, use of sensitive analytical method for the analysis of bulk drugs during its development is a prerequisite to build a quality into a product.1,2
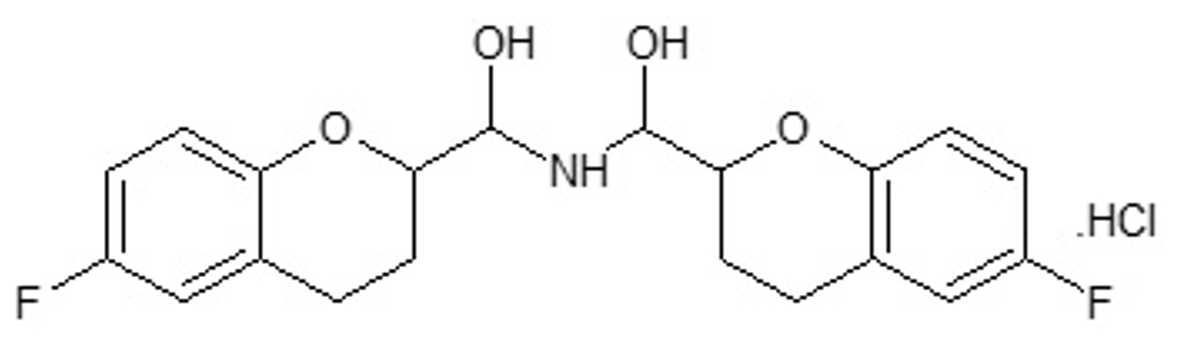
Figure 1:
Chemical structure of Nebivolol hydrochloride.
Analytical techniques, especially Liquid Chromatographic (LC) methods like High Performance Liquid Chromatography (HPLC) and Ultra Performance Liquid Chromatography (UPLC), are essential in drug product development and manufacturing. However, with the increasing complexity of drug products, method development becomes challenging. Robustness, the ability to consistently generate reliable results, is a crucial attribute. Conventional One-Factor-at-a-Time (OFAT) approaches often lead to non-optimized methods lacking insights into robustness, resulting in routine usage failures.3
In response to these challenges, the pharmaceutical industry is increasingly adopting the Quality by Design (QbD) approach in analytical methods. This approach emphasizes understanding the product and process from the outset and controlling specific Critical Quality Attributes (CQA) to ensure product quality. The implementation of Quality by Design is required by the European Medicines Agency (EMA) and the approval of new drug applications by the FDA is contingent upon the incorporation of analytical QbD principles.4,5
Box-Behnken Design (BBD) is indeed a valuable approach for optimizing chromatographic conditions and it typically employs a three-factor analysis integrated into a staged process. One of the key advantages of BBD is its ability to significantly reduce the total number of experimental runs required for optimization when compared to other techniques. This makes BBD a highly practical and efficient method for optimizing chromatographic parameters in analytical methods. The implementation of Quality by Design (QbD) in experimental processes is considered a novel and valuable technique. When applied in the development and validation of method using the HPLC analytical technique, optimized chromatographic condition under QbD principles can results in substantial savings in both cost and time. This is especially advantageous for routine analysis in laboratory settings, as it enhances the efficiency and reliability of results.6
The development of AQbD and QBD, in general, is supported by international standards, such as those from the International Conference of Harmonisation (ICH), like Q8 and the newly developed Q14 guideline. These standards provide a systematic and scientifically sound approach to product development, emphasizing the control of CQAs and robustness in analytical methods.4,6
To further enhance method development, the concept of Analytical Quality by Design (AQbD) has emerged. AQbD involves predetermining the Analytical Target Profile (ATP), establishing Critical Method Attributes (CMA) and their specifications and identifying Critical Method Parameters (CMPs). Design of Experiments (DoE) is integral to AQbD, employing statistical tools and mathematical modeling to explore factor effects and their interactions on specific responses (CMAs).7–9
The thorough literature survey revealed that, even though various analytical methods have been developed and validated for analysis of nebivolol but these methods lack the utilization of AQbD approach. In this context, in the present study AQbD approach is applied to develop a highly efficient RP-HPLC method for screening of NBV, in both bulk drugs and pharmaceutical products. The study employed an experimental design approach to depict the connection between various factors and responses, ensuring method robustness through validation studies with the aim to create a simple, rapid, sensitive, robust and reliable method.
MATERIALS AND METHODS
Reagents and Chemicals used
Nebivolol (NBV) sample used in this study was kindly provided as a gift by 4care Life Science PVT. Ltd. located in Bagdol, Gujrat. HPLC grade methanol was supplied by Merck India Limited and to ensure its purity, the solvent system was filtered through a 0.45 μm membrane filter (Nylon) purchased from Pall India Pvt. Ltd., Mumbai. Further, solvent system was degassed and sonicated using sonicator. The commercial tablets containing NBV were purchased from local pharmacies in Belagavi, Karnataka, India (Neirite Hydrochloride-5 mg Mfg. by Jaslin Pharma). These tablets were subjected to a comprehensive quality analysis utilizing a BBD-assisted RP-HPLC methodology.
Equipment and Chromatographic Parameters
HPLC: Agilent 1100 (Autosampler).
Software: Chemstation-10.01.
Stationary phase: Agilent C18 (250×4.6 mm i.d. 5μm).
Mobile phase: Methanol (MeOH): 0.1% aqueous acetic acid (71:29).
Detection Wavelength: 282 nm.
Flow rate: 0.8 mL/min.
Column Temperature: 25°C.
Sample size: 20 μL.
All the samples were filtered through 0.45 μm nylon filters, then utilized for solvent system preparation and degassed. To solubilize the drug and powdered drug sample in the solvent, a sonicator (MJL lab) was employed. Before use, all glassware, including pipettes, measuring cylinder and volumetric flask, was calibrated.
Preparation Mobile Phase
To prepare the mobile phase, 0.1% v/v aqueous acetic acid solution was prepared by diluting 1 mL of acetic acid solution with Milli-Q water upto 1000 mL. Appropriate quantity of resulting solution is further mixed with methanol in such way that final composition was consisted of MeOH:0.1% v/v aqueous acetic acid solution (71:29) ratio on v/v basis. Further the prepared mobile phase is subjected to filtration through 0.45 μm nylon membrane filter. The obtained solution is kept aside till its further use.
Preparation of standard stock solution
To prepare a stock solution of NBV, 10mg of NBV was dissolved in 10 mL of methanol and sonicated to ensure its dissolution. From this, 1 mL was transferred to a 10 mL volumetric flask and diluted to 10 mL using mobile phase as a diluent to get stock solution of 100 μg/mL. Further NBV working solution were prepared through serial dilutions using mobile phase as the diluent and then the final solution was filtered using a 0.45 μ syringe filter.
Preliminary Method Development
To determine the most appropriate stationary phase, initial experimental runs were performed to explore potential combinations and selection of column and solvent system suitability. Preliminary trials were carried out to optimize methodology in terms of cost-effectiveness, methanol is selected as the solvent for trial study. Additionally, aqueous solvent with different concentrations of acetic acid were selected to achieve effective separation under different pH conditions. After analyzing the outcomes of these initial trials, the Agilent C18 stationary phase used for further investigation.
AQbD enabled RP-HPLC method development
Quality by Design (QbD), is described as a systematic approach to development that commences with predetermined objectives and emphasizes the comprehension of both product and process based on robust scientific principles and effective risk management. QbD involves gaining a better scientific understanding of the essential factors and qualities of a product. It includes designing tests and controls based on our scientific knowledge during development and using the information gathered throughout the product’s life to continually enhance it. In this study, we have actively engaged with various aspects of AQbD (Analytical Quality by Design) and implemented them in practical methodologies. The process initiated with the identification of the Analytical Target Profile (ATP), followed by an examination of Critical Quality Attributes (CQAs) and Risk Assessment (RA) using the fishbone approach. Subsequently, our focus shifted towards the application of Design of Experiments (DOE), facilitating factor screening and the optimization of RP-HPLC method.
Analytical Target Profile (ATP) and Critical Quality Attributes (CQAs)
Formulating an Analytical Target Profile (ATP) is a crucial initial step in implementing the Quality by Design (QbD) approach. The ATP involves defining the specific quality attributes expected from an analytical method, providing a guiding framework for ensuring the method’s precision and accuracy. Factors like method application, instrument characteristics, drug properties and sample preparation methods are taken into consideration when establishing the ATP. Selecting Critical Quality Attributes (CQAs) is essential for achieving the desired ATP. CQAs represent measurable chromatographic attributes directly linked to the quality of the analytical method developed. Examples of CQAs include parameters such as Tailing factor (Tf), Retention time (Rt), Number of Theoretical Plates (NTPs), peak area and resolution between peaks.
Risk Assessment studies and Factor screening studies
The primary focus of risk assessment study is to determine the risks and probabilities of failure associated with method development. In the present study, Ishikawa Fish-Bone (IFB) diagram was developed to establish risk assessment, wherein examination of cause effect relationship between CPP and CMAs with Critical Analytical Attributes (CAAs) was studied. Further, optimization of chromatographic conditions was carried out by design expert software. The BBD was opted to optimize the CPPs or CMPs and interactions thereof on CQA. The three factors, three level configuration with reduced experimental runs utilized during optimization.10,11
Optimization study
Analytical Quality by Design was employed to assess the effect of independent variables on various responses. For the optimization, a BBD (Design expert 13 version software) was selected because it gives optimal results with a relatively few numbers of experimental trials. The three-factor, three-level BBD was applied to enhance an analytical method. The factors under scrutiny included the composition of mobile phases, specifically the percentage of methanol (A), flow rate in mL/min (B) and UV wavelength in nanometre (C) their individual and combined impact were assessed with respect to selected responses: Y1-Retention time (Rt) measured in min, Y2-Peak Area (PA) and Y3-tailing factor. Using software, a total seventeen experimental runs were conducted with various composition to identify the optimal combination (Tables 2 and 3). To ascertain the most suitable model, various kinetic models- including linear, 2nd order and quadratic models- were utilized to fit the experimental data. Polynomial equations, 3D plots and 2D contour plots were utilized to assess the variables’ effect on the responses. Statistical analyses, including “ANOVA and regression analysis”, were conducted to ascertain the model’s significance and identify the most suitable fit.12
Sl. No. | AQbD Parameters | Description |
---|---|---|
1 | Quality Target Product Profile | Flow rate, Mobile phase composition, Wavelength of detection, pH, Temperature, and Column attributes, Injection volume, Pressure, Buffer attributes. |
2 | Critical Quality Attributes | Retention time, Peak area, Theoretical plates, Peak purity, Tailing factor. |
3 | Process Parameters | Specificity and Selectivity, Linearity, range, Precision, Robustness, Ruggedness, Accuracy, LOD, LOQ. |
QTPP, CQAs and Process Parameters of Proposed RP-HPLC Method.
Factor | Levels used, actual (coded) | |
---|---|---|
Low (-1) | High (+1) | |
Independent variables | ||
A: Solvent conc. in mobile phase (% v/v) | 70 (-1) | 72 (+1) |
B: Flow rate (mL/min) | 0.7 (-1) | 0.9 (+1) |
C: Wavelength (nm) | 281 | 283 |
Dependent variables | ||
Y1: Retention time | Minimize | |
Y2: Peak area | In the range | |
Y3: Tailing factor | Minimize |
Variables and their levels used in Box-Behnken design.
Run No | Independent Variables | Dependent variables | ||||
---|---|---|---|---|---|---|
A | B | C | RT | PA | TF | |
1 | 71 | 0.8 | 282 | 3.612 | 546.621 | 0.49 |
2 | 71 | 0.8 | 282 | 3.619 | 545.124 | 0.44 |
3 | 70 | 0.7 | 282 | 4.194 | 622.816 | 0.42 |
4 | 71 | 0.7 | 281 | 4.187 | 616.703 | 0.48 |
5 | 70 | 0.8 | 281 | 3.703 | 539.244 | 0.44 |
6 | 71 | 0.8 | 282 | 3.55 | 548.039 | 0.47 |
7 | 72 | 0.8 | 281 | 3.592 | 541.349 | 0.57 |
8 | 71 | 0.9 | 281 | 3.225 | 478.997 | 0.5 |
9 | 71 | 0.8 | 282 | 4.184 | 545.128 | 0.45 |
10 | 71 | 0.8 | 282 | 4.19 | 546.874 | 0.44 |
11 | 70 | 0.8 | 283 | 3.743 | 550.477 | 0.45 |
12 | 72 | 0.9 | 282 | 3.112 | 488.084 | 0.54 |
13 | 72 | 0.7 | 282 | 4.022 | 630.453 | 0.51 |
14 | 71 | 0.7 | 283 | 4.184 | 631.114 | 0.46 |
15 | 71 | 0.9 | 283 | 3.25 | 490.101 | 0.5 |
16 | 70 | 0.9 | 282 | 3.401 | 488.354 | 0.46 |
17 | 72 | 0.8 | 283 | 3.542 | 552.433 | 0.52 |
Box-Behnken Design (BBD) based independent variable and their effect on dependent variables.
The “Analysis of Variance” (ANOVA) F test was utilized to select best fit 2nd order quadratic polynomial equation (Eq. 1) based on its level of significance
Where Y refers to response selected; while β0 indicates intercept; β1-β33 denote “regression coefficients”. The factors under investigation are denoted by A, B and C.
The Model, developed through the design, underwent evaluation and comparison based on several parameters. These parameters encompassed the “correlation coefficient, adjusted correlation coefficient, predicted correlation and adequate precision”. To gain insights from the model involved conducting response surface analysis, which included generation of “2D-contour and 3D-response surface plots”. Through the utilization of a numerical optimization method, the model predicted the optimal analytical condition. These optimized conditions were subsequently identified within the designated “Analytical Design Space”. This was visualized as an “overlay plot” that incorporated a flag consisting of all relevant parameters.12,13
Method validation
ICH Q2 (R1) guidelines were used to validate the optimized RP-HPLC method. Various parameters studied were linearity range, system suitability, LOD and LOQ limits, interday and intraday precision, accuracy, ruggedness and robustness. Further the optimized method was checked for its efficacy in assessment of quality of marketed NBV tablets.14
System Suitability and Specificity
The suitability of the system was assessed by studying various responses viz. Retention time (Rt), Peak Area (PA) and Tailing factor (Tf). These measurements were carried out in three replicates. Further, the interference of the mobile phase in the detection of NBV was studied by specificity study.
Linearity and Sensitivity analysis
Aliquots of stock solution were diluted serially in order to get the NBV solution of different concentration viz. 10-50 μg/ mL. These solutions were then injected into system and peak area of resulting chromatograms were determined. Further, the linearity of the method was determined by plotting the graph of concentration vs peak area along X and Y axis respectively. The regression coefficient (R2) value is indicative of linearity of the method. Further LOD and LOQ were determined by the following formulae
Precision
Precision of the method was established by observing the closeness of results obtained by injecting solutions of same concentration in triplicate. The precision was determined both interday (within a day) and intraday (different days). Based on the results obtained % RSD value were calculated.
Accuracy study
The recovery method was used to determine the accuracy of the proposed method. The % recovery of the drug after spiking 10 μg/ mL solution at 80%, 100% and 120% of its concentration level was determined by using the following formula
Robustness
Few deliberate changes in the optimized analytical parameters were carried out to determine the robustness of the method. The parameters changed were solvent system composition, wavelength and flow rate.
Ruggedness
Analysis of the NBV solution was carried out by two different analysts using optimized method in order to determine the ruggedness of the method. The ruggedness of the method was determined by calculating the % RSD of the obtained results.
Assay of Marked tablets
Weighed and powdered 20 NBV tablets (Nebicia Hydrochloride-5 mg Mfg. by Daxia Healthcare) into a fine powder. The powder sample equivalent to 10 mg of NBV was transferred to volumetric flask of 10 mL capacity. The powder sample is diluted with 2 mL solvent system and further sonication was carried out 15 min in order to get the sample completely solubilize. The volume was made up to mark by solvent. Filtration of the resulting solution was carried out. The obtained solution was further serially diluted to get the final solution of 30 μg/mL. The analysis of this sample was carried out and the % assay of the tablet was determined.
RESULTS
Optimization of Chromatographic Condition
Optimization of RP-HPLC method for the analysis of NBV was achieved by implementing AQbD. Initially preliminary experiments were carried out wherein various mobile phases were tried in order to get well resolved, sharp peak (mobile phase was varied by using different combinations of the solvents like methanol, water, acetic acid and phosphate buffers with different flow rate). Based on the results obtained it was found that mobile phase consisting of MeOH:0.1% aqueous acetic acid in 71:29 ratio was giving optimal sharp peak at 4.19 min with a flow rate of 0.8 mL/min and at 282 nm. All other compositions failed to give sharp peak. Further optimization with respect to wavelength, mobile phase and flow rate was carried out by utilizing BBD approach.
Risk Assessment and Screening Studies10
As per the discussion in the methodology study, the findings of analysis of ATP, CQAs and process parameters are detailed in Table 1. Ishikawa Fish-Bone diagram (Figure 2) depicting the association of different inputs of process parameters and analytical attributes was explored. The probable risk factors related to the solvent system were method of extraction of sample, extraction time, choice of solvents and mobile phase composition while instrument-related parameters were chromatographic mode, flow rate, injection volume and wavelength of detection. The risk factors viz flow rate, mobile phase composition and wavelength of detection are explored. Further for optimization by applying suitable experimental design.
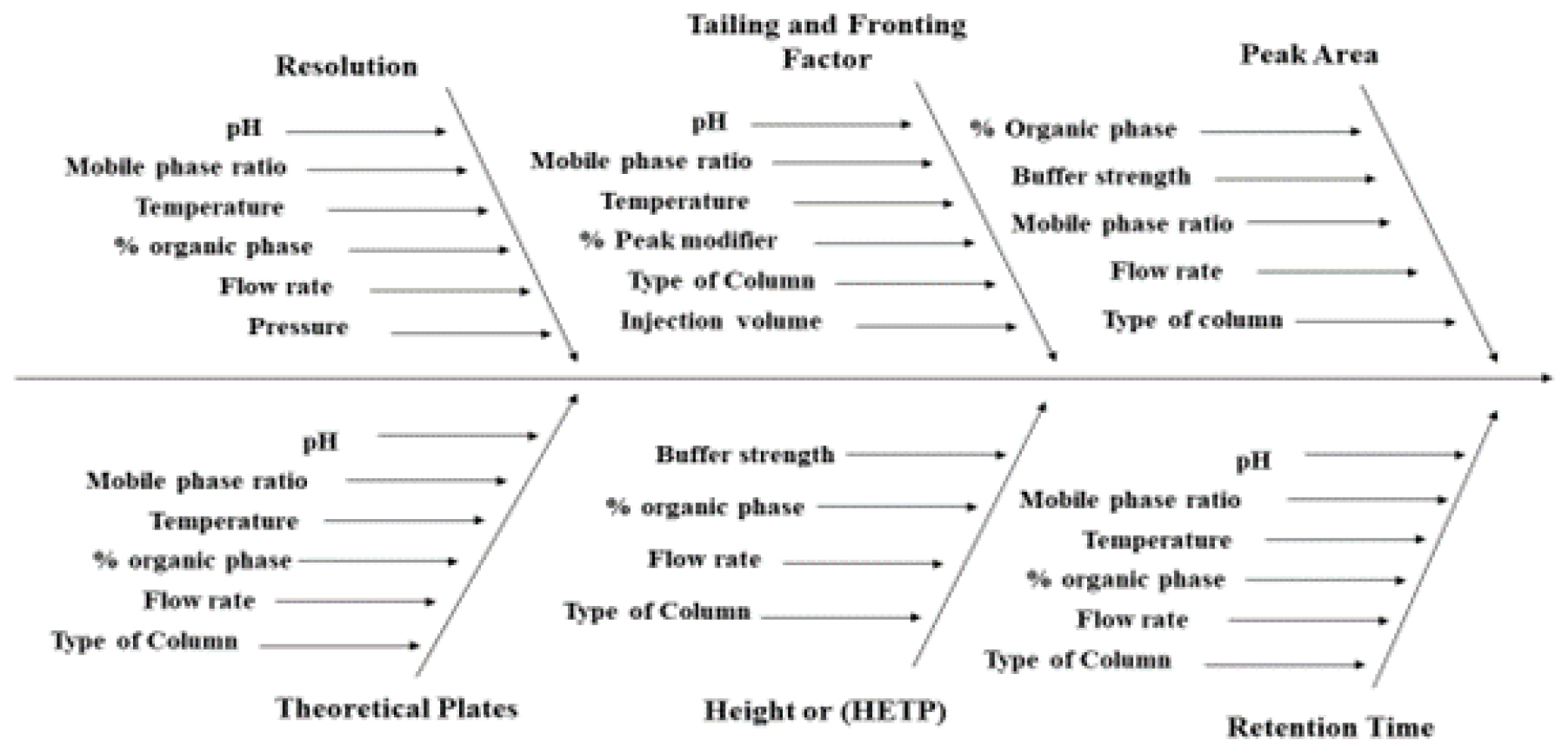
Figure 2:
Ishikawa fishbone diagram.
Method Optimization
The DoE software was employed to optimize analytical RP-HPLC parameters in order to get the best condition for estimating NBV. The impact of independent variables such as methanol (70 to 72%v/v), flow rate (0.7 to 0.9 mL/min) and wavelength (281 to 283 nm), on dependent variables such as Rt, PA and Tf, was estimated. The DoE software, a total of 17 experimental trial runs were obtained, facilitating the identification of the optimum chromatographic separation for NBV (Tables 2 and 3). The central composition point was defined by the variables: mobile phase ratio (MeOH:0.1% aqueous acetic acid 71:29% v/v), flow rate (0.8 mL/min) and wavelength (282nm), were identified for the design space selection. The effect of these variables on the responses were evaluated to establish the design space. Response data were fitted into various polynomial models, such as linear, second order and quadratic. The quadratic model emerged as the best fit, as evidenced by its high regression coefficient (R2=0.9999), significantly surpassing other models (p<0.0001). ANOVA tests were conducted for each model and parameters such as “sum of squares, F value, mean square and p value” for each response were determined. The results of the same are as depicted in Tables 4 and 5. The 3D-response surface plots and 2D-contour plots of each response, demonstrating the influence of many factors (integration effect) simultaneously on a response are shown in (Figures 3–8).
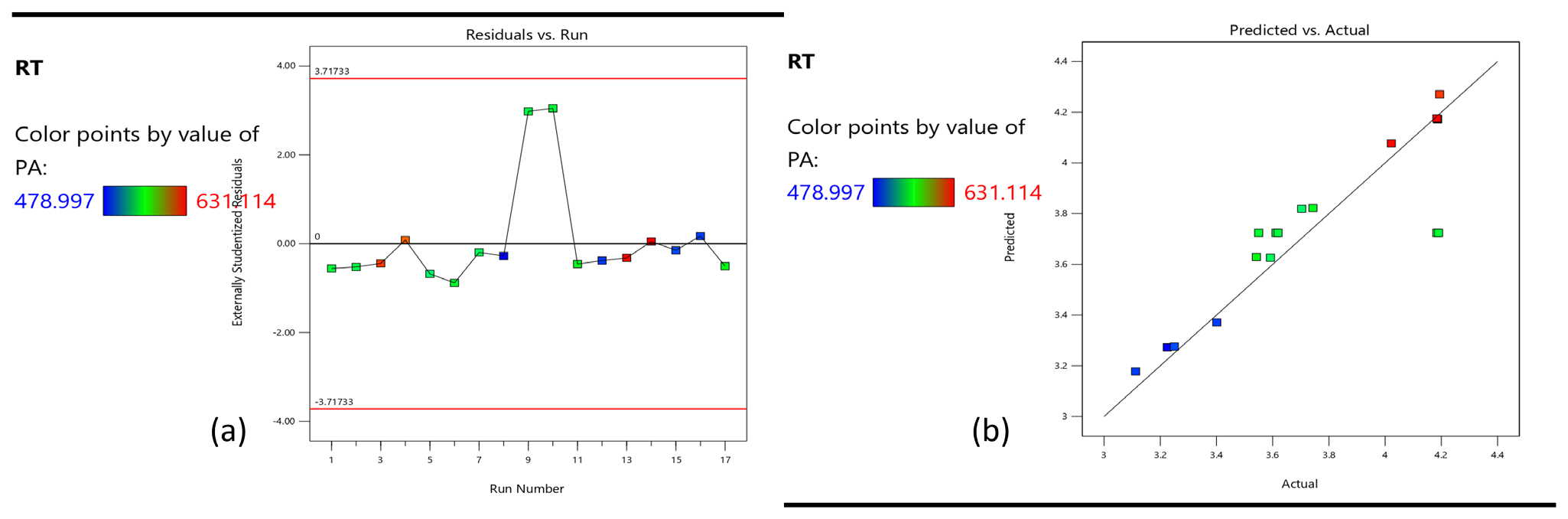
Figure 3:
Plot (a) showing true versus predicted values, with residual plot (b) for retention time.
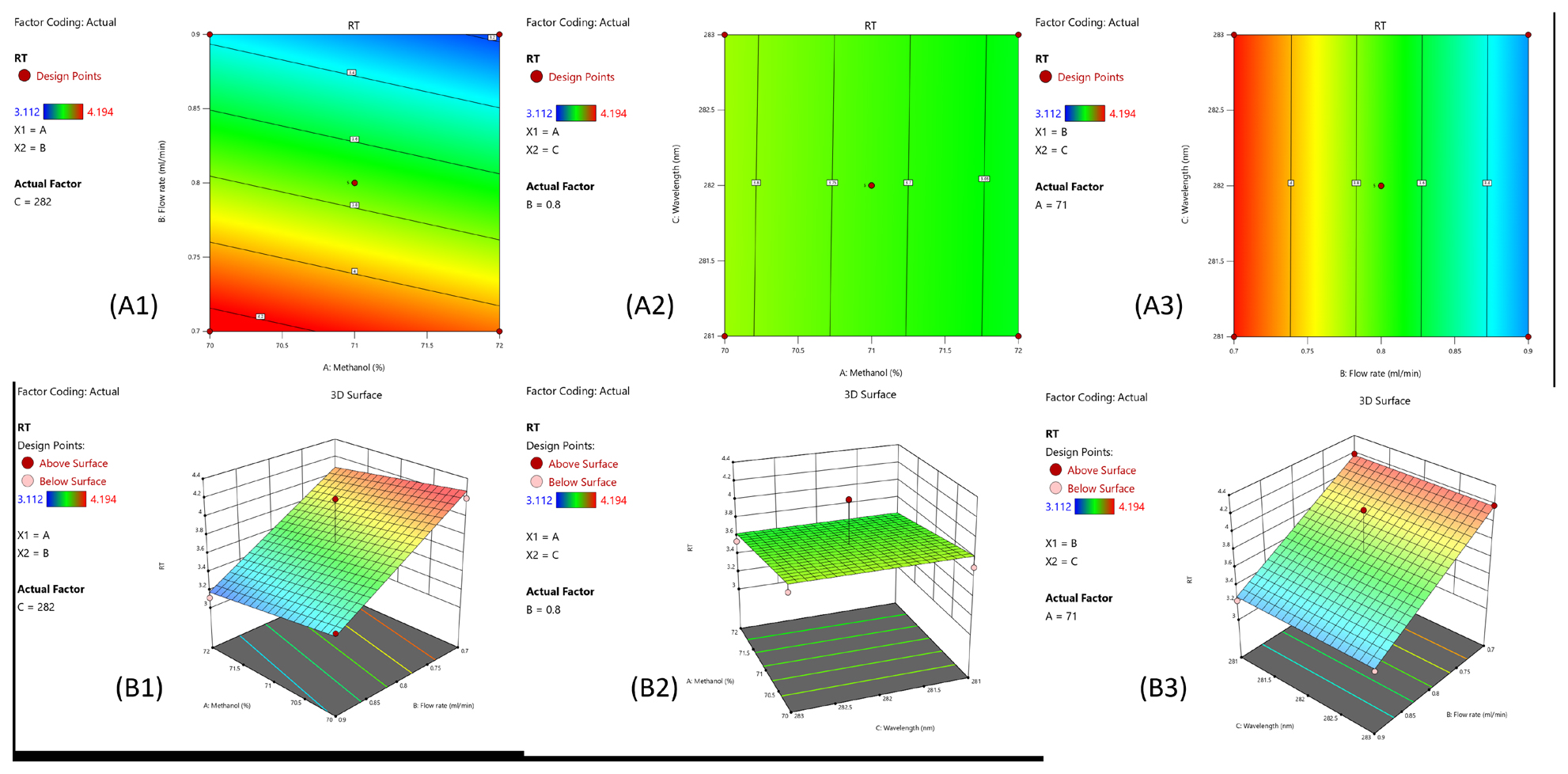
Figure 4:
Relation plot (A1-A3 and B1-B3) 2-D counter plots and 3-D response surface plots showing the influence of CMP’s i.e., (a) Solvent concentration in mobile phase (% v/v), (b) Flow rate (mL/min), (c)Wavelength (nm) on retention time (RT) as a CAA
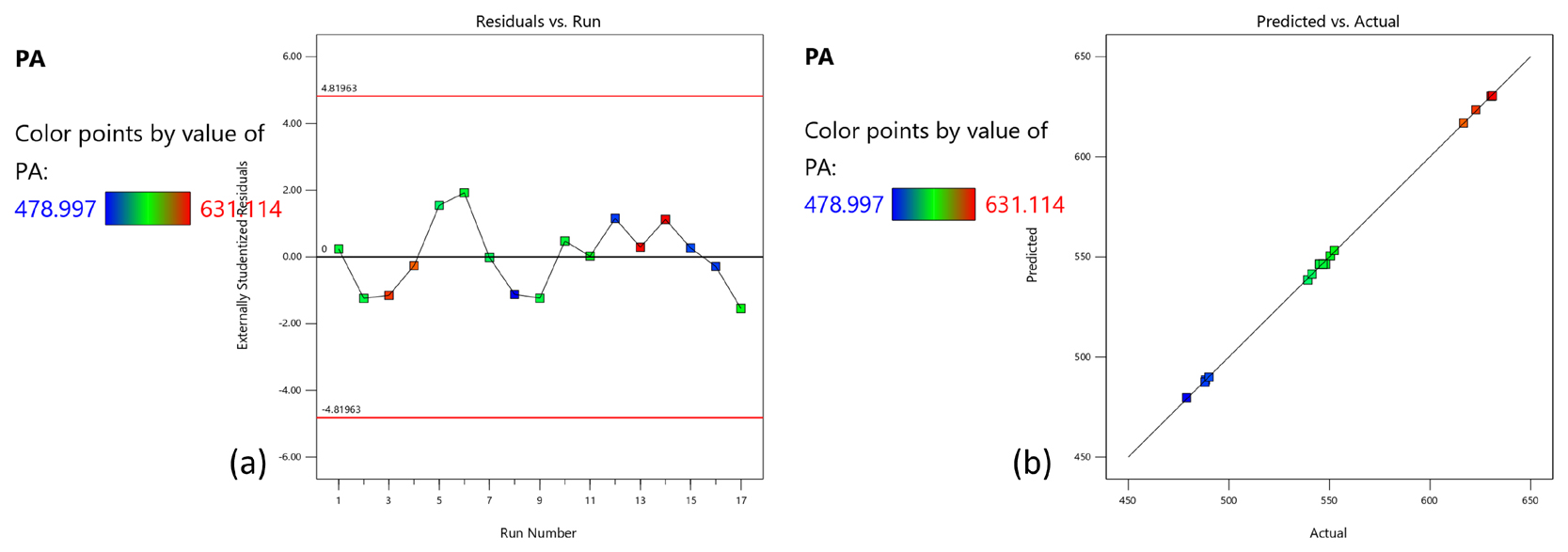
Figure 5:
Plot (a) showing true versus predicted values, with residual plot (b) for peak area.
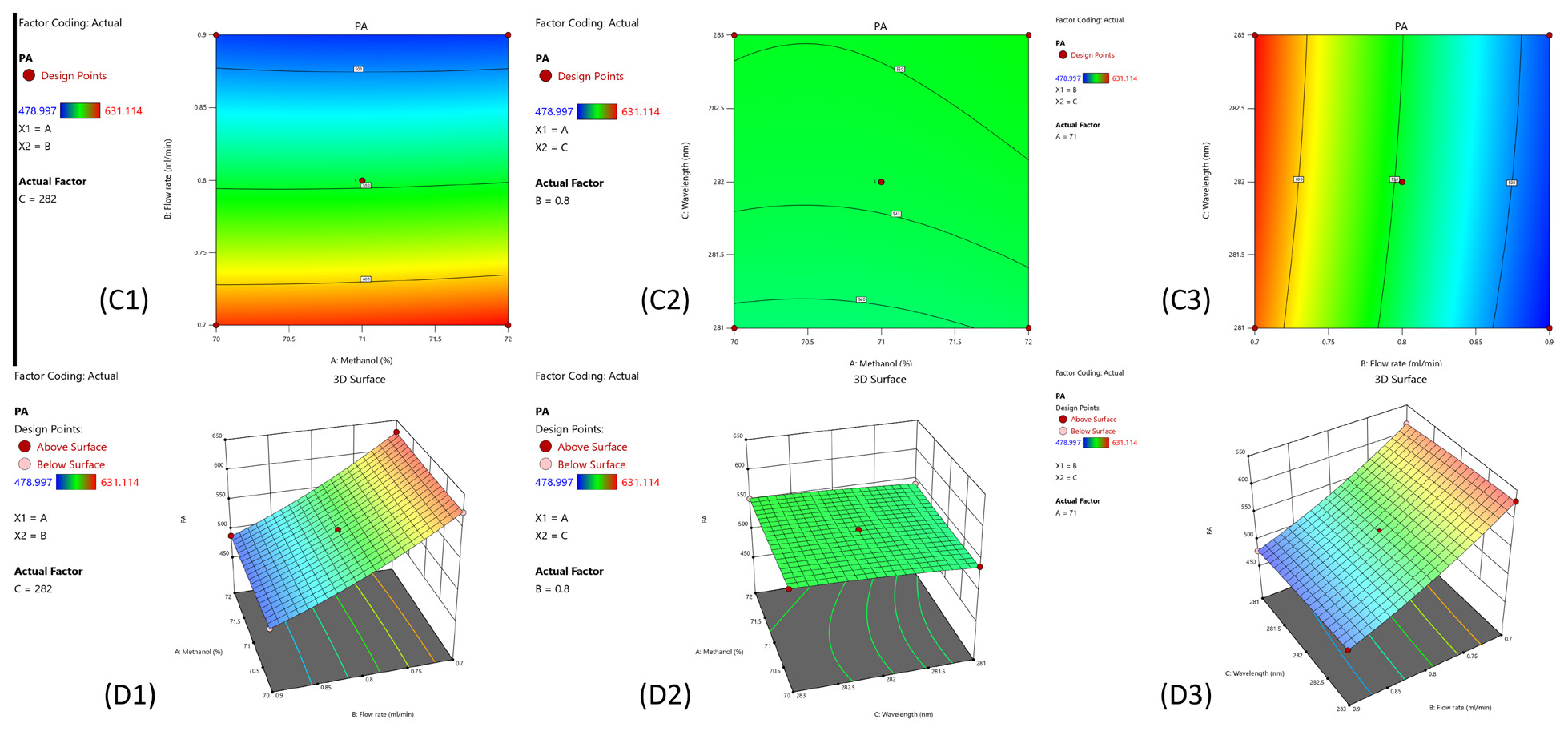
Figure 6:
Relation plot (C1-C3 and D1-D3) 2-D counterplots and 3-D response surface plots showing the influence of CMP’s i.e., (a) Solvent concentration in the mobile phase (% v/v), (b) Flow rate (mL/min), (c) Wavelength (nm) on Peak Area (PA) as a CAA.
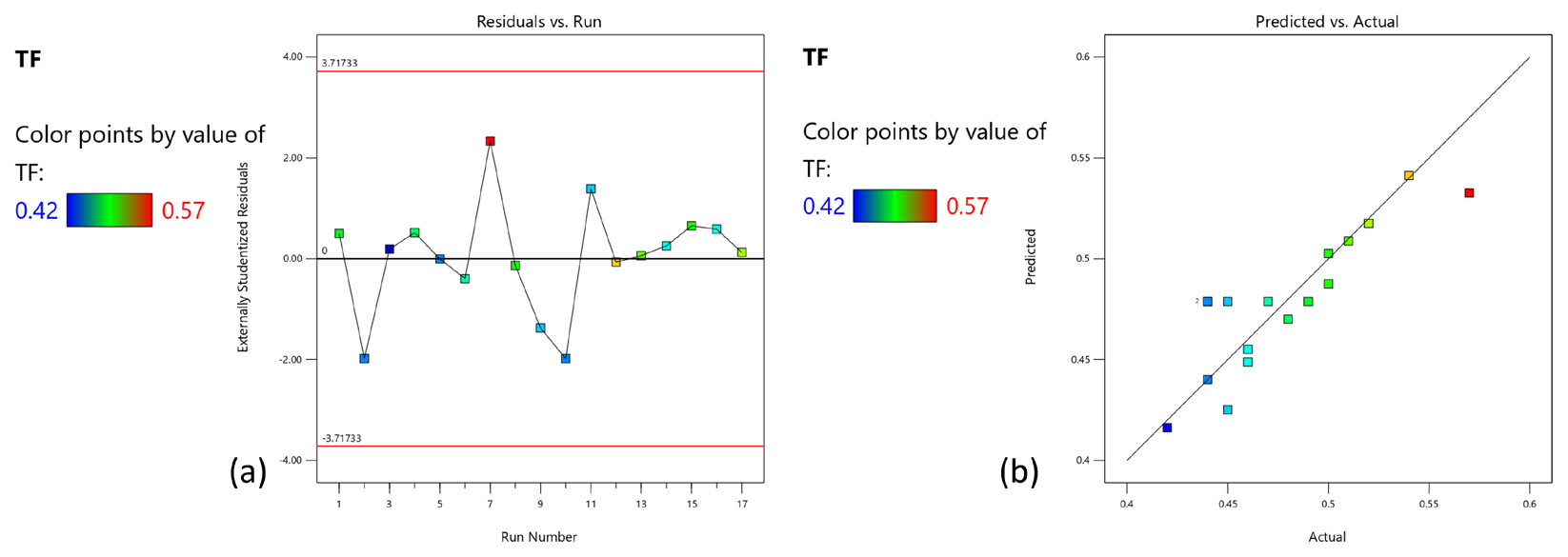
Figure 7:
Plot (a) showing true versus predicted values, with residual plot (b) for tailing factor.
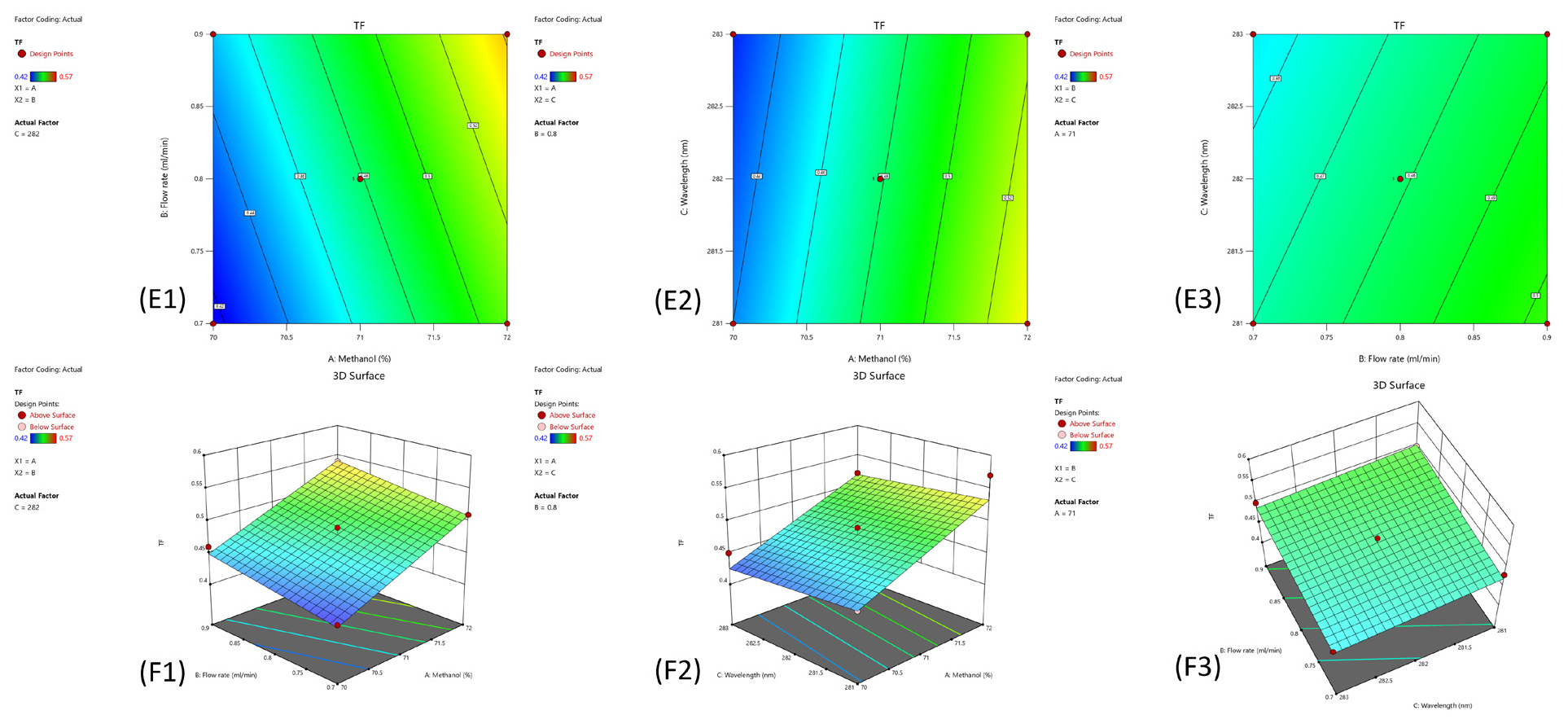
Figure 8:
Relation plot (E1-E3 and F1-F3) 2-D counterplots and 3-D response surface plots showing the influence of CMP’s i.e., (a) Solvent concentration in the mobile phase (% v/v), (b) Flow rate (mL/min), (c) Wavelength (nm) on the Tailing Factor (TF) as a CAA.
Models | SD | R2 | Adjusted R2 | Predicted R2 | PRESS | Adequate Precision | Remark |
---|---|---|---|---|---|---|---|
Retention Time (RT) | |||||||
Linear | 0.2016 | 0.7622 | 0.7074 | 0.7117 | 0.6407 | 11.1769 | Suggested |
2FI | 0.2286 | 0.7648 | 0.6236 | 0.6618 | 0.7514 | ||
Quadratic | 0.2487 | 0.8052 | 0.5547 | 0.6465 | 0.7856 | ||
Peak Area (PA) | |||||||
Linear | 5.86 | 0.9887 | 0.9860 | 0.9787 | 836.32 | 170.7498 | |
2FI | 6.54 | 0.9891 | 0.9826 | 0.9544 | 1793.27 | ||
Quadratic | 1.15 | 0.9998 | 0.9995 | 0.9985 | 59.13 | Suggested | |
Tailing Factor (TF) | |||||||
Linear | 0.0224 | 0.7516 | 0.6943 | 0.6292 | 0.0097 | 11.5231 | Suggested |
2FI | 0.0234 | 0.7908 | 0.6653 | 0.5451 | 0.0119 | ||
Quadratic 0.0171 | 0.9215 | 0.8206 | 0.7808 | 0.0057 |
Regression analysis for responses Retention time; Peak area, Tailing factor for fitting to different polynomial models.
Results (ANOVA) | Rt | PA | Tf |
---|---|---|---|
Regression | |||
Sum of squares | 1.69 | 39318.28 | 0.0197 |
Degrees of freedom (df) | 3 | 9 | 3 |
Mean Square | 0.5646 | 4368.70 | 0.0066 |
F-value | 13.89 | 3292.75 | 13.11 |
p-value | 0.0002 | <0.0001 | 0.0003 |
Lack of fit tests | |||
Sum of squares | 0.1030 | 3.09 | 0.0046 |
Degrees of freedom (df) | 9 | 3 | 9 |
Mean Square | 0.0114 | 1.03 | 0.0005 |
F-value | 0.1076 | 0.6650 | 1.09 |
p-value | 0.9971 | 0.6159 | 0.5046 |
Residual | |||
Sum of squares | 0.5284 | 9.29 | 0.0065 |
Degrees of freedom (df) | 13 | 7 | 13 |
Mean Square | 0.0406 | 1.33 | 0.0005 |
Analysis of Variance (ANOVA) for the responses (Y1: Retention time; Y2: Peak area, Y3: Tailing factor)
Effect of independent factors on retention time (Y1)
To depict the effect of variables under control on retention time, expressions like 3D response, counter plot and model equation were utilized. The effect of independent variables on retention time is as shown in Figures 3 and 4 (A1-A3 and B1-B3). When the data obtained after trial batches as per 33 factorial design is treated with software analytics, it was found that interaction terms have an insignificant effect on retention time. Thus, the linear equation obtained for Y1 (retention time) is given as follows
The term, B were found to be significant as the p-value for corresponding factors was found to be less than 0.05. Further, the significance of the model was determined by the model F-value which was 13.89. This witnessed that model is significant with a 99.98% chance of F-value being due to signal (i.e. noise<0.02%). The closeness between predicted (0.7117) and adjusted (0.7074) R2 was observed. The sufficiency of the signal is highlighted by an adequate precession value of 11.1769. The results of the same are depicted in Tables 4 and 5. The composition of mobile phase (A) and flow rate (B) have a “negative effect” on retention time, while wavelength (C) has a “positive effect” on retention time. The flow rate has more dominant effect on retention time as compared to the other two independent variables.
Effect of independent factors on peak area (Y2)
To depict the effect of variables under control on retention time, expressions like 3D response, counter plot and model equation were utilized. The effect of independent variables on peak area is as shown in Figures 5 and 6 (C1-C3 and D1-D3). When the data obtained after trial batches as per 33 factorial design is treated with software analytics, it was found that, both individuals as well as interaction terms have insignificant effect on peak area. Thus, the 2nd order quadratic equation obtained for Y1 (peak area) is expressed as given below
The term, A, B, C, AB, A2, B2 and C2 were found to be significant as the P-values for corresponding factors were found to be less than 0.05. Further, the significance of the model was determined by the model F-value which was 3292.75. This witnessed that the model is significant with a 99.99% chance of F-value being due to signal (i.e. noise<0.01%). The closeness between predicted (0.9985) and adjusted (0.9998) R2 was observed. The sufficiency of signal is highlighted by adequate precession value of 170.7498. The results of the same are as depicted in Tables 4 and 5. The composition of mobile phase (A) and wavelength (C) have “positive effect” on peak area, while flow rate (B) has “negative effect” on peak area.
The impact of the flow rate on peak area has more pronounced compared to the other two independent variables.
Effect of independent factors on tailing factor (Y3)
To depict the effect of variables under control on tailing factor, expressions like 3D response, counter plot and model equation were utilized. The effect of independent variables on the tailing factor is as shown in Figures 7 and 8 (E1-E3 and F1-F3). When the data obtained after trial batches as per 33 factorial design is treated with software analytics, it was found that interaction terms have an insignificant effect on retention time. Thus, the linear equation obtained for Y3 (tailing factor) is given as follows
The term, A was found to be significant as the P-values for corresponding factors were found to be less than 0.05. Further, the significance of the model was determined by the model F-value which was 13.11. This witnessed that the model is significant with a 99.97% chance of F-value being due to signal (i.e. noise<0.03%). The closeness between predicted (0.0.6292) and adjusted (0.6943) R2 was observed. The sufficiency of the signal is highlighted by an adequate precession value of 11.5231. The results of the same are depicted in Tables 4 and 5. The composition of mobile phase (A) and flow rate (B) have “positive effect” on tailing factor, while wavelength (C) has “negative effect” on tailing factor. The mobile phase has more dominant effect on tailing factor as compared to other two independent variables.
Optimization (Graphical and numerical)
Figure 9(a) shows the overlay plot depicting the relationship between independent variables and responses for graphical optimization, whereas Figure 9(b) depict the results of the numerically optimized method, having the highest desirability of 0.940.
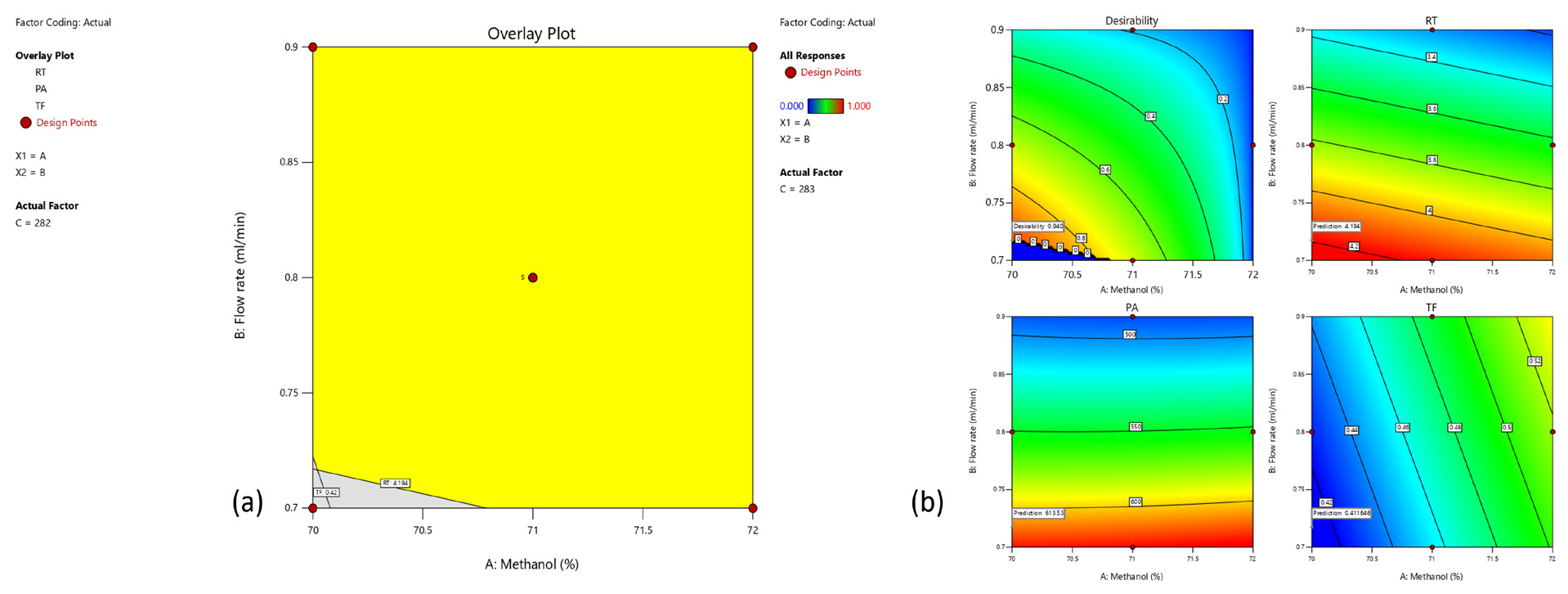
Figure 9:
a. Overlay plot showing the optimized chromatographic conditions and their predicted responses, b. Graphs showing numerical optimization with the highest desirability.
Method validation
Validation of an analytical method is an important step before its implementation in routine qualitative and quantitative analyses.
System Suitability and Specificity
The developed analytical method was assessed for system suitability, focussing on critical attributes such as chromatogram “retention time, peak area and tailing factor”. The results showed no significant difference, (%RSD<0.5) when it is studied with three replicates of NBV having a concentration of 30 μg/mL. The method specificity was assessed by analyzing both blank and spiked quality control samples which revealed that there is no interference in either case. This confirmed the suitability and specificity of the method for quantifying NBV in the formulation. Figure 10(a) presented an HPLC chromatogram of standard NBV.
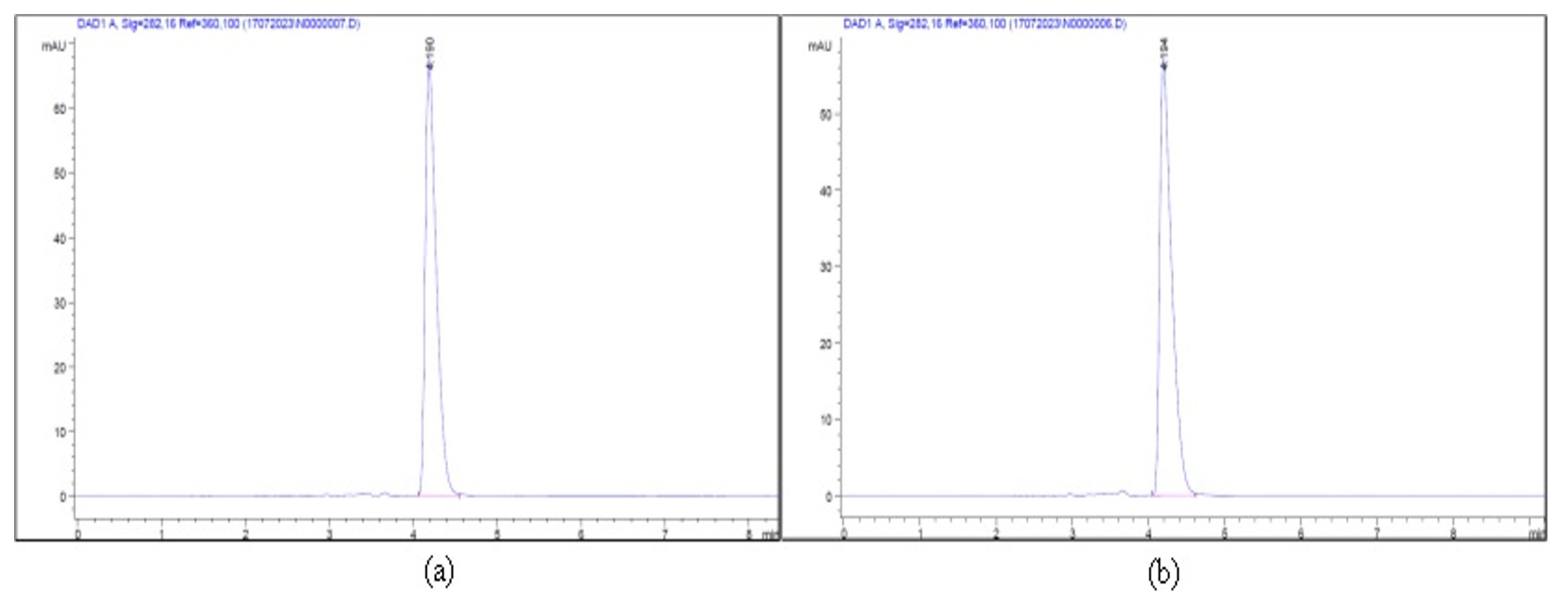
Figure 10:
Chromatogram depicting (a) NBV Standard (b) NBV assay.
Assay of Marketed tablets
The assay of the marketed tablet was carried out by the developed method and drug content was found to be 105.60% of the label claim which complies with prescribed standards (90.0% to 110.0%). The chromatogram for the assay study is as shown in Figure 10 (b).
Linearity and Sensitivity analysis
ICH guidelines were used to establish the linearity of the proposed analytical method. Linearity results obtained within the studied concentration range of 10 to 50 μg/mL, with a regression coefficient value of 0.999 and equation of the linear line obtained is y=15.215x-6.625. The LOD and LOQ represents the sensitivity of the method. The LOD and LOQ values for the method developed were found to be 0.54 μg/mL and 1.64 μg/mL respectively. These finding shows the high sensitivity of the method.
Precision
The results of precision study are presented in Table 6 the %RSD value for peak area in terms of interday and intraday precision study was obtained below 2% which indicates that method is precise for routine analysis.
Analyte | Conc. (μg/mL) | Intraday (n=3) | Inter day (n=3) | ||||||
---|---|---|---|---|---|---|---|---|---|
Day 1 | Day 2 | Day 3 | |||||||
Peak area | % RSD | Peak area | % RSD | Peak area | % RSD | Peak area | % RSD | ||
NBV | 10.00 | 147.82 | 0.02 | 147.92 | 0.07 | 155.82 | 1.43 | 147.47 | 0.04 |
30.00 | 441.76 | 0.10 | 442.70 | 0.10 | 451.85 | 1.57 | 442.85 | 0.05 | |
50.00 | 771.62 | 0.02 | 761.02 | 1.47 | 778.71 | 0.06 | 764.96 | 0.73 |
Intraday and inter-day precision of NBV.
Accuracy study
Method accuracy signifies its capability to align experimental results with theoretical expectations. The accuracy study results, as presented in Table 7, reveal that the recovered concentration of NBV falls within the range of 102.49 to 103.44%. Additionally, the % RSD value is less than 2%, demonstrating the high accuracy of the method.
Compound | Contents (μg/mL) | Quantity added (μg/mL) | Theoretical amount (μg/mL) | Recovered amount (μg/mL) | Recovery (%) | % RSD |
---|---|---|---|---|---|---|
NBV | 10 | 8 (80%) | 18 | 18.28 | 103.44 | 0.02 |
10 (100%) | 20 | 20.27 | 102.71 | 0.12 | ||
12 (120%) | 22 | 22.30 | 102.49 | 0.20 |
Recovery studies of NBV (n=3).
Robustness
The robustness of the study evaluated the process parameters and it was observed that the % RSD value remained consistently below 2 percent, well within the specified limits. The chromatogram showed no noticeable change, indicating that the developed RP-HPLC procedure is indeed robust (Table 8).
Robustness | |||||
---|---|---|---|---|---|
Change of Flow Rate | 0.7 mL | 0.9 mL | |||
Sl. No. | Conc μg/mL | Area | Sl. No. | Conc μg/mL | Area |
1 | 40 | 687.6149 | 1 | 40 | 535.0284 |
2 | 40 | 687.9306 | 2 | 40 | 534.8267 |
Mean | 687.77 | Mean | 534.93 | ||
SD | 0.22 | SD | 0.14 | ||
%RSD | 0.03 | %RSD | 0.03 | ||
Mobile Phase | 70 MeOH+30% | 72 MeOH+28% | |||
Sl. No. | Conc μg/mL | Area | Sl. No. | Conc μg/mL | Area |
1 | 40 | 601.7283 | 1 | 40 | 602.4002 |
2 | 40 | 601.4721 | 2 | 40 | 602.5197 |
Mean | 601.6 | Mean | 602.46 | ||
SD | 0.18 | SD | 0.08 | ||
%RSD | 0.03 | %RSD | 0.01 | ||
Wavelength Change | 281 nm | 283 nm | |||
Sl. No. | Conc μg/mL | Area | Sl. No. | Conc μg/mL | Area |
1 | 40 | 594.9376 | 1 | 40 | 605.8355 |
2 | 40 | 594.5432 | 2 | 40 | 605.5332 |
Mean | 594.7 | Mean | 605.68 | ||
SD | 0.28 | SD | 0.21 | ||
%RSD | 0.05 | %RSD | 0.04 | ||
Ruggedness | |||||
Analyst | Conc μg/mL | Area | Amt Found | ||
1 | 20.00 | 306.92 | 19.743261 | ||
2 | 20.00 | 306.627 | 19.723997 | ||
Mean | 306.77 | 19.73 | |||
SD | 0.207 | 0.014 | |||
%RSD | 0.068 | 0.069 |
Result of robustness and ruggedness study.
Ruggedness
The ruggedness of the developed method was assessed by analyzing the prepared samples by different analysts. There were no any significant differences in observed responses (%RSD <2%) which indicates that the method is rugged (Table 8).
DISCUSSION
The RP-HPLC method for analyzing NBV was fine-tuned using AQbD principles and BBD. Optimal conditions were found with a mobile phase of MeOH:0.1% aqueous acetic acid (71:29), a flow rate of 0.8 mL/min and a wavelength of 282 nm, resulting in well-defined peaks at 4.19 min. A risk assessment focused on key factors: Peak Area (PA), Retention time (Rt) and Tailing factor (Tf). Using DoE software, a quadratic model with a high R2 value of 0.9999 was selected. The impact of variables on responses was clear: methanol and flow rate affected retention time negatively, while wavelength had a positive effect. Peak area was influenced positively by the mobile phase and wavelength but negatively by the flow rate. Tailing factor was positively affected by the mobile phase and flow rate but negatively by wavelength. Graphical and numerical optimization highlighted the relationship between variables and responses, with the numerically optimized method achieving a high desirability of 0.940. Method validation ensured system suitability, specificity, linearity (R2=0.999), sensitivity (LOD=0.54 μg/mL, LOQ=1.64 μg/mL), precision (%RSD<2%), accuracy (recovery 102.49% to 103.44%, %RSD<2%) and robustness (%RSD<2%). Ruggedness was confirmed by different analysts. Analyzing marketed tablets using the method resulted in drug content within standards (105.60% of label claims). In conclusion, the optimized RP-HPLC method, validated for NBV analysis, is robust and precise, making it suitable for routine use in pharmaceutical analysis.
CONCLUSION
In the present study a simple, precise, robust RP-HPLC method was successfully developed for analysis of NBV with the aid of Analytical Quality by Design approach. The study utilizes Box-Behnken Design as a tool of optimization. Various analytical parameters optimized were mobile phase, wavelength and flow rate. The optimized conditions obtained were mobile phase (MeOH:0.1% aqueous acetic acid 71:29% v/v), wavelength (282 nm) and flow rate (0.8 mL/min). The effect of these analytical conditions on retention time, peak area and tailing factor was assessed. The developed method was validated as per ICH guidelines. The study concludes that AQbD can be effectively utilized for development of the RP-HPLC method for the analysis of NBV.
Cite this article:
Randive KH, Maste MM, Kempwade AA. Exploration of AQbD Approach for Development of RP-HPLC Method of Nebivolol. Int. J. Pharm. Investigation. 2024;14(3):915-27.
ACKNOWLEDGEMENT
The Authors would like to express their sincere gratitude to Dr. Sunil S Jalalpure, Principal, KLE College of Pharmacy, KAHER, Belagavi, Karnataka, India for his constant support and providing the necessary analytical infrastructure to carry out the research work. Additionally, authors are also thankful to the Principal KLE College of Pharmacy, Nipani, Karnataka, India for consistent encouragement and support throughout the research work.
ABBREVIATIONS
AQbD | Analytical quality by Design |
---|---|
ANOVA | Analysis of Variance |
ATP | Analytical Target Attributes |
BBD | Box-Behnken design |
CAA | Critical Analytical Attributes |
CMAs | Critical Material Attributes |
CPPs | Critical Process Parameters |
CQA | Critical Quality Attributes |
NBV | Nebivolol |
DOE | Design of Experiment |
ICH | International Council for Harmonization |
LOD | Limit of Detection |
LOQ | Limit of Quantification |
OPA | Ortho Phosphoric Acid |
PA | Peak Area |
QbD | Quality by Design |
RA | Risk Assessment |
RSD | Residual Standard Deviation |
RT | Retention Time |
NTP | Number of Theoretical Plates |
RP-HPLC | Reverse Phase-High Performance Liquid Chromatography |
TF | Tailing Factor |
References
- Ingle U, Lali A. Preparative separation of nebivolol isomers by improved throughput reverse phase tandem two-column chromatography. Chirality. 2020;32(11):1324-35. [PubMed] | [CrossRef] | [Google Scholar]
- Nandania J, Rajput SJ, Contractor P, Vasava P, Solanki B, Vohra M, et al. Quantitative determination of nebivolol from human plasma using liquid chromatography-tandem mass spectrometry. J Chromatogr B Analyt Technol Biomed Life Sci. 2013;923-924:110-9. [PubMed] | [CrossRef] | [Google Scholar]
- Nandania J, Rajput SJ, Contractor P, Vasava P, Solanki B, Vohra M, et al. Quantitative determination of nebivolol from human plasma using liquid chromatography-tandem mass spectrometry. Journal of Chromatography B. 2013;923-924:110-9. [CrossRef] | [Google Scholar]
- Peraman R, Bhadraya K, Padmanabha Reddy Y. Analytical quality by design: a tool for regulatory flexibility and robust analytics. Int J Anal Chem. 2015;2015:868727 [PubMed] | [CrossRef] | [Google Scholar]
- Hakiem AF, Hamdy AK, Ali HR, Gomaa M, Aboraia AS. In depth investigation of the retention behavior of structurally related β-blockers on RP-HPLC column: quality by Design and quantitative structure-property relationship complementary approaches for optimization and validation. J Chromatogr B Analyt Technol Biomed Life Sci. 2021;1166:122549 [PubMed] | [CrossRef] | [Google Scholar]
- Vogt FG, Kord AS. Development of Quality-by-Design analytical methods. J Pharm Sci. 2011;100(3):797-812. [PubMed] | [CrossRef] | [Google Scholar]
- Alam P, Shakeel F, Taleuzzaman M, Foudah AI, Alqarni MH, Aljarba TM, et al. Box-Behnken design (BBD) application for optimization of chromatographic conditions in RP-HPLC method development for the estimation of thymoquinone in Nigella sativa Seed Powder. Processes. 2022;10(6):1082 [CrossRef] | [Google Scholar]
- Suryawanshi SS, Palled MS. Box-Behnken design assisted optimization and standardization of chromatographic methodology for quality assessment of metformin: analytical quality by design avenue. IJPER. 2022;56(2s):s152-62. [CrossRef] | [Google Scholar]
- Tome T, Žigart N, Časar Z, Obreza A. Development and optimization of liquid chromatography analytical methods by using AQbD principles: overview and recent advances. Org Process Res Dev. 2019;23(9):1784-802. [CrossRef] | [Google Scholar]
- Mandpe SR, Parate VR, Naik JB. Experimental design approach, screening and optimization of system variables, analytical method development of flurbiprofen in nanoparticles and stability-indicating methods for high-pressure liquid chromatography. Future J Pharm Sci. 2022;8(1):1-21. [CrossRef] | [Google Scholar]
- Patil TS, Deshpande AS. Development of an innovative quality by design (QbD) based stability-indicating HPLC method and its validation for clofazimine from its bulk and pharmaceutical dosage forms. Chromatographia. 2019;82(2):579-90. [CrossRef] | [Google Scholar]
- Saini S, Sharma T, Patel A, Kaur R, Tripathi SK, Katare OP, et al. QbD-steered development and validation of an RP-HPLC method for quantification of ferulic acid: rational application of chemometric tools. J Chromatogr B Analyt Technol Biomed Life Sci. 2020;1155:122300 [PubMed] | [CrossRef] | [Google Scholar]
- Saini S, Sharma T, Patel A, Kaur R, Tripathi SK, Katare OP, et al. QbD-steered development and validation of an RP-HPLC method for quantification of ferulic acid: rational application of chemometric tools. Journal of Chromatography B. 2020:1155 [CrossRef] | [Google Scholar]
- Alruwaili NK. Analytical Quality by Design approach of reverse-phase high-performance liquid chromatography of atorvastatin: method development, optimization, validation and the stability-indicated method. Int J Anal Chem. 2021;2021:8833900 [PubMed] | [CrossRef] | [Google Scholar]
- Patil TS, Deshpande AS. Development of an innovative quality by design (QbD) based stability-indicating HPLC method and its validation for clofazimine from its bulk and pharmaceutical dosage forms. Chromatographia. 2019;82(2):579-90. [CrossRef] | [Google Scholar]