ABSTRACT
Artificial Intelligence (AI) is ushering in a transformative era for personalized medicine by customizing therapeutic interventions based on individual patient profiles, including genetic information and environmental influences. Through the analysis of intricate biological datasets, AI significantly improves diagnostic accuracy, prognostic assessments and therapeutic planning, thereby enabling more precise patient stratification and enhanced clinical outcomes. This advancement is particularly evident in the realms of genomic analysis and pharmacogenomics. Nonetheless, the integration of AI into healthcare introduces substantial legal and ethical considerations. Foremost among these are concerns related to data privacy, security and equitable access to treatment. It is imperative to address these issues to effectively leverage the full potential of AI. Achieving this requires a reevaluation of regulatory frameworks and an enhancement of training programs for healthcare professionals. As AI continues to advance within the healthcare sector, it promises to fundamentally redefine disease management and prevention, heralding a new epoch of medical innovation.
INTRODUCTION
Self-healing medicine powered by artificial intelligence is gaining acceptance from the medical industry, policymakers and regulators. The integration of AI and personalized medicine is expected to improve treatment and patient safety while reducing healthcare costs. In oncology, AI-informed molecular diagnostics are guiding treatment options, giving each patient the best chance of survival. AI is also enhancing the accuracy of HLA genotyping, leading to better transplant outcomes and more accurate predictions of high antibody levels. Additionally, intelligent genotyping of drug-metabolizing enzymes can increase the understanding of genetic variation, thereby providing better treatment for patients while reducing side effects.
AI also enables the analysis of individual and environmental differences, offering new insights for medical treatments and prevention models. There is a shift from a one-size-fits-all approach to treatments tailored to the specific needs of each patient. Background information plays a crucial role in distinguishing personalized medicine from standard prevention and treatment guidelines. The emergence of new genetic testing technologies and molecular diagnostics has further stimulated the development of personalized medicine. By using the molecular uniqueness of each patient, AI can predict whether a patient will benefit from a particular treatment or whether they might experience adverse reactions. The success of AI-driven personalized medicine requires collaboration among researchers, AI developers, analysts, regulators, healthcare professionals, physicians and patients (Jiang & Zhang, 2023).
Understanding Personalized Medicine
The concept of self-medication has gained popularity in recent years, driven by the recognition that conventional treatments can often be ineffective or unsafe and by advances such as the Human Genome Project. These advances facilitate the identification of disease subtypes based on genetic data and other methods like histology, which many believe will enhance disease prevention and pain management. For instance, genetic studies can reveal that patients with certain conditions are more likely to benefit from specific new or existing drugs. Artificial Intelligence (AI) plays a crucial role in analyzing data, drawing conclusions, identifying new relationships and supporting doctors in their decision-making. Companies have demonstrated that supercomputers, deep learning and AI can significantly enhance the accuracy of personalized medicine.
This article focuses on creating universal solutions for treating patients with similar symptoms while incorporating evidence-based practices. The evolution of medicine from Hippocrates to the twentieth century highlights a shift from acquiring knowledge to relying on evidence. Technological advancements and treatment innovations are transforming how doctors diagnose and treat patients. Modern medical decisions are increasingly based on research and clinical trials, offering access to advanced treatments and necessitating thorough research on potential side effects. Innovations such as affordable genome sequencing, advanced biotechnology and health sensors integrated into daily life are accelerating this change. However, the influx of information and the digital health revolution driven by smartphones and healthcare providers have introduced data analysis and interpretation challenges (Redekop & Mladsi, 2013).
According to the National Institutes of Health, personalized medicine develops disease and prevention strategies based on an individual’s genetics, environment and bioactivity. This approach enhances the ability of doctors and researchers to predict the most effective treatments and preventive measures for individuals (Redekop & Mladsi, 2013; Collins & Varmus, 2015). AI functions similarly to a supercomputer, utilizing fast-learning algorithms like deep learning to achieve high accuracy in treating diseases, often matching, or surpassing human expertise in fields such as cardiology, dermatology and oncology (Gilvaryet al., 2019).
Nevertheless, it is crucial to emphasize the integration of intelligent algorithms with human expertise. While AI can provide accurate diagnoses, combining its capabilities with doctors’ knowledge and experience is essential for optimal patient care (Johnsonet al., 2021). For instance, at the World Biomedical Imaging Symposium, a computational model for identifying metastatic breast cancer in lymph node images achieved a success rate of 92.5% in a competition. This rate increased to 96.6% with the addition of doctors’ evaluations and combining AI predictions with human diagnoses raised the success rate to 99.5%, reducing human error by approximately 85% (Johnsonet al., 2021).
In personalized medicine, AI serves as a powerful tool for accurate diagnosis and reduced human error, especially when complemented by clinical expertise. The shift from traditional methods to personalized medicine exemplifies the transition to more precise, data-driven healthcare. This evolution underscores the need to balance technological advancements with clinical knowledge to uphold high standards in patient care. As the field progresses, it presents both unprecedented opportunities and challenges that require ongoing collaboration among experts, practitioners and policymakers to fully realize its potential (Davenport & Kalakota, 2019).
Current Technologies and Methodologies
The growth of AI-driven personalized medicine is propelled by several key technological advancements:
- Genomic Sequencing: Advanced sequencing technologies have made it possible to quickly and affordably decode genetic information. This enables the detection of genetic variations linked to diseases and treatment responses, allowing for more personalized medical care.
- Bioinformatics: By applying powerful computational methods, bioinformatics helps analyze large-scale biological data, uncovering disease-related genes and pathways. This deepens our understanding of disease mechanisms and Aids in the development of targeted therapies.
- Machine Learning and Predictive Analytics: These tools use algorithms to analyse data from various sources, predicting disease risks and treatment outcomes. Their ability to process extensive datasets enhances clinical decision-making and supports the creation of personalized treatment plans.
- Wearable Health Devices: These devices offer continuous monitoring of vital signs and lifestyle habits, providing real-time data that can be used to tailor medical treatments. They are crucial in the proactive management and prevention of diseases by delivering insights into a patient’s daily health.
- Electronic Health Records (EHRs): EHRs serve as digital repositories that consolidate patient data, making it easier to incorporate personalized medicine into routine clinical practice. They offer a valuable source of information that can be analyzed with AI to improve patient care and treatment outcomes.
AI and the Future of Personalized Medicine
The combination of AI and Personalized medicine has the potential to revolutionize healthcare. Personalized medicine focuses on identifying patient phenotypes with poor clinical response or specific treatment needs. AI uses computation and reasoning to understand and empower healthcare professionals through artificial intelligence. Recent studies suggest that research on the integration of intelligence with personalized medicine can solve important problems, especially the integration of genomic and non-genomic markers with patients’ symptoms, clinical history and lifestyle to facilitate individual diagnosis and prognosis (Greenberget al., 2020). The report emphasizes the need for caution when using these technologies, but also recognizes their significant promise. The digitalization of health information and the rapid use of technology are accelerating progress in the use of AI in healthcare. However, integrating multiple data sources, ensuring security and overcoming challenges related to government training, performance standards and biases are important issues that need to be addressed (Holzingeret al., 2017). The use of AI is essential: data security and transparency, analysis and visualization and integration. While data security provides transparency and trust in AI systems, analytics and insights provide support to healthcare professionals. Experts share the collaboration between humans and AI that supports the development of new skills and the need for cutting-edge AI models and effective business practices (Holzingeret al., 2017).
Sl. No. | Applications of AI in Healthcare | Impact of artificial intelligence in different sectors within healthcare |
---|---|---|
1 | AI for Drug Discovery | AI accelerates the drug discovery process by automating the identification of drug targets and assessing potential compounds. Leading pharmaceutical companies like Pfizer and Sanofi are incorporating AI tools to streamline the development of new treatments. AI is anticipated to transform drug development by making it faster, more cost-effective and more efficient. |
1.1 | AI for Clinical Trials | AI enhances the clinical trial process by automating data monitoring and improving the accuracy of results. Key applications of AI in clinical trials include: |
1.2 | Intelligent Clinical Trials | AI helps to optimize clinical trials by analyzing Real-World Data (RWD) and employing predictive models. These advancements enable researchers to gain deeper insights into diseases, identify suitable patients and design innovative trial protocols. AI also aids in data management, reducing human error and ensuring more reliable results. |
1.21 | Clinical Trial Cooperation and Model Sharing | Effective global collaboration and data sharing are crucial for advancing AI applications in clinical trials. Initiatives for scalable data-sharing and open science contribute to the rapid development and deployment of AI solutions. Projects like EIOS leverage open-source data to improve public health responses and early detection of health threats. |
1.3 | Patient Care | AI contributes to enhanced patient care by providing systems that generate valuable insights and improve the quality of life. These AI-driven systems support various aspects of patient care. |
1.31 | Maternal Care | AI aids in predicting risks for pregnant women and improves access to both routine and specialized care. This can lead to a reduction in complications and mortality for high-risk pregnancies by delivering timely and precise care recommendations. |
1.32 | Healthcare Robotics | Medical robots, including exoskeletons and advanced prosthetics, assist with rehabilitation and surgical procedures. For instance, Cyberdyne’s HAL exoskeleton helps individuals with lower limb disorders by detecting electrical signals and enabling joint movement, thus supporting recovery from conditions like spinal cord injuries and strokes. |
1.33 | Genetics AI Data-Driven Medicine | The integration of AI and extensive data collection enhances the detection of genetic diseases and facilitates personalized medical treatments. This approach boosts the precision and adaptability of healthcare by utilizing comprehensive data from diverse sources. |
1.34 | AI-powered Stethoscope | AI-enabled stethoscopes provide accurate readings even in noisy environments and are easy to use. They improve medical care in remote areas and for patients with chronic conditions, while also reducing the risk of COVID-19 transmission. AI can analyze large volumes of clinical data to identify disease patterns and abnormalities, enhancing diagnostic accuracy. |
Applications of AI in Healthcare.
A vast amount of data influencing our health exists outside traditional medical systems. Factors such as lifestyle, diet, environmental factors, healthcare accessibility and access to care significantly impact our health. These behavioral and social determinants can be tracked through wearables and medical devices. Over our lifetimes, we generate extensive Individual and health data that could offer insights for a longer, healthier life. Large-scale data analysis and AI are progressively prevalent in healthcare, impacting payers, providers, policymakers, patients and product manufacturers. AI-powered tools assist in reducing fraud, waste and abuse in payer programs, saving significant amounts of money, time and effort. For instance, IBM’s AI-based tool, Data Probe, detected and recovered substantial funds in medical fraud cases. In the healthcare sector, AI is in evidence-based clinical decision-making and forecasts patient readmission risks. Policymakers and governments leverage AI to manage and predict infections and outbreaks, exemplified by the FINDER model for detecting foodborne illnesses. During crises, AI-enabled solutions have integrated data and services to streamline care coordination, as seen in Sonoma County’s response to wildfires. AI is not the only data-driven field influencing health care. Personalized medicine, with its goal of personalizing care for every individual, also plays a significant role. Personalized medicine relies on extensive data, such as that collected through biobanks and health projects and a healthcare ecosystem willing to adopt personalized strategies. The integration of AI and Personalized medicine is anticipated to accelerate the achievement of personalized care goals. As already discussed, the digitalization of health information and the rapid use of technology are accelerating progress in the use of AI in healthcare.
AI in Healthcare
The acceptance of AI has increased in many areas, especially healthcare, in the last decade. While AI offers the opportunity to create smart products, create new services and create new business models, it also brings with it social and ethical issues related to security, privacy and human rights. AI technologies range from virtual machines such as deep-learning information for the management of health to cyber-physical systems such as surgical robots and nanorobots for drug delivery. AI’s ability to recognize complex patterns makes image-based diagnosis as effective as or better than doctors. AI-based medical decision-making processes can reduce misdiagnoses and support decision-making by processing EHR data. Advances in computing resources such as natural language processing and pattern recognition will empower AI to solve complex problems.
AI supports and enhances human capabilities, making the role of AI in healthcare clearer. AI systems; provide skills such as perception, reasoning, learning and motivation that support human skills such as understanding, reasoning and creativity. Insights can be developed in oncology, imaging and primary care. For example, AI algorithms trained on mammogram images and EHR data can predict breast cancer with higher accuracy than radiologists, potentially reducing the number of diagnoses. Personalized medicine is a rapidly growing field that focuses on personalized medicine based on individual characteristics. Initially characterized as the development of a novel theory of human disease grounded in molecular biology, this theory now combines multidisciplinary data with clinical history, social decision-making/behavior and environmental factors to determine health and disease states. Personalized medicine enables doctors to deliver personalized care based on individual characteristics. The adoption of genotyping and electronic health records allows researchers to derive new phenotypes from clinical data, thereby improving diagnosis and treatment planning. A great work in personalized medicine that helps determine the accuracy of medicine and provides treatment plans for cancer patients. Integration of Personalised medicine into healthcare promises to provide more accurate diagnosis, disease risk prediction and treatment plans. The creation of data repositories such as biobanks supports a different world of personalized medicine training algorithms and addressing governance, privacy and governance.
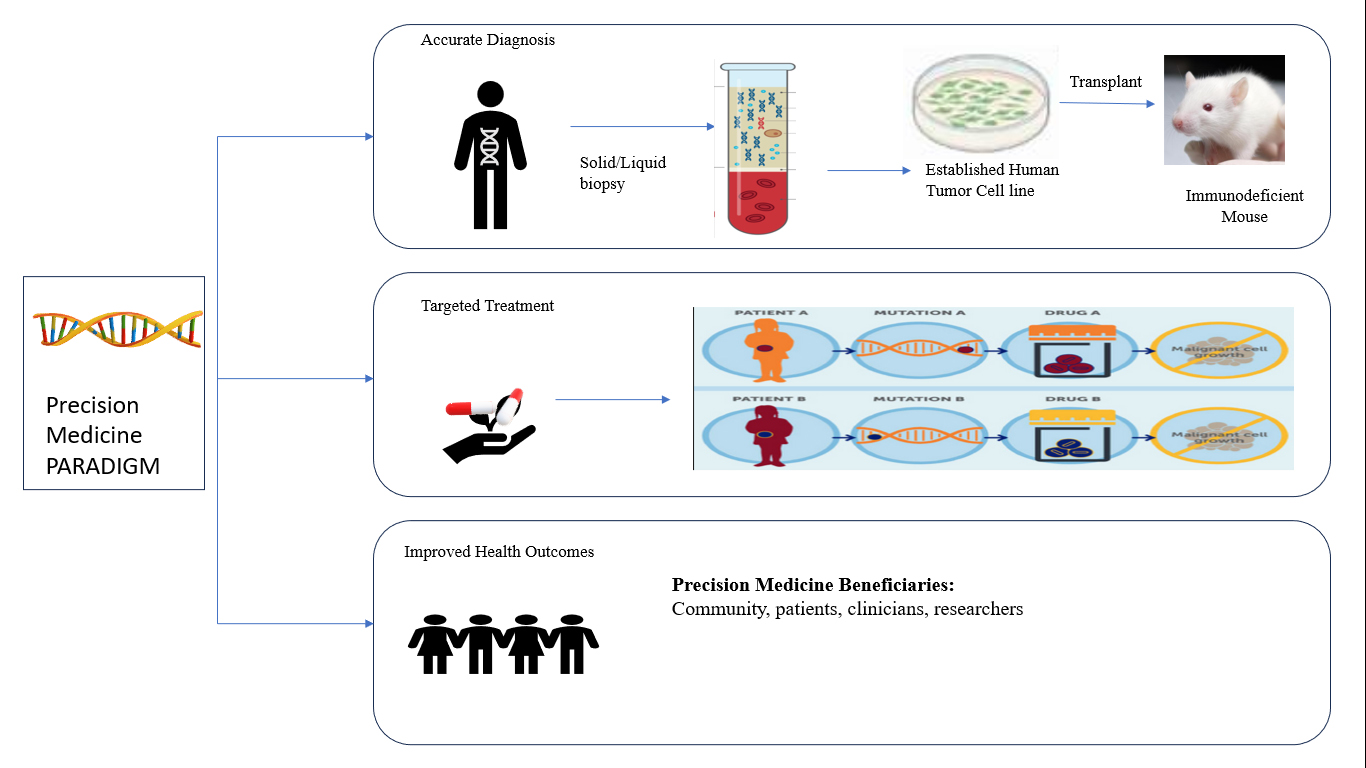
Figure 1:
Personalized medicine Data quality relevance.
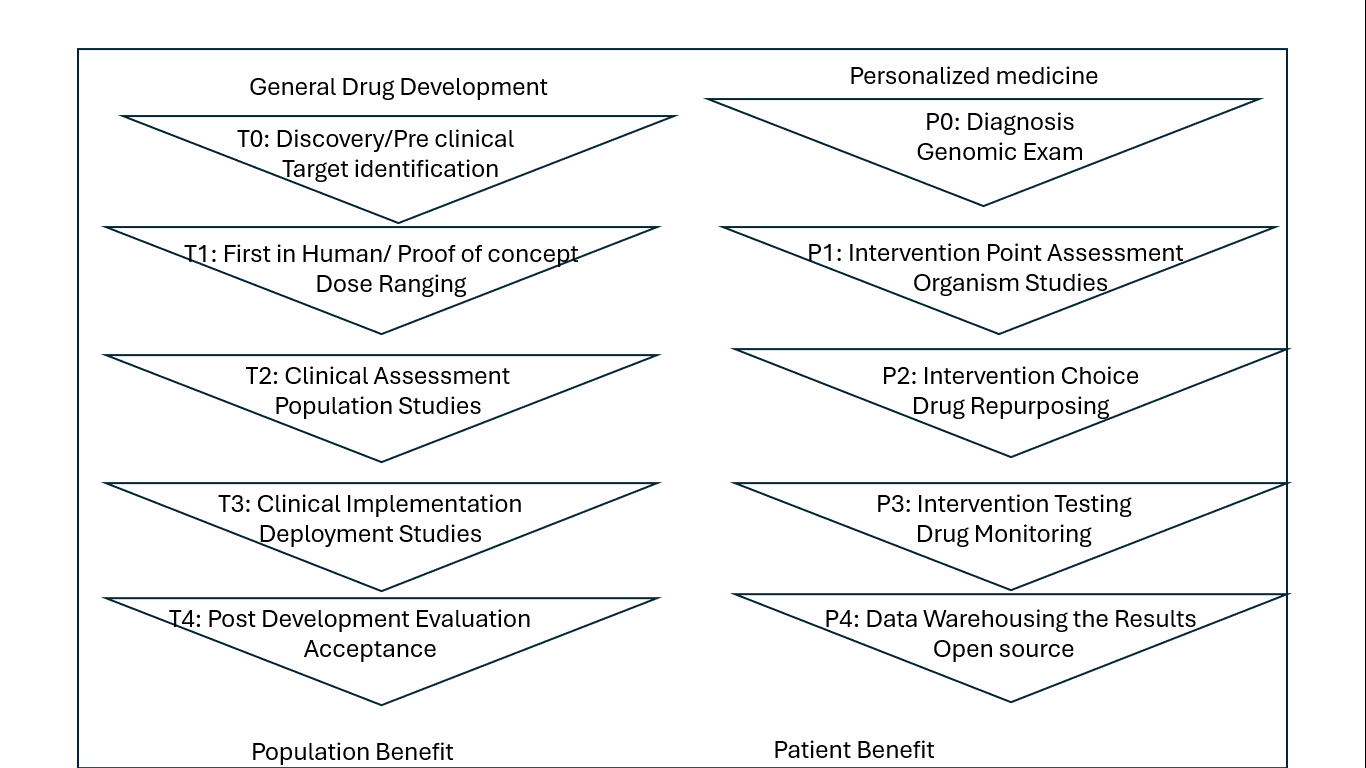
Figure 2:
Work flow in the personalized medicine Federal Policies Shaping AI-Driven Personalized Medicine.
Applications for AI in Healthcare
AI is rapidly transforming healthcare by addressing critical challenges such as rising costs, limited access and the need for more efficient processes. AI’s ability to analyze vast data sets allows for enhanced diagnostics, personalized treatments and streamlined operations. The following Table 1 outlines key applications of AI in healthcare, showcasing how this technology is being utilized to improve patient outcomes and operational efficiency.
AI for Personalized Medicine: Data Accuracy and Applicability
Although AI has made significant strides in recent times, its potential in clinical research, gaps and opportunities for improvement remain. Highlights the need for either more data or improved algorithms to further enhance AI capabilities. Currently, AI focuses on developing algorithms that require large amounts of data to optimize. This is because current machine learning methods are too data-intensive. Comprehensive data combined with simple algorithms have been shown to provide better results compared to complex algorithms using limited data. This means that advanced AI systems work best when variability is low, which is usually not a problem in clinical practice. It plays an important role in thinking about reality. The quality of the predictions made by the model cannot exceed the quality of the data used for training. Although traditional statistical methods can filter data by removing outliers, their effectiveness is limited because they cannot measure the quality and accuracy of the data. Therefore, inaccuracies in the data content can introduce bias and affect the study sample. To overcome these challenges in AI, particularly when handling complex data, researchers leverage data analysis, including sensitive analysis, feature selection and model reduction. Relationships are also important for specific diseases. Most recent efforts to apply ML techniques are based on complex tools that have been studied without appropriate analysis for many diseases as depicted in Figure 1 (Gulshanet al., 2016).
AI-driven personalized Medicine in Practice
AI technologies are revolutionizing healthcare by providing innovative solutions for personalized medicine, drug discovery, diagnostics and treatment optimization. Below are some key examples of how AI is being harnessed in these areas:
- Curate. AI: Dose Recommendation Platform: CURATE.AI is a pioneering AI-driven platform designed to optimize drug dosing for individual patients. By mapping the relationship between drug dose (input) and patient response (output), it creates a personalized profile for each patient based on their unique data. This profile dynamically adjusts as the patient’s condition changes over time, recommending the most effective dosing strategies to achieve the best possible therapeutic outcomes. Unlike traditional methods, it incorporates complex biological phenomena without explicitly modelling them, making it a robust and mechanism-independent tool for personalized treatment (Blasiaket al., 2020).
- Quadratic Phenotypic Optimization Platform (QPOP): QPOP is another innovative AI technology focused on optimizing combination therapies. It utilizes a quadratic phenotypic optimization model to determine the most effective drug combinations and their initial doses. By analyzing how different drug combinations affect phenotypic outcomes, QPOP helps in selecting the best therapeutic strategies, especially in complex diseases where multi-drug regimens are necessary. This platform minimizes the trial-and-error approach traditionally associated with drug combination therapy, improving both efficacy and safety (Lin & Ho, 2018).
- IBM Watson for Oncology: IBM Watson for Oncology is an AI-powered decision-support system that assists oncologists in selecting the most appropriate cancer treatments. By analyzing vast amounts of medical literature, clinical trial data and patient records, Watson provides evidence-based treatment recommendations tailored to individual patients. This system enhances the decision-making process, helping clinicians choose the most effective therapies based on the latest research and patient-specific factors (Ferrucciet al., 2010).
- Path AI: AI-Powered Pathology: Path AI is an AI-driven platform that improves the accuracy and efficiency of pathology diagnostics. Using machine learning algorithms, Path AI analyzes medical images, such as tissue biopsies, to identify patterns and diagnose diseases with high precision. This technology is particularly valuable in cancer diagnostics, where early and accurate detection is critical for effective treatment. Path AI not only assists pathologists in making more accurate diagnoses but also reduces the time required for analysis, enabling faster clinical decision-making (Howardet al., 2021).
- DeepMind’s AlphaFold: AlphaFold, developed by DeepMind, is an AI system that predicts the 3D structures of proteins based on their amino acid sequences. This breakthrough technology has significant implications for drug discovery and development, as understanding protein structures is key to identifying how drugs interact with their targets. AlphaFold’s ability to accurately predict protein folding has accelerated research in various biomedical fields, opening new avenues for the development of targeted therapies (Jumperet al., 2021).
Integrating Personalized Medicine into the Workflow
To ensure seamless integration, the concept of personalized medicine must extend beyond isolated innovations and into the broader healthcare workflow. By combining individual and collaborative drug activity within a unified process, personalized medicine can transform patient care across all levels, from diagnosis to treatment and monitoring. This holistic approach is vital for realizing the full potential of AI-driven solutions in enhancing patient outcomes and enabling more precise, tailored therapies. Combined and individual drug activity: P0-P4 Individual Drug Activity is like the T0-T4 Drug and Medical Equipment Development Study. For these projects to work effectively, change needs to be achieved at all levels, although different elements require different skills and ideas. Traditionally these differences have led to differences, but emerging ideas in personalized medicine, particularly cognitive, promise to enable integration and shared efficiency of patient care across the P0-P4 workflow. Ideal scenario Personalized medicine enables the transformation of all levels by integrating diagnosis, treatment and monitoring into an integrated process. An important example is the development of cell transplantation for various diseases. For example, in some cancer immunoassays, a patient’s tumor may be analyzed for specific neoantigens, or mutations, that trigger the body’s immune response against cancer cells. If neoantigens are identified, the immune system can be harvested from the donor (allogeneic transplant) or the patient (autologous transplant) and then trained to recognize the neoantigens. The aim is for the sensitized cells to cause the patient’s immune system to attack the tumor cells. This approach, called controlled production, suits the immediate needs of patients, unlike pharmaceutical production, which the medical center reserves for future use. The management approach will become more common in personalized medicine than in cancer treatment because it is not good to plan all treatment options. Production management, artificial intelligence-supported robots and 3D printers can be used for quality production. The FDA approved the first 3D-printed drugs in 2015, demonstrating the potential of point-of-care manufacturing in medical care. AI can also instantly support N-of-1 testing, where treatments are personalized and their results carefully monitored using advanced technology. AI-based simulations can also help predict possible treatment strategies, further improving patient care as depicted in Figure 2 (Keerthanaet al., 2020).
Federal policies play a fundamental role in guiding the development and implementation of AI-driven personalized medicine, ensuring that the balance between encouraging innovation and protecting patient safety is maintained. These policies set the groundwork for a healthcare landscape that is increasingly tailored to individual patient needs, driven by advancements in technology and data science.
Key Federal Initiatives and Regulations
- Personalzsed Medicine Initiative (PMI): In 2015, PMI sought to advance personalized medicine by tailoring treatments to individual genetic, environmental and lifestyle factors, thereby enhancing therapeutic outcomes
- HIPAA Privacy Rule and GINA: These regulations protect patient privacy and genetic information, building trust and ensuring ethical practices in applying personalized medicine.
- 21st Century Cures Act: Enacted in 2016, this legislation accelerates the development and approval of medical products, emphasizing the integration of real-world evidence and advanced biomarkers critical for personalized medicine strategies.
- FDA Personalized Medicine Framework: The FDA has developed a regulatory framework.
- FDA’s Role: The Food and Drug Administration (FDA) is actively adapting its regulatory practices to accommodate the advancements in AI and personalized medicine. This includes creating new approval pathways and establishing specific guidelines for AI-driven technologies, ensuring that they meet rigorous safety and effectiveness standards before they can be widely implemented.
- NIH’s Contribution: The National Institutes of Health (NIH) is a key player in promoting the development of personalized medicine, particularly through initiatives like the All of Us Research Program. This program seeks to collect various health data from diverse populations to drive research and ultimately improve healthcare outcomes. By supporting such initiatives, the NIH is helping lay the groundwork for more effective and personalized treatment options.
Supportive Aspects
Federal initiatives like the Personalized Medicine Initiative (PMI) andthe 21st Century Cures Acthavesignificantlypropelledresearch and development in personalized medicine by providing essential funding and streamlining regulatory pathways. These efforts have accelerated innovation and ensured that new treatments can reach patients more efficiently. Furthermore, regulations such as the Health Insurance Portability and Accountability Act (HIPAA) and the Genetic Information Non-discrimination Act (GINA) are crucial in safeguarding patient data. This protection is vital for maintaining ethical standards in the application of AI technologies in medicine, where data privacy and security are paramount (Magrabiet al., 2019).
Analysis of AI-driven Personalized Medicine and Federal Policies
The convergence of AI with personalized medicine marks a significant evolution in patient care, offering the potential for more tailored and effective treatments. Federal policies and regulations are critical in shaping this field, ensuring that technological progress occurs responsibly and ethically. This analysis examines the interplay between federal policies and AI advancements, addressing both the challenges and opportunities of integrating these technologies within existing regulatory frameworks. It also presents case studies showcasing how these policies have influenced the development of AI-driven solutions. Federal policies are integral in promoting AI innovation within personalized medicine, providing a regulatory backbone while encouraging advancements. Initiatives like the Personalized Medicine Initiative (PMI) and the 21st Century Cures Act are pivotal in supporting AI’s integration into healthcare, ensuring that patient safety remains a top priority. These programs enable the responsible application of AI in personalized medicine by balancing innovation with stringent safety standards. However, aligning AI developments with current regulations presents several hurdles. These include modernizing outdated policies to account for new technologies and tackling issues related to proprietary algorithms and data privacy. Despite these difficulties, there are opportunities for regulatory adaptation, as seen in the FDA’s Digital Health Innovation Action Plan. This initiative represents a proactive effort to update regulatory approaches in line with rapid technological advances, ensuring that AI-based solutions are evaluated ethically and efficiently (Amnesty International, 2024).
Regulatory Power and Epistemic Authority in AI-Driven Personalized Medicine
The FDA’s role is crucial in asserting regulatory authority and setting scientific standards for AI-driven personalized medicine. As Carpenter (2010) describes, the FDA’s influence spans three main areas: directive, conceptual power and gatekeeping.
Directive Power
The FDA exercises directive power by enforcing regulations on AI technologies used in personalized medicine. This can involve requiring modifications to AI algorithms or mandating transparency in how these systems are validated and monitored, ensuring they meet safety and efficacy standards.
Conceptual Power
The FDA’s conceptual power helps shape the regulatory landscape for AI in personalized medicine. This authority builds on the legacy of past regulations, such as the 1962 Kefauver Amendments, which set high standards for drug approvals. Similarly, the FDA is now developing guidelines to ensure AI systems used in personalized medicine meet rigorous evidence and validation requirements.
Gatekeeping Power
The FDA acts as a gatekeeper by evaluating and approving AI tools for personalized medicine before they enter the market. This role is essential for maintaining high standards between the development and clinical application stages. However, the FDA’s oversight diminishes post-market, making it harder to monitor performance and address issues that arise after these AI systems are deployed. Post-market oversight of AI tools in personalized medicine remains a challenge. While the FDA can revise product labeling or require post-market studies, monitoring the ongoing effectiveness of AI systems in diverse clinical environments is complex. Additionally, the use of AI tools beyond their approved purposes often goes unregulated, creating gaps in oversight. The regulatory landscape for AI in personalized medicine involves not just the FDA, but also a range of stakeholders and mechanisms. This results in a polycentric regulatory approach, where multiple entities contribute to the oversight and governance of AI technologies (Timmermans & Berg, 2010).
Challenges and Future Directions
The rapid pace of innovation in AI often surpasses the capacity of existing regulatory frameworks to keep up, posing significant challenges in maintaining the safety and efficacy of new technologies. Additionally, issues related to data sharing and system interoperability remain substantial obstacles. The integration of AI systems across different platforms and institutions can be hindered by these challenges, slowing down the potential benefits of personalized medicine. AI holds undeniable potential to enhance cancer patient care and significantly impact cancer outcomes. In clinical settings, its success has been so profound that it is altering practices in nearly every phase of cancer research and treatment. In healthcare, gathering sufficient data from the entire population is crucial for building robust AI models. It appears that differences in race, gender and socioeconomic status influence the occurrence and recurrence of diseases. For instance, there are ethnic disparities in the occurrence and frequency of genomic abnormalities in cancer. Research by Bhargava and colleagues even revealed racial disparities in bacterial aggression between Caucasian and African American women suffering from breast cancer. However, existing data used to train and diagnose cancer AI models often lack representation from certain groups and races. For instance, the largest database, TCGA, predominantly comprises individuals of European descent. Moreover, biases exist in large datasets, for example, the TCGA dataset has a higher prevalence of solid tumors and a low incidence of metastatic tumors. While cell lines populate huge genomic datasets, they fail to capture real scenarios due to issues like genetic drift. Apart from unbiased information, there is a disparity between the ease of accessing information from various platforms and the ease of independent usage, particularly concerning personal information or access control. As prospective clinical trials and interventional data become more integrated and validated, the clinical efficacy of AI will continue to improve, alongside addressing disparate data influencing practice. Sharing coded cognitive models is another way to ensure transparency, reproducibility and suitability for clinical use. However, universal methods for sharing complex model code effectively are still lacking.
Fortunately, most reputable journals now mandate detailed explanations of the reporting process, enhancing clarity and sharing. Although the model emphasizes imaging and omics data, one of the richest resources, Electronic Health Records (EHRs), remains largely untapped due to its unstructured nature, noise, sparsity, inconsistency and the need for special management and data cleansing. Models like the Healthcare Outcomes-partnership Common Profile model Aim to address these issues, supported by client software enabling visibility into long-term patient information. Establishing trust in artificial intelligence-supported decision-making among physicians is crucial in integration into hospitals. To this end, Begoli and colleagues recommend developing a measure of uncertainty in cognitive models. Model uncertainty can arise from data selection, accuracy, completeness, biases, artifacts and inaccurate models.
Estimating uncertainty in driven data forecasting models is an active research area that will improve model robustness and increase confidence in information, thereby enhancing future decisions. Although deep learning is often termed a “black box,” preserving data relationships is vital. Further research to improve model interpretation and understanding of cellular and molecular mechanisms is crucial. Ground research has led to the compilation of cancer risk factors and technological advancements offer various ways to collect patient information, including genetic testing, EHRs and smartphone sensors. AI systems can improve diagnostic accuracy, enhance self-care, remotely monitor cancer patients and provide personalized options for prevention, early intervention and management of critical situations (Idekeret al., 2011).
Limitations of AI in the Promotion of Personalized Medicine
Despite the potential of AI, the field of personalized medicine still faces some challenges. A major problem is the lack of ergodicity, meaning that models based on big data will not reflect the relationship between individuals. If the model prioritizes public disclosure of the patient’s data, this will make the treatment decision impossible. Some AI tools, such as IBM’s Watson, have shown inconsistent results, underscoring the importance of quality analysis from clinical trials. If an AI system is trained with biased or incomplete data, its recommendations will be unreliable, just like Google’s flu predictions. Additionally, the SHIVA comparative medicine study showed no benefit compared to conventional treatment, raising questions about the effectiveness of the matching algorithm. Use them in learning whose algorithms are constantly updated. This approach requires initially conflicting data to provide reliable results and takes a lot of time for the system to develop high accuracy. AI-based tools that can provide accurate predictions suffer from a black box problem where the connection between inputs and outputs is invisible. This lack of explanation reduces confidence in AI predictions. Additionally, many AI methods focus on correlation rather than causation; this is not enough to identify drug targets that directly address the need. Understanding the relationship and impact is important for effective design (Schmidt, 2017).
Case Studies: The Influence of Federal Policies on AI-Driven Innovation.
- Oncology Personalized medicine: Federal regulations have played a significant role in advancing personalized cancer treatments. For example, the FDA’s expedited approval of AI-powered diagnostic tools demonstrates how supportive policies can accelerate the use of innovative technology in oncology, leading to more precise and individualized cancer care.
- AI in Drug Discovery: Legislation such as the 21st Century Cures Act has been vital in incorporating AI into drug discovery. By fostering the use of AI, this act has sped up the development of new drugs and enhanced the success of clinical trials, contributing to the advancement of personalized medicine.
- Wearable Health Technologies: Federal backing for digital health has fueled the rise of AI-driven wearable devices. These devices provide personalized health insights and early warnings through real-time data, empowering individuals to control their health proactively (Shortliffe, 2019).
AI Employment in Investigation of TNBC
Triple-NEGATIVE BREAST CANCER (TNBC) is an aggressive type of breast cancer that lacks the three main receptors (estrogen, progesterone and HER2), making it harder to treat. This case study explores the spread of cancer (metastasis) and survival rates in patients diagnosed with TNBC. Metastasis in TNBC Metastasis is when cancer cells move from the original tumor to other areas of the body. In TNBC, this process tends to happen more quickly compared to other breast cancer types. TNBC often spreads to distant organs like the lungs, liver and brain. Detecting metastasis early is crucial, as it greatly impacts a patient’s chances of survival. Survival Rates in TNBC Patients TNBC patients generally have lower survival rates than those with other breast cancer types, due to the aggressive nature of the disease and limited treatment options. Early-stage TNBC can often be managed with surgery and chemotherapy, but once the cancer has spread to other parts of the body, treatment becomes more difficult. The five-year survival rate is relatively good for patients without metastasis but drops significantly if the cancer spreads. Factors Affecting Survival The survival of TNBC patients is influenced by factors such as the stage of cancer at diagnosis, the patient’s age, overall health and how well they respond to treatment. Younger patients and those diagnosed earlier typically have better survival outcomes. Research and Treatment Advances Current research is focused on developing new treatments for TNBC, including immunotherapy and targeted therapies. These advancements aim to increase survival rates and reduce the risk of metastasis in those diagnosed with this aggressive cancer. In summary, TNBC is a challenging cancer to treat due to its aggressive nature and tendency to spread. However, ongoing research efforts involving AI offer hope for better treatment options and improved survival outcomes in the future (Cerneaet al., 2019; Shortliffe, 2019).
CONCLUSION
The integration of Artificial Intelligence (AI) into personalized medicine marks a monumental advancement in healthcare, offering extraordinary potential for customized treatment and enhanced patient outcomes. Through the application of advanced technologies such as genomic sequencing, bioinformatics and machine learning, AI significantly augments our capacity to interpret complex biological data and forecast individual responses to therapies. Moreover, the utilization of wearable health devices and Electronic Health Records (EHRs) provides a continuous influx of real-time data, further refining treatment strategies and enabling proactive care. Nevertheless, the adoption of AI in personalized medicine introduces formidable legal and ethical challenges. Critical issues related to data privacy, security and equitable access must be meticulously addressed to fully unlock the transformative potential of these technologies. The establishment of robust regulatory frameworks and the provision of thorough training for healthcare professionals are essential to navigating these challenges and ensuring the judicious application of AI. As this field continues to evolve, the collaboration among researchers, developers, regulators and clinicians will be essential in shaping the future trajectory of personalized medicine. By achieving a balance between technological innovation and clinical expertise, we can usher in an era of healthcare that not only advances precision in treatment but also fosters a more equitable and effective healthcare system for all individuals.
Cite this article:
Nori LP, Lohitha M, Vadapalli RR, Bonthagarala B, Nagineni SR, Kalidindi VR. Revolutionizing Healthcare: The Impact of AI on Precision Medicine. Int. J. Pharm. Investigation. 2025;15(2):10-8.
ACKNOWLEDGEMENT
The authors are thankful to Shri Vishnu College of Pharmacy, Bhimavaram for providing the necessary facilities.
ABBREVIATIONS
PMI | Personalized Medicine Initiative |
---|---|
EHR | Electronic Health Records |
FDA | Food and Drug Administration |
NIH | National Institutes of Health |
HIPAA | Health Insurance Portability and Accountability Act |
GINA | Genetic Information Non-discrimination Act |
QPOP | Quadratic Phenotypic Optimization Platform |
HLA | Human Leukocyte Antigen |
TNBC | Triple-Negative Breast Cancer |
IBM | International Business Machines |
FDA | Food and Drug Administration |
References
- (2024) Driven personalized medicine: Assessing the impact of federal policies on advancing patient-centric care. Eur. Int. J Med Health Sci 6: 77-89 Google Scholar
- Blasiak A., Khong J., Kee T.. (2020) CURATE.AI: Optimizing personalized medicine with artificial intelligence. SLAS Technology 25: 95-105 Google Scholar
- Cernea A., Fernández-Martínez J. L., de Andrés-Galiana E. J., Fernández-Muñiz Z., Bermejo-Millo J. C., González-Blanco L., Solano J. J., Abizanda P., Coto-Montes A., Caballero B., et al. (2019) Prognostic networks for unraveling the biological mechanisms of sarcopenia. Mechanisms of Ageing and Development 182: 111129 Google Scholar
- Collins F. S., Varmus H.. (2015) A new initiative on precision medicine. The New England Journal of Medicine 372: 793-795 Google Scholar
- Davenport T., Kalakota R.. (2019) The potential for AI in healthcare. Future Healthcare Journal 6: 94-98 Google Scholar
- Ferrucci D., Brown E., Chu-Carroll J., Fan J., Gondek D., Kalyanpur A. A., Lally A., Murdock J. W., Nyberg E., Prager J., Schlaefer N., Welty C., et al. (2010) Building Watson: An overview of the DeepQA project. AI Magazine 31: 59-79 Google Scholar
- Gilvary C., Madhukar N., Elkhader J., Elemento O.. (2019) The missing pieces of artificial intelligence in medicine. Trends in Pharmacological Sciences 40: 555-564 Google Scholar
- Greenberg N., Docherty M., Gnanapragasam S., Wessely S.. (2020) Managing mental health challenges faced by healthcare workers during Covid-19 pandemic. BMJ 368: m1211 Google Scholar
- Gulshan V., Peng L., Coram M., Stumpe M. C., Wu D., Narayanaswamy A., Venugopalan S., Widner K., Madams T., Cuadros J., Kim R., Raman R., Nelson P. C., Mega J. L., Webster D. R., et al. (2016) Development and validation of a deep learning algorithm for detection of diabetic retinopathy in retinal fundus photographs. JAMA 316: 2402-2410 Google Scholar
- Holzinger A., Biemann C., Pattichis C. S., Kell D. B.. (2017) What do we need to build explainable AI systems for the medical domain?. Arxiv Google Scholar
- Howard F. M., Dolezal J., Kochanny S., Schulte J., Chen H., Heij L., et al. (2021) The impact of digital pathology and artificial intelligence on the accuracy of pathology diagnostics. JAMA Network Open 4 Google Scholar
- Ideker T., Dutkowski J., Hood L.. (2011) Boosting signal-to-noise in complex biology: Prior knowledge is power. Cell 144: 860-863 Google Scholar
- Jiang X., Zhang Y.. (2023) Artificial intelligence in personalized medicine: Current applications and prospects. Advanced Drug Delivery Reviews 182: 114-127 Google Scholar
- Johnson K. B., Wei W. Q., Weeraratne D., Frisse M. E., Misulis K., Rhee K., Snowdon J. L., et al. (2021) Personalised medicine, AI and the future of personalized health care. Clinical and Translational Science 14: 86-93 Google Scholar
- Jumper J., Evans R., Pritzel A., Green T., Figurnov M., Ronneberger O., Tunyasuvunakool K., Bates R., Žídek A., Potapenko A., Bridgland A., Meyer C., Kohl S. A. A., Ballard A. J., Cowie A., Romera-Paredes B., Nikolov S., Jain R., Adler J., Hassabis D., et al. (2021) Highly accurate protein structure prediction with AlphaFold. Nature 596: 583-589 Google Scholar
- Keerthana G., Revathi M. P., Ambika M., Usharani P.. (2020) Unlocking genetic insights: Exploring the potential of AI systems in gene analysis. NeuroQuantology 18: 224 Google Scholar
- Lin W., Ho D.. (2018) Quadratic phenotypic optimization platform (QPOP): A tool for quantitative modeling of phenotype-drug interactions. Nature Communications 9 Google Scholar
- Magrabi F., Ammenwerth E., McNair J. B., De Keizer N. F., Hyppönen H., Nykänen P., Rigby M., Scott P. J., Vehko T., Wong Z. S.-Y., Georgiou A., et al. (2019) Artificial intelligence in clinical decision support: Challenges for evaluating AI and practical implications. Yearbook of Medical Informatics 28: 128-134 Google Scholar
- Redekop W. K., Mladsi D.. (2013) The faces of personalized medicine: A framework for understanding its meaning and scope. Value in Health 16: S4-S9 Google Scholar
- Schmidt C.. (2017) Raising questions about AI in oncology. Journal of the National Cancer Institute 109: 4-5 Google Scholar
- Shortliffe E. H.. (2019) Artificial intelligence in medicine: Weighing the accomplishments, hype and promise. Yearbook of Medical Informatics 28: 257-262 Google Scholar
- Timmermans S., Berg M.. (2010) The gold standard: The challenge of evidence-based medicine and standardization in health care Google Scholar