ABSTRACT
Background
Uninterrupted spread of drug-resistant tuberculosis has necessitated prioritization of new and effective antitubercular compound. This investigation aimed to apply in silico methods to find out potential inhibitor to KatG protein which is the major cause of drug-resistance in Mycobacterium tuberculosis strains.
Materials and Methods
To understand and identify effective candidate as inhibitor, virtual screening of 2632 compounds of ZINC library, modelling of KatG and its docking with 3 best hit compounds were performed. ADMET analysis of best hit compounds and MD Simulation of KatG with lead compound were also executed.
Results
Molecular docking exhibited higher binding affinity (-11.3 Kcal/mol) for ZINC-65407173 hit compound indicating its strong binding with KatG. Analysis of MD Simulation indicated that deviations (2-3 stable bonds) and fluctuation (RMSF<0.5 nm) were minimal at ZINC-65407173 binding residues for KatG and this compound was identified as a new lead for KatG inhibition based on higher confidence score (0.997), higher drug score (0.994), drug-likeness (3.6), better interaction (ΔG=-22.64 kcal/mol), no toxicity risks, ADMET profiling, stable binding (RMSD=0.4 nm) and insignificant conformational change (SASA=320 nm2).
Conclusion
Experimental validation combined with clinical trials should be performed to determine the efficacy of this lead compound.
INTRODUCTION
Tuberculosis is the foundation of the 2nd major reason of human fatalities just after HIV which leads to ‘AIDS’ (World Health Organization, 2023). The persistent spread of anti-microbial resistant strains has reduced the number of usable anti-tubercular drugs (Soltanet al., 2021). Genetic research has denoted that resistance is the outcome of spontaneous point mutations, deletions and insertions in genes that are involved in drug inactivation (Alcocket al., 2020). Drug resistance to antimicrobials are divided into 4 categories (Reygaert, 2018). There are 3 virulence factors like number of enzyme coding genes, superfamilies of enzymes and active drug effiux systems which are involved in antimicrobial resistant activities of M. tuberculosis strains (Coleet al., 1998). The cell wall decreases permeability of antimicrobial agents (Bentley, 1997). The virulence factors encoded by a number of genes of pathogens, produce disease in host cells (Ruiz-Bacaet al., 2021) and these genes are related to virulence associated life style (Echeverria-Valenciaet al., 2018). These virulence factors rely upon its transmission across cell membrane of bacteria (Comaset al., 2010). Moreover, various transporter systems are involved in drug resistance (Poseyet al., 2006). The ‘KatG’ which is the most important amid all protective enzymes, detoxifies the toxic elements and neutralizes redox or oxidative stress (Trivediet al., 2012). MTB strains having mutations at codon 315 in ‘KatG’ gene persist in virulent form (Pymet al., 2002). Moreover, ‘KatG’ protein is actively connected with pathogenicity (DeVito and Morris, 2003). The in silico approaches, are designed on the basis of wet-lab based results and literature reports denoting its successful application (Barhet al., 2011). Now a days, bioinformatic approaches, have achieved remarkable importance in the area of potential drug target identification (Boeckmannet al., 2003) and development of promising drug compounds as they have brought down the costs and time needed for wet-lab investigation (Boeckmannet al., 2003; Linet al., 2020).
The present study has, been oriented to exploit various computational approaches like newly designed DNN (Mukul and Sarkar, 2022) including CodonW and Genetic Algorithms (G.A) for biological data extraction of various MDR-strains of M. tuberculosis. The subtractive genomics approaches, structure-based approaches, molecular dynamics simulation including ADMET properties analysis have been undertaken.
MATERIALS AND METHODS
The present study has been conducted to identify promising candidate for KatG inhibition in M. tb_DKC2 strain by employing advanced computational methods.
Prediction of drug target protein
The whole genome sequence of M. tb_DKC2 were retrieved from NCBI GenBank and submitted to CodonW followed by GA with Random Forest for data extraction. The DNN trained with genomic data of MTB strains was executed on dataset to predict essential proteins corresponding to essential genes followed by subtractive genomics methods for drug target identification.
Molecular docking
Preparation of 3D-modelled protein
The katG protein was predicted as drug target by retrieval of essential proteins from NCBI GenBank and it was submitted to SWISS MODEL (Waterhouseet al., 2018) for homology model. PROCHECK, PSIPRED, PROSA and ERRAT Computational tools were used for validation of katG protein.
Identification of modelled protein active site
The active binding site with co-crystalized ligand (HEME) of template protein was identified by Biovia Discovery Studio Visualizer (BIOVIAiovia, 2017). A ‘Grid Box’ was constructed on the same active binding site using 86.4388, 37.3258 and 50.228 co-ordinates of center pointing with a size of 18.3818Å, 15.9234Å and 22.0152Å in X, Y and Z directions, respectively. The ‘Grid Box’ with same parameters was formed on KatG modelled protein through virtual screening using UCSF Chimera v 1.12 user interface to predict active binding site and the same was confirmed by using DoGSiteScorer (Volkameret al., 2012).
HEME-Ligand identification
The naturally occurring ligand ‘HEME’ found within the active binding site of template protein (2CCD) was retrieved from RCSB PDB for validation of docking parameters of Autodock Vina by redocking of template protein and the validated Autodock Vina parameters was used during docking of modelled protein with ligands of ‘ZINC’ database.
Virtual screening of ligands of ZINC database
The modelled protein prepared by computing Gasteiger charges and adding polar hydrogens, was used to perform virtual screening against compounds (ligands) obtained from ‘ZINC’ database using Autodock Vina with validated docking parameters. Eventually, best possible ZINC compounds were obtained.
Protein and ligand preparation for molecular docking analysis
Molecular docking analysis
Autodock Vina v 1.12 (Tanchuket al., 2016; Gaillard, 2018) was executed for molecular docking of KatG (PDB ID: 2CCD) with its ‘HEME’ ligand. An iterated Local Search global optimization algorithm based on BFGS method was executed in docking study and UCSF Chimera v 1.12 was used for various orientations and conformations of ‘HEME’ ligand binding in active site of template. The lowest binding (-16.8 kcal/mol) score was selected which indicated stable binding of ‘HEME’ ligand. The modelled protein was then docked with prepared ZINC ligands using same parameters which were used for AutoDock validation. Altogether 3 hit compounds were identified as drug like candidates and ranked as per their binding energies to verify the ligand binding site. The post docking analysis of 3 hit compounds was performed using BIOVIA Discovery Studio Visualizer v 4.5 (BIOVIAiovia, 2017).
Prediction of Drug-likeness and ADMET parameters
The prediction of ADMET properties of 3 selected compounds (ZINC-65407173, ZINC-09849203 and ZINC-09349296) was performed using SWISS ADME (Dainaet al., 2017) and pkCSM (Pireset al., 2015) computational tools (Table S2 and S3) while OSIRIS property explorer (Sander, 2001; Oladejoet al., 2023) was executed to ascertain drug-likeness and other properties of the same drug like compounds (Table 2).
Molecular Dynamics (MD) Simulation
Both protein-HEME and protein-ZINC65407173 complexes were subjected to MD Simulation using GROMACS 2021.4 software (Hesset al., 2008). The pdb2gmx module of GROMACS and SWISS-PARAM (Zoeteet al., 2011) were used to built topology of protein and ligands, respectively. CHARMM v 27 (Bjelkmaret al., 2010) was assigned to protein and ligands for intramolecular interactions. The long range coulombic electrostatic force was treated by PME algorithm. The neutralization of charges was performed by adding Na+ and Cl– ions. The solvation of both complexes was performed using TIP3P water cube model. The steepest descent method was used to minimize energy. The system was set up maintaining NVT and simulation was performed for 100ps at constant 300k using modified Berendsen Thermostat algorithm. NPT ensemble was performed to stabilize pressure at 1 atm for 100ps employing Parrinello-Rahman and Berendsen algorithms. Finally, simulation was performed at both constant pressure and temperature of 1 bar and 310K, respectively employing time step of 2fs. H-bonds were constrained with LINCS algorithm and 60ns simulation was generated by recording coordinate trajectories at every 10ps. The graphs were plotted using XMGrace software (Turner, 2005). The trajectory files were analysed by HeroMDAnalysis tool (Rawatet al., 2021) to calculate change in Gibb’s free energy of binding for protein-ligand complexes.
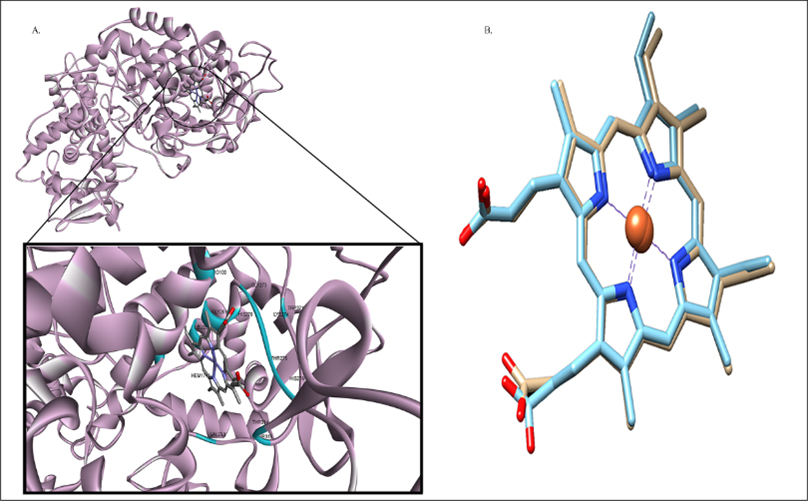
Figure 1:
A. Predicted binding of Catalase-peroxidase: Displayed by Pro100(3.270Å), Gly273(3.316 Å), Gly269(3.417Å), His270(2.452Å), Lys274(3.228Å), Trp321(3.384Å), Thr275(3.262Å), Leu265(3.215Å), His276(2.501Å), Val230(3.363Å), Thr314(3.259Å), Thr315(2.905Å), residues. B. The superimposed docked complex of pre-docked ‘HEME’ (Sky Blue) over post-docked (Khaki) depicting the validation of docking study in terms of RMSD value indicated as 0.525Å.
RESULTS
The most active binding site of KatG was selected using ‘HEME’ ligand in order to perceive functions of protein and the active binding site was further confirmed using ‘DoGSiteScorer’ (Figure 1). RMSD value>2.0 Å justified suitable method for re-creation of binding pose (Shoichetet al., 2002).
The highest simple score (0.63) predicted by DoGSiteScorer, confirmed the most active binding site of target protein amid 26 druggable binding sites for the purpose of docking analysis (Uddin and Rafi, 2017). Molecular docking analysis of 2632 druglike compounds was performed to ascertain potential lead compound against KatG (Figure 2). Three (3) best hit compounds viz. ZINC65407173, ZINC09849203 and ZINC09349296 with binding energy of -11.3, -10.3 and -10.9 Kcal/mol., respectively (Table 1) were identified by Autodock Vina 1.1.2.
Hit compounds retained substantial interactions like H-bonding, Van der Waals interactions, Pi-Pi stacking, Pi-Sigma interactions (Figure 3) which may have important role in inhibition of KatG (Oladejoet al., 2023). Highest confidence score of 0.997, was generated for ZINC65407173 (Table S1) The docking interactions was more in respect of ZINC65407173 RMSD value of 0.525Å as output of re-docking validated the virtual screening of hit compounds, ADME profiling (Table S2) and Toxicity properties (Table S3) of hit compounds were analysed.
The toxicity risks, drug likeness, topological polar surface area (Å), drug score and ClogP values of 3 drug like compounds (Table 2) were investigated using ‘OSIRIS property explorer tool’ (Sander, 2001). MD simulation of two docked complexes viz HEME-KatG and ZINC65407173-KatG as well as virtual drug screening were performed to identify potent inhibitor which may be used as antitubercular compound. The graphs of Figure 4 represented molecules A) HEME and B) ZINC65407173 bound inside the active site of modelled protein KatG which was displayed in ‘Cartoon’ and ligands were shown in ‘CPK’ (Corey-Paulin-Koltun) representation (Corey and Pauling, 1953; Koltun, 1965). The equilibrium ‘RMSD’ value for both protein complexes stood around 0.4nm. The effect of ‘HEME’ and ZINC65407173 ligands conformational changes on KatG was observed from the fluctuation in residues as shown in ‘RMSD’, ‘RMSF’, ‘Radius of gyration’ (Rg), ‘SASA’ and ‘Free energy of solvation’ plots (Figure 5).
The SASA plot displayed similar results (320 nm2 as average value) for packing distribution of residues in respect of both HEME-protein and ZINC65407173-protein complexes and it showed insignificant conformational changes of complexes.
The average ‘Rg’ value of ZINC65407173 and HEME were recorded as 2.8 nm and 2.9 nm, respectively.
KatG-HEME and KatG-ZINC65407173 complexes were also analysed based on H-bonds % occupancy (Figure 6) during MD simulation. In respect of ZINC65407173 ligand, amino acid residues namely TRP82 and HIS245 were found common with ‘HEME’ active site after screening of (%) occupancy. The interaction of ZINC65407173 with TRP82 and HIS245 residues were stable for 3.2 and 64.60% duration of simulation, respectively while the interaction of ‘HEME’ ligand with TRP82 and HIS245 residues were for 2.59 and 24.13% duration, respectively. The interaction of ‘HEME’ ligand with Lys249 residue in the binding loop between protein and HEME was most stable for 70.18% duration of simulation. The other amino acid residues involved in stable binding included TRP82, LYS188, HIS245, HIS251, THR289, SER290 and LYS532.
Sl. No. | Ligand | Receptor | Interaction | Distance(Å) | Binding Energy (Kcal/Mol) |
---|---|---|---|---|---|
1. | ZINC65407173 | ||||
O2 | HE:ARG104 | H-bond | 2.448 | -11.3 | |
O2 | HH21:ARG104 | H-bond | 2.571 | ||
HN7 | NE2:HIS270 | H-bond | 2.785 | ||
6-Ring | TRP107 | Pi-Pi Stacked | 4.65 | ||
6-Ring | TRP107 | Pi-Pi Stacked | 3.61 | ||
6-Ring | TRP107 | Pi-Pi Stacked | 3.94 | ||
6-Ring | TRP107 | Pi-Pi Stacked | 4.49 | ||
2. | ZINC09849203 | ||||
O3 | LYS274:H | H-bond | 2.307 | -10.5 | |
H5 | PRO100:O | H-bond | 2.58 | ||
H5 | PHE272:O | H-bond | 3.04 | ||
6-Ring | ARG104:HB2 | Pi-Sigma | 2.71 | ||
3. | ZINC09349296 | ||||
HN4 | HIS270:NE2 | H-bond | 2.28 | -10.9 | |
6-Ring | TRP107 | Pi-Pi Stacked | 3.68 | ||
6-Ring | TRP107 | Pi-Pi Stacked | 3.83 | ||
6-Ring | TRP107 | Pi-Pi Stacked | 4.18 | ||
6-Ring | TRP107 | Pi-Pi Stacked | 4.21 |
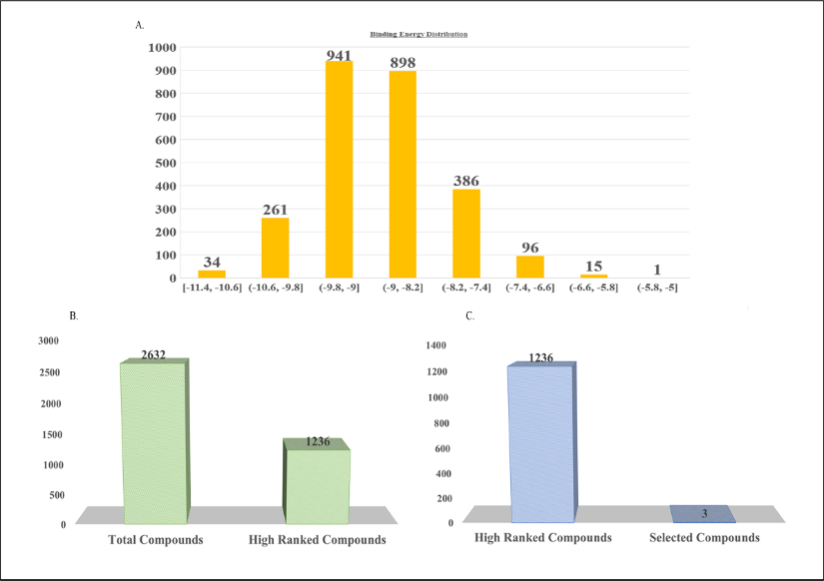
Figure 2:
A. Virtual screening of 2632 compounds. B. Identified leads like compounds. C. proposed 3 lead compounds.
The increased average ΔG binding free energy (Table 3 and Figure 5F) in case of ‘HEME’ and ZINC65407173 were reported as -40.13 Kcal/mol and -22.64 Kcal/mol, respectively which denoted their ability to interact better with modelled protein i.e. KatG protein rather than the initial docked pose.
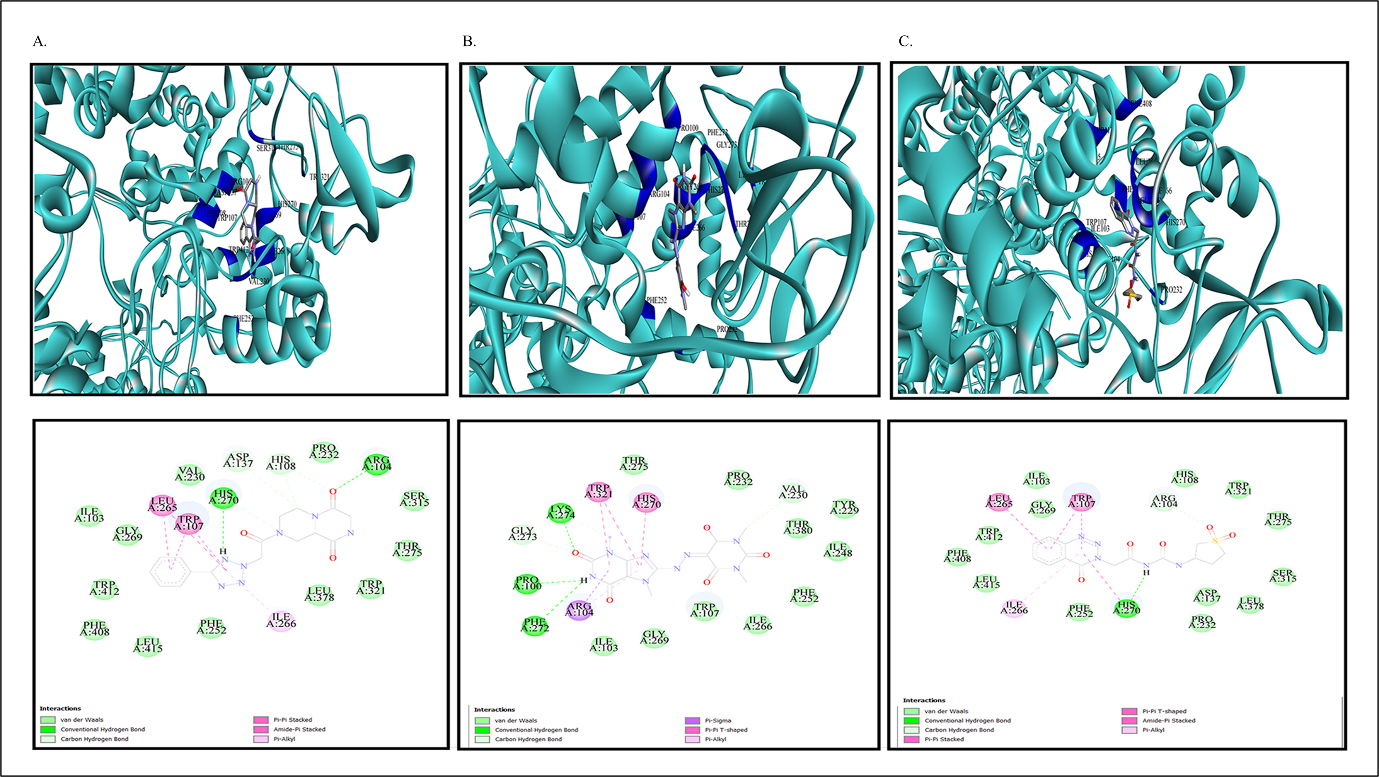
Figure 3:
Docked compounds incorporating modelled protein: A. For ZINC65407173, B. For ZINC09849203. C. For ZINC09349296.
Proposed potential drug compounds | TPSA(Å) | Mutagenic | Tumorigenic | Irritant | Reproduction | Drug Score(ds) | CLogP | Drug-likeness |
---|---|---|---|---|---|---|---|---|
ZINC65407173 | 113.3 | No | No | No | No | 0.904 | -0.81 | 3.6 |
ZINC09849203 | 149.3 | No | No | No | Yes | 0.542 | -0.02 | -3.59 |
ZINC09349296 | 145.7 | Yes | Yes | No | No | 0.159 | -0.79 | 5.73 |
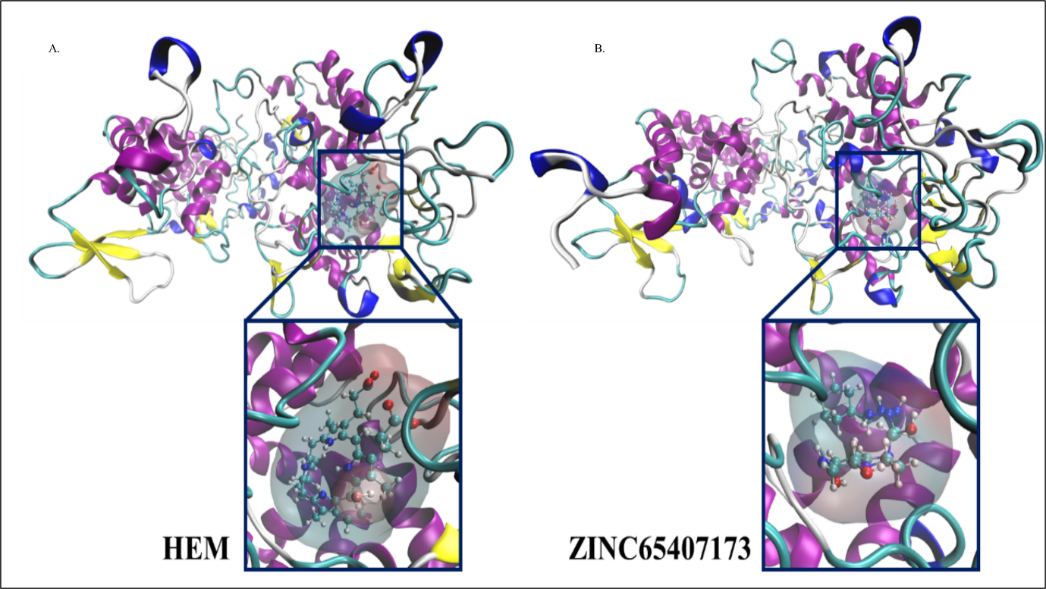
Figure 4:
Graphical representation of molecules A. HEME and B. ZINC65407173 bound inside active site of modelled protein where protein is shown in cartoon representation and ligand is shown in CPK representation.
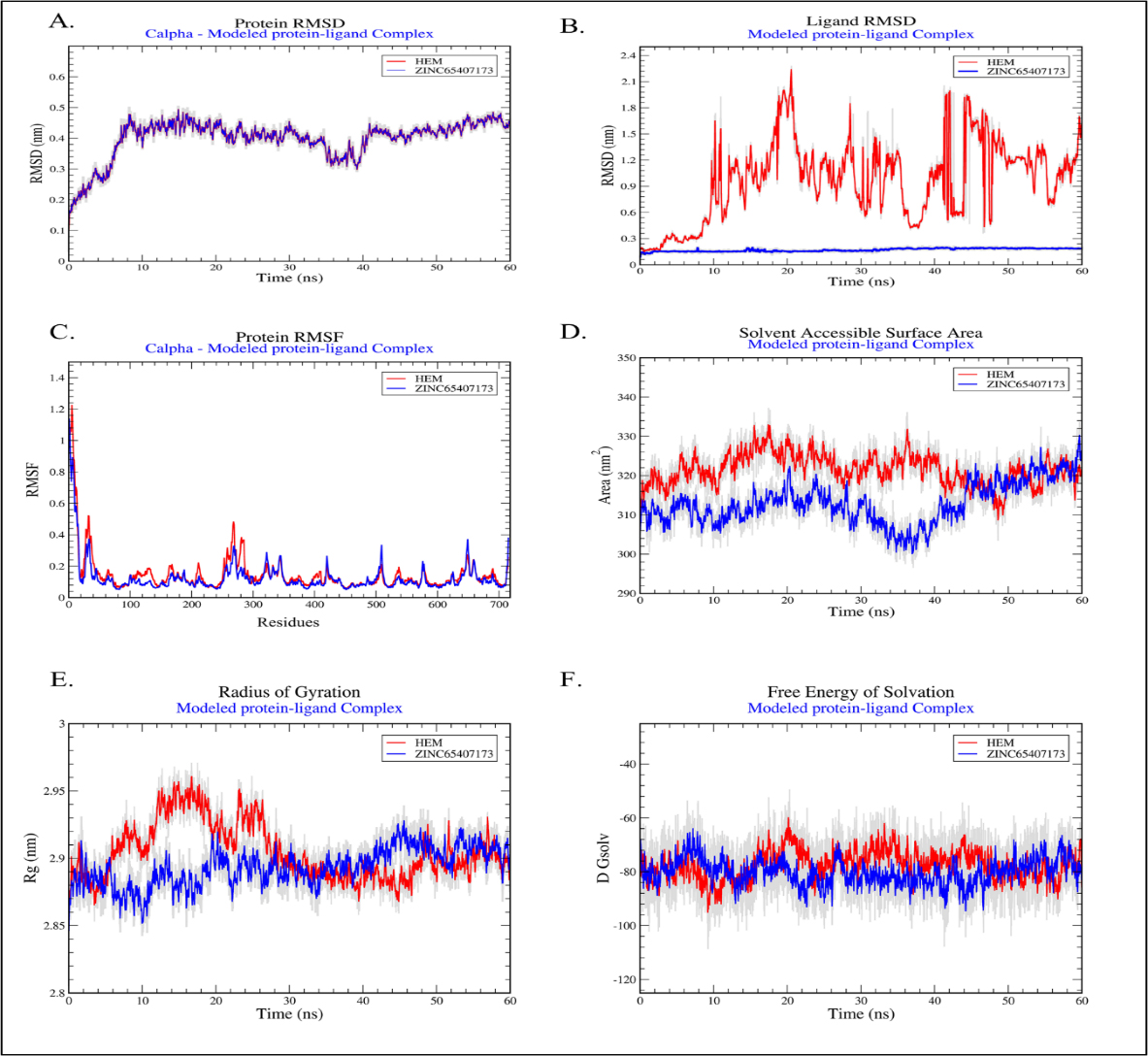
Figure 5:
Graphical representation of plots: A. Protein RMSD, B. Ligand RMSD, C. Protein RMSF, D. Solvent Accessible Surface Area, E. Radius of Gyration and F. Free Energy of Solvation.
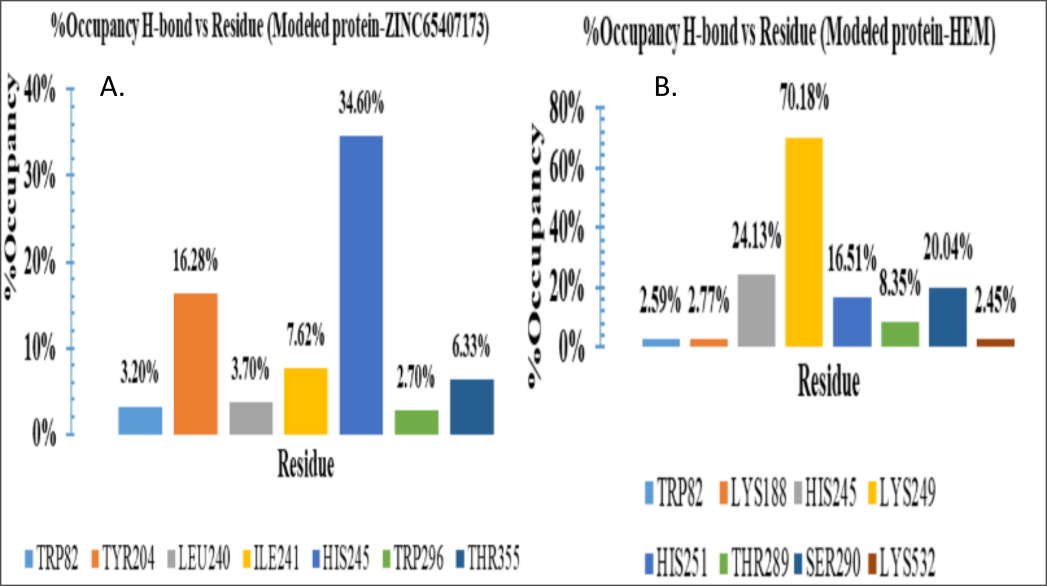
Figure 6:
Percent (%) Occupancies of h-bond protein-ligand contacts: A. Modelled protein-HEME complex, B. Modelled protein-ZINC65407173 complex.
DISCUSSION
In modern era, computational approaches have achieved appreciable attention in the field of identification of drug target and drug candidates (Boeckmannet al., 2003; Linet al., 2020). In the present study, 2682 compounds of ZINC library were screened against KatG protein to identify promising drug candidates. Eventually, ZINC-65407173, ZINC-09849203 and ZINC-09349296 were shortlisted as drug-like molecules based on docking analysis. ADMET (Absorption, Distribution, Metabolism, Excretion and Toxicity) analysis for 3 drug-like molecules were performed. MD simulation was executed to investigate stability of binding conformation and mode of residual interactions between ZINC-65407173 compound and KatG at active site. The predicted results were discussed in this section. It was revealed from investigation (Table 2, Tables S2 and S3) that ZINC-65407173 compound having molecular weight<500 Da and solubility value of -2.182 is soluble in water and it might reach to active site. The Blood-Brain-Barrier (BBB) value (-0.837>-1) justified that ZINC65407173 was poorly permeable and it can’t cross BBB (Tamai and Tsuji, 2000). CYP4501A2 metabolizing enzymes (Di, 2014) is not inhibited by this compound. The CaCO-2 permeability value of 0.18, % value of HIA (65.379), skin permeability value (-2.822) and Biochemical PAINS with no false positive result for ZINC-65407173 compound indicated that this compound has good oral doping (Rasulev and Casañola-Martin, 2018), good intestinal absorption (Pireset al., 2015), high permeability (Potts and Guy, 1992) and no inhibiting property against Renal Organic Cation transporter protein. Compound ZINC-65407173 possesses ‘ds’ value of 0.904, MRTD value of 0.187 log (<0.477) (Stampferet al., 2019), Minnow toxicity (Chenet al., 2020), T. pyriformis toxicity (Yoshioka et al., 1985), Oral rat acute toxicity and hepatotoxicity but this compound is Ames negative (Vijayet al., 2018) and does not show skin sensitization. Lowest CLogP and Druglikeness values denote that ZINC-65407173 is suitable drug-like compound within no-risk range. TPSA value of 113.3 Å (<140 Å) indicates that this compound does not possess poor cell permeability (Gregson and Plowe, 2005). The stability and conformation of protein and ligand complexes were verified employing RMSD, RMSF, Rg, SASA and % occupancies of H-bonds in protein-ligand complexes during simulation. The RMSD value of 0.4 nm denoted that both HEME-KatG and ZINC65407173-KatG complexes were stable. RMSF showed similar residual fluctuation (<0.5 nm) of lower magnitude for both complexes. SASA plot did not show any occurrence of unfolding or folding incident. H-bonds % occupancy also indicated stable interactions between ‘ZINC-65407173’ compound and ‘HIS-245’ residues for 64.60% duration of simulation while interactions of ‘HEME’ with ‘HIS245’ residues were 24.13% duration. The ‘Rg’ measurement denoted a similarity in protein stability and compactness between KatG-HEME and KatG-ZINC-65407173 (Srinivasanet al., 2021). The good binding affinity, minimal deviation in simulation and decent ADMET properties including drug score with no toxicity risk observed for ZINC-65407173 indicated that compound ZINC-65407173 (C16H17N7O3) is a potential candidate for KatG inhibition.
Sl. No. | Ligand | ΔG values (kcal/mol) | Average ΔG value | |
---|---|---|---|---|
1. | HEM | 0 ns | -22.48 | -40.13 kcal/mol |
30 ns | -47.27 | |||
60 ns | -50.64 | |||
2. | ZINC65407173 | 0 ns | -6.73 | -22.64 kcal/mol |
30 ns | -23.89 | |||
60 ns | -37.28 |
CONCLUSION
The wet lab studies and clinical trials coupled with this in silico approaches are required to be performed to evaluate the reported activities of Compound No.: ZINC65407173 as promising lead against various strains of M. tuberculosis including M. tb_DKC2 strain.
Cite this article:
Das MM, Chakraborty S. Identification of a Potential Compound against Drug Resistant Strain of Mycobacterium tuberculosis Using in silico Methods. Int. J. Pharm. Investigation. 2025;15(2):10-8.
REFERENCES
- Alcock, B. P., Raphenya, A. R., Lau, T. T. Y., Tsang, K. K., Bouchard, M., Edalatmand, A., Huynh, W., Nguyen, A. V., Cheng, A. A., Liu, S., Min, S. Y., Miroshnichenko, A., Tran, H.-K., Werfalli, R. E., Nasir, J. A., Oloni, M., Speicher, D. J., Florescu, A., Singh, B., . . . McArthur, A. G. (2020). CARD 2020: Antibiotic resistome surveillance with the comprehensive antibiotic resistance database. Nucleic Acids Research, 48(D1), D517–D525. https://doi.org/10.1093/nar/gkz935
- Barh, D., Tiwari, S., Jain, N., Ali, A., Santos, A. R., Misra, A. N., Azevedo, V., & Kumar, A. (2011). In silico subtractive genomics for target identification in human bacterial pathogens. Drug Development Research, 72(2), 162–177. https://doi.org/10.1002/ddr.20413
- Bentley, P. (1997). Anti-infectives. Recent advances in chemistry and structure–activity relationships. Royal Society of Chemistry Special Publication, 198.
- Biovia, D. S. (2017). BIOVIA discovery studio visualizer. Software version, 20, 779.
- Bjelkmar, P., Larsson, P., Cuendet, M. A., Hess, B., & Lindahl, E. (2010). Implementation of the CHARMM force field in GROMACS: Analysis of protein stability effects from correction maps, virtual interaction sites and water models. Journal of Chemical Theory and Computation, 6(2), 459–466. https://doi.org/10.1021/ct900549r
- Boeckmann, B., Bairoch, A., Apweiler, R., Blatter, M.-C., Estreicher, A., Gasteiger, E., Martin, M. J., Michoud, K., O’Donovan, C., Phan, I., Pilbout, S., & Schneider, M. (2003). The Swiss-PROT protein knowledgebase and its supplement TrEMBL in 2003. Nucleic Acids Research, 31(1), 365–370. https://doi.org/10.1093/nar/gkg095
- Butt, S. S., Badshah, Y., Shabbir, M., & Rafiq, M. (2020). Molecular docking using chimera and autodock vina software for nonbioinformaticians. JMIR Bioinformatics and Biotechnology, 1(1), Article e14232. https://doi.org/10.2196/14232
- Chen, X., Dang, L., Yang, H., Huang, X., & Yu, X. (2020). Machine learning-based prediction of toxicity of organic compounds towards fathead minnow. RSC Advances, 10(59), 36174–36180. https://doi.org/10.1039/d0ra05906d
- Cole, S., Brosch, R., Parkhill, J., Garnier, T., Churcher, C., Harris, D. et al. (1998). Deciphering the biology of Mycobacterium tuberculosis from the complete genome sequence. Nature, 396(6707), 190–.
- Comas, I., Chakravartti, J., Small, P. M., Galagan, J., Niemann, S., Kremer, K., Ernst, J. D., & Gagneux, S. (2010). Human T cell epitopes of Mycobacterium tuberculosis are evolutionarily hyperconserved. Nature Genetics, 42(6), 498–503. https://doi.org/10.1038/ng.590
- Corey, R. B., & Pauling, L. (1953). Molecular models of amino acids, peptides and proteins. Review of Scientific Instruments, 24(8), 621–627. https://doi.org/10.1063/1.1770803
- Daina, A., Michielin, O., & Zoete, V. (2017). SwissADME: A free web tool to evaluate pharmacokinetics, drug-likeness and medicinal chemistry friendliness of small molecules. Scientific Reports, 7(1), Article 42717. https://doi.org/10.1038/srep42717
- DeVito, J. A., & Morris, S. (2003). Exploring the structure and function of the mycobacterial KatG protein using trans-dominant mutants. Antimicrobial Agents and Chemotherapy, 47(1), 188–195. https://doi.org/10.1128/AAC.47.1.188-195.2003
- Di, L. (2014). The role of drug metabolizing enzymes in clearance. Expert Opinion on Drug Metabolism and Toxicology, 10(3), 379–393. https://doi.org/10.1517/17425255.2014.876006
- Echeverria-Valencia, G., Flores-Villalva, S., & Espitia, C. I. (2018). Virulence factors and pathogenicity of Mycobacterium. Mycobacterium-Research and Development. InTech, 231–255.
- Gaillard, T. (2018). Evaluation of AutoDock and AutoDock Vina on the CASF-2013 benchmark. Journal of Chemical Information and Modeling, 58(8), 1697–1706. https://doi.org/10.1021/acs.jcim.8b00312
- Gregson, A., & Plowe, C. V. (2005). Mechanisms of resistance of malaria parasites to antifolates. Pharmacological Reviews, 57(1), 117–145. https://doi.org/10.1124/pr.57.1.4
- Hess, B., Kutzner, C., Van Der Spoel, D., Lindahl, E., & GROMACS. (2008). GROMACS 4: Algorithms for Highly Efficient, Load-Balanced, and Scalable Molecular Simulation. Journal of Chemical Theory and Computation, 4(3), 435–447. https://doi.org/10.1021/ct700301q
- Koltun, W. L. (1965). Precision space-filling atomic models. Biopolymers, 3(6), 665–679. https://doi.org/10.1002/bip.360030606
- Lin, X., Li, X., & Lin, X. (2020). A review on applications of computational methods in drug screening and design. Molecules, 25(6), 1375. https://doi.org/10.3390/molecules25061375
- Mukul Das, M. M., & Sarkar, K. (2022). Evaluation of machine learning classifiers for predicting essential genes in Mycobacterium tuberculosis strains. Bioinformation, 18(12), 1126–1130. https://doi.org/10.6026/973206300181126
- Oladejo, D. O., Duselu, G. O., Dokunmu, T. M., Isewon, I., Oyelade, J., Okafor, E., Iweala, E. E., & Adebiyi, E. (2023). In silico Structure Prediction, Molecular Docking and Dynamic Simulation of Plasmodium falciparum AP2-I transcription factor. Bioinformatics and Biology Insights, 17, Article 11779322221149616. https://doi.org/10.1177/11779322221149616
- Pires, D. E. V., Blundell, T. L., & Ascher, D. B. (2015). pkCSM: Predicting small-molecule pharmacokinetic and toxicity properties using graph-based signatures. Journal of Medicinal Chemistry, 58(9), 4066–4072. https://doi.org/10.1021/acs.jmedchem.5b00104
- Posey, J. E., Shinnick, T. M., & Quinn, F. D. (2006). Characterization of the twin-arginine translocase secretion system of Mycobacterium smegmatis. Journal of Bacteriology, 188(4), 1332–1340. https://doi.org/10.1128/JB.188.4.1332-1340.2006
- Potts, R. O., & Guy, R. H. (1992). Predicting skin permeability. Pharmaceutical Research, 9(5), 663–669. https://doi.org/10.1023/a:1015810312465
- Pym, A. S., Saint-Joanis, B., & Cole, S. T. (2002). Effect of katG mutations on the virulence of Mycobacterium tuberculosis and the implication for transmission in humans. Infection and Immunity, 70(9), 4955–4960. https://doi.org/10.1128/IAI.70.9.4955-4960.2002
- Rasulev, H. L.-T.-T., & Casañola-Martin, G. (2018). In silico assessment of ADME properties: Advances in Caco-2 Cell Permeability Modeling. Current Topics in Medicinal Chemistry, 20:000-.
- Rawat, R., Kant, K., Kumar, A., Bhati, K., & Verma, S. M. (2021). HeroMDAnalysis: An automagical tool for GROMACS-based molecular dynamics simulation analysis. Future Medicinal Chemistry, 13(5), 447–456. https://doi.org/10.4155/fmc-2020-0191
- Reygaert, W. C. (2018). An overview of the antimicrobial resistance mechanisms of bacteria. AIMS Microbiology, 4(3), 482–501. https://doi.org/10.3934/microbiol.2018.3.482
- Ruiz-Baca, E., Pérez-Torres, A., Romo-Lozano, Y., Cervantes-García, D., Alba-Fierro, C. A., Ventura-Juárez, J., & Torriello, C. (2021). The role of macrophages in the host’s defense against Sporothrix schenckii. Pathogens, 10(7), 905. https://doi.org/10.3390/pathogens10070905
- Sander, T. (2001):1099-0690. OSIRIS property explorer. Organic Chemistry Portal.
- Shoichet, B. K., McGovern, S. L., Wei, B., & Irwin, J. J. (2002). Lead discovery using molecular docking. Current Opinion in Chemical Biology, 6(4), 439–446. https://doi.org/10.1016/s1367-5931(02)00339-3
- Soltan, M. A., Elbassiouny, N., Gamal, H., Elkaeed, E. B., Eid, R. A., Eldeen, M. A., & Al-Karmalawy, A. A. (2021). In silico prediction of a multitope vaccine against Moraxella catarrhalis: Reverse vaccinology and immunoinformatics. Vaccines, 9(6), 669. https://doi.org/10.3390/vaccines9060669
- Srinivasan, E., Chandrasekhar, G., Chandrasekar, P., Anbarasu, K., Vickram, A. S., Tayubi, I. A., Rajasekaran, R., & Karunakaran, R. (2021). Decoding conformational imprint of convoluted molecular interactions between prenylflavonoids and aggregated amyloid-Beta42 peptide causing Alzheimer’s disease. Frontiers in Chemistry, 9, Article 753146. https://doi.org/10.3389/fchem.2021.753146
- Stampfer, H. G., Gabb, G. M., & Dimmitt, S. B. (2019). Why maximum tolerated dose? British Journal of Clinical Pharmacology, 85(10), 2213–2217. https://doi.org/10.1111/bcp.14032
- Tamai, I., & Tsuji, A. (2000). Transporter-mediated permeation of drugs across the blood–brain barrier. Journal of Pharmaceutical Sciences, 89(11), 1371–1388. https://doi.org/10.1002/1520-6017(200011)89:11<1371::aid-jps1>3.0.co;2-d
- Tanchuk, V. Y., Tanin, V. O., Vovk, A. I., & Poda, G. (2016). A new, improved hybrid scoring function for molecular docking and scoring based on AutoDock and AutoDock Vina. Chemical Biology and Drug Design, 87(4), 618–625. https://doi.org/10.1111/cbdd.12697
- Trivedi, A., Singh, N., Bhat, S. A., Gupta, P., & Kumar, A. (2012). Redox biology of tuberculosis pathogenesis. Advances in Microbial Physiology, 60, 263–324. https://doi.org/10.1016/B978-0-12-398264-3.00004-8
- Uddin, R., & Rafi, S. (2017). Structural and functional characterization of a unique hypothetical protein (WP_003901628.1) of Mycobacterium tuberculosis: A computational approach. Medicinal Chemistry Research, 26(5), 1029–1041. https://doi.org/10.1007/s00044-017-1822-0
- Vijay, U., Gupta, S., Mathur, P., Suravajhala, P., & Bhatnagar, P. (2018). Microbial mutagenicity assay: Ames test. Bio-Protocol, 8(6), Article e2763. https://doi.org/10.21769/BioProtoc.2763
- Volkamer, A., Kuhn, D., Rippmann, F., & Rarey, M. (2012). DoGSiteScorer: A web server for automatic binding site prediction, analysis and druggability assessment. Bioinformatics, 28(15), 2074–2075. https://doi.org/10.1093/bioinformatics/bts310
- Waterhouse, A., Bertoni, M., Bienert, S., Studer, G., Tauriello, G., Gumienny, R., Heer, F. T., de Beer, T. A. P., Rempfer, C., Bordoli, L., Lepore, R., & Schwede, T. (2018). Swiss-MODEL: Homology modelling of protein structures and complexes. Nucleic Acids Research, 46(W1), W296–W303. https://doi.org/10.1093/nar/gky427
- World Health Organization. (2023). (Tuberculosis report). Tuberculosis. World Health Organization. https://www.who.int/en/news-room/fact-sheets/detail/tuberculosis
- Xmgrace, T. P. (version 5.1), 19. Center for Coastal and Land-Margin Research, Oregon Graduate Institute of Science and Technology. (2005) p. 2.
- Yoshioka, Y., Ose, Y., & Sato, T. (1985). Testing for the toxicity of chemicals with Tetrahymena pyriformis. The Science of the Total Environment, 43(1–2), 149–157. https://doi.org/10.1016/0048-9697(85)90037-3
- Zoete, V., Cuendet, M. A., Grosdidier, A., & Michielin, O. (2011). SwissParam: A fast force field generation tool for small organic molecules. Journal of Computational Chemistry, 32(11), 2359–2368. https://doi.org/10.1002/jcc.21816