ABSTRACT
This review article explores the transformative impact of Artificial Intelligence (AI) and Machine Learning (ML) in cancer research. It focuses on the critical role of AI in medical imaging for cancer detection, exploring deep learning algorithms for image recognition and feature extraction. The study also examines the challenges and considerations in implementing AI for image analysis in cancer diagnosis. AI is also utilized for mining and analyzing large-scale omics data, such as genomics and proteomics. It is used to decipher complex genetic mutations and signaling pathways in cancer, integrating clinical and molecular data for more accurate diagnosis and treatment planning. The review also discusses AI applications in drug discovery, target identification and drug repurposing for cancer. AI-driven algorithms are used to predict drug responses and identify novel therapeutic targets, with case studies illustrating successful applications. The review evaluates the impact of AI on clinical decision-making and patient care, highlighting the challenges and opportunities in translating AI research into clinical practice. The review concludes with future directions and innovations, exploring emerging trends and potential advancements in AI-driven cancer research.
INTRODUCTION
Cancer, being a complex and adaptive process, remains a formidable challenge for patients, researchers and clinicians, despite significant strides in understanding its biological aspects. Managing cancer poses dilemmas at various stages, including early detection, distinguishing preneoplastic and neoplastic lesions, determining tumor margins during surgery, tracking tumor evolution and treatment resistance and predicting aggressiveness, metastasis and recurrence. The growing role of Artificial Intelligence (AI), Deep Learning (DL) and Machine Learning (ML) is significantly transforming healthcare industries.1,2 The AI and ML algorithms can analyse vast amounts of medical data, including patient records, images and genetic information to assist in diagnosing diseases more accurately and at an earlier stage. Rapid evolution of health digitalisation through AI is impacting the field of cancer significantly. Predictive analysis can help in identifying potential health risks and diseases, allowing for proactive and preventive interventions.3 Further, Natural Language Processing (NLP) and Large Language Models (LLMs) emerge as a distinctive category of AI algorithms. These sophisticated models harness the power of deep learning techniques and extensive datasets to comprehend, synthesize, create and predict new text-based content.4 Specifically designed for text generation, LLMs exhibit remarkable versatility in handling a spectrum of NLP tasks, ranging from translation, content summarization, rewriting, classification, categorization, to sentiment analysis. NLP, a specialized field within AI, revolves around the intricate interaction between computers and humans using natural language. It encompasses a wide array of techniques such as text mining, sentiment analysis, speech recognition and machine translation. The evolutionary trajectory of AI is noteworthy, progressing from its early stages rooted in rule-based systems to the contemporary landscape dominated by machine learning and deep learning algorithms. The expanding landscape of cancer research is marked by a continual influx of algorithms harnessing the capabilities of machine learning and brain-inspired methodologies and a large number of studies in AI are predominantly focused on classification and prediction.5 These algorithms play a pivotal role in deciphering intricate patterns within vast datasets, aiding in the identification of novel insights and predictive models. By leveraging learning and brain-based principles, these algorithms contribute to advancements in cancer diagnostics, prognostics and treatment strategies. The dynamic interplay between computational algorithms and biological understanding has the potential to unravel complex tumor behaviors and guide more targeted therapeutic interventions.
AI enables the development of personalized treatment plans based on individual patient data, genetic information and responses to specific interventions. It further can analyse real-time data, providing timely insights into a patient’s health status and alerting healthcare providers to potential issues.6,7 AI also accelerates the drug discovery process by analysing biological data, identifying potential drug candidates and predicting their effectiveness.8–10 Providing workflow optimization, AI streamlines administrative tasks, such as appointment scheduling, billing and medical coding, reducing administrative burdens on healthcare professionals. Automation of routine tasks allows healthcare providers to focus more on patient care and complex decision-making. AI-powered image recognition helps in interpreting medical imaging, such as X-rays, MRIs and CT scans, assisting radiologists in detecting abnormalities.11,12 Virtual assistants driven by AI provide information, schedule appointments and answer common medical queries, enhancing patient engagement. Telemedicine platforms use AI for remote consultations,13 making healthcare more accessible and convenient for patients. This review discusses the significant strides that AI has made in the field of cancer imaging and detection, data interpretation, prediction of patient outcomes, drug discovery and development particularly in the cancer research. It further delves into the challenges in the integration of AI into cancer research workflows. Emerging trends and future directions in AI-driven cancer research is also explored.
AI in precision oncology
Precision Oncology (PO), also known as personalized or individualized oncology, is the molecular profiling of tumors to identify targetable alterations. It is an approach to cancer treatment and care that takes into account the unique characteristics of each patient and their cancer. The goal of precision oncology is to tailor medical interventions, such as therapies and treatment plans, based on the specific genetic, molecular and clinical profile of an individual’s cancer.14 This approach recognizes that cancers can differ significantly between patients, even if they share the same traditional diagnostic label and aims to provide more targeted and effective treatments.
Precision oncology utilizes advanced technologies, such as genomic sequencing and molecular profiling, to analyze the genetic and molecular makeup of a patient’s tumor.15,16 By identifying specific alterations or mutations driving the growth of the cancer cells, oncologists can select therapies that specifically target those abnormalities.17 This personalized approach allows oncologists to create personalized treatment plans based on the unique characteristics of an individual’s cancer. This can involve a combination of targeted therapies, chemotherapy, immunotherapy and other interventions tailored to the patient’s specific genetic and molecular profile. Personalized treatment plans aim to maximize efficacy while minimizing potential side effects. PO allows patients to be matched with relevant clinical trials testing new and innovative therapies. This not only provides access to cutting-edge treatments but also contributes to ongoing research, advancing our understanding of cancer and its treatment. PO can play a role in early cancer detection and risk assessment.18 Genetic testing and molecular profiling may identify individuals with a higher predisposition to certain types of cancer, for instance, colorectal cancer, enabling proactive screening, surveillance and preventive measures.19 Thus, precision oncology represents a paradigm shift in cancer care, moving away from a ‘one-size-fits-all’ approach to treatment and embracing a more individualized and targeted strategy based on the unique characteristics of each patient’s cancer.
AI in image analysis
AI applications in medical imaging leverage advanced algorithms and machine learning techniques to enhance the accuracy and efficiency of cancer diagnosis.20 Medical imaging informatics are employed to enhance the current standards of medical care. The integrative analytics methods advocated by the affiliated research branches have the potential to transform the current landscape of imaging informatics in healthcare, affecting both radiology and digital pathology applications (Table 1).
Sl. No. | Software | Imaging data | Analysis |
---|---|---|---|
1 | SubtlePET/SubtleMR | PET/MRI. | Enhancement of PET/MR Images. |
2 | Lung/AI Liver/AI | Lung/CT Liver/CT, MRI. | Segmentation of lesions and nodules. |
3 | AmCAD-UT | AmCad Biomed. | Characterisation and assessment of thyroid issue. |
4 | IDx-DR | Retinal | Feedback on Image quality, and instructions for patient follow-up or referral. |
5 | icobrain | Brain MRI, CT. | Interpretetion of CT and MRI brain images. |
6 | OsteoDetect | Wrist X-ray. | Detection of distal radius fracture. |
7 | AI1 | CT, X-ray of various diseases. | Detection and quantification of abnormalities. |
8 | Aidoc Head/Chest/Spine/Abdomen. | Radiology images. | Detection of acute abnormalities across the body. |
9 | ProFound AI | 2D mammograms. | Detection of malignancies and calcifications. |
10 | Transpara | 2D and 3D mammograms. | Detection and likelihood of cancer. |
11 | Accipio | Head CT. | Triaging of intracranial hemorrhage. |
12 | Paige AI | Digital slides. | Diagnosis for digital pathology. |
Represents some FDA-approved AI-based imaging software.21
There is an unprecedented number of studies that explored the application of AI in diversity of fields and cancer imaging techniques.22 As healthcare services face increasing demands and generate vast amounts of data daily, optimizing and streamlining clinical workflows becomes crucial. AI’s strength in recognizing intricate patterns in images offers the potential to transform image interpretation from a qualitative and subjective task to a quantifiable and easily reproducible one. Additionally, AI can identify information in images that may go unnoticed by humans, thereby complementing clinical decision-making. It also facilitates the integration of diverse data streams, creating powerful diagnostic systems that span radiographic images (Figure 1), genomics, pathology, electronic health records and social networks.23
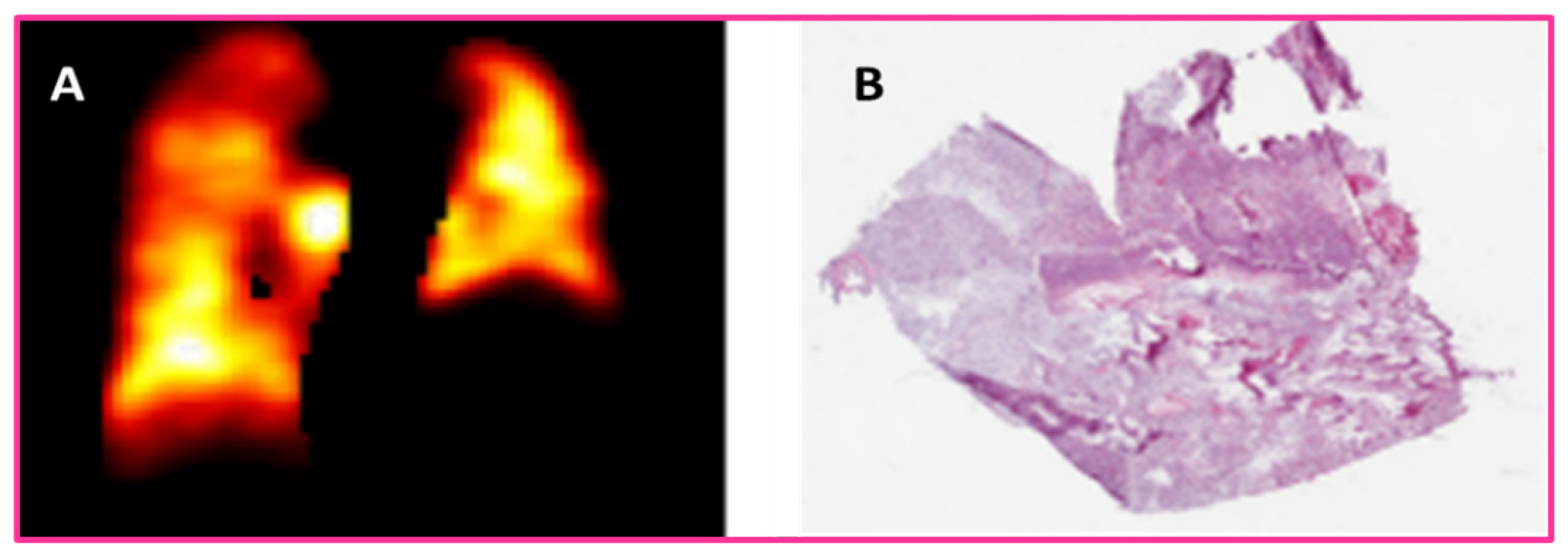
Figure 1:
(A) A typical Q SPECT image displaying lung perfusion in a lung-cancer patient; (B) A magnified, digitized image of brain tissue to look for signs of Glioblastoma (taken from TCGA Glioblastoma Multiforme collection (https://cancergenome.nih.gov/).
On the other hand, deep learning algorithms have emerged as powerful tools for image recognition and feature extraction, offering significant advancements in the understanding and diagnosis of cancer.24,25 Convolutional Neural Networks (CNNs), a prominent deep learning architecture, have demonstrated exceptional capabilities in automatically identifying complex patterns within medical images. In cancer imaging, CNNs play a crucial role in tasks such as tumor detection, segmentation and classification, providing accurate and efficient analyses.26 Recently, a hybrid CNN-support vector machine threshold segmentation approach was employed for the brain tumor detection which showed better accuracy of classification of MRI brain images.27 Autoencoders, another deep learning approach, contribute to feature extraction by learning a compressed representation of medical images.28,29 This condensed representation helps in uncovering intrinsic features relevant to cancer diagnosis, aiding in tasks such as distinguishing between benign and malignant tumors. A method based on computer-aided diagnosis was analyzed for the early detection of disease in patients with asymptomatic breast cancer.30 The proposed model was able to provide a significant response to different morphologies of the breast tissue with the accuracy of more than 95%. Transfer learning, where pre-trained models are adapted to new tasks, is particularly valuable in cancer imaging due to limited labeled datasets, allowing for the efficient use of knowledge gained from large image datasets.31,32 Transfer learning has enabled the advancement of breast cancer diagnosis through ultrasound imaging, addressing the common issue of acquiring an extensive training dataset.33 This is achieved by employing models pre-trained on larger datasets of natural images. Overall, these deep learning algorithms hold immense promise in transforming cancer imaging, facilitating precise diagnoses and contributing to a deeper understanding of tumor characteristics, ultimately advancing cancer research and patient care.
Several case studies underscore the success of AI in various realms of cancer research,34 particularly in radiology, pathology and other imaging modalities. In radiology, AI applications have demonstrated remarkable accuracy in detecting abnormalities and lesions on medical images such as X-rays, CT scans and MRIs.35 For example, AI algorithms have shown effectiveness in identifying early signs of lung cancer on chest X-rays.36,37 In pathology, AI has proven valuable in analyzing histopathological slides, aiding pathologists in diagnosing and grading cancers.38 Notably, AI has showcased its prowess in detecting breast cancer from mammograms.39,40 providing an additional layer of precision to existing diagnostic methods. Furthermore, including molecular imaging and functional imaging, enhancing the understanding of tumor biology and treatment response. These case studies collectively highlight the transformative impact of AI in revolutionizing cancer research across diverse imaging domains.
Implementing AI in image analysis for cancer diagnosis comes with various challenges and considerations. One primary challenge is the need for large and diverse datasets for training robust AI models. Obtaining representative datasets that encompass the heterogeneity of cancers is crucial for ensuring the generalizability of AI algorithms across different patient populations and imaging conditions. Another challenge is the interpretability of AI models, as complex deep learning architectures may function as “black boxes,” making it difficult for clinicians to understand the rationale behind specific predictions.23 Ethical considerations, such as patient privacy and data security, are paramount, necessitating the development and adherence to robust protocols to protect sensitive medical information. Additionally, the integration of AI into clinical workflows requires addressing issues related to standardization, interoperability with existing systems and regulatory compliance. Continuous validation and updating of AI models to accommodate evolving medical knowledge and changing clinical practices are also critical for their sustained effectiveness. Despite these challenges, the potential benefits of AI in enhancing diagnostic accuracy and efficiency make overcoming these hurdles imperative for the successful implementation of AI in image analysis for cancer diagnosis.
AI in data interpretation
AI plays a pivotal role in deciphering complex genetic mutations and signaling pathways in cancer, offering innovative applications that enhance our understanding of the molecular intricacies of cancer biology.41 AI algorithms can analyze vast genomic datasets, identifying and characterizing genetic mutations associated with cancer development.42 By recognizing patterns within genomic data, AI facilitates the discovery of novel mutations and their potential implications in tumorigenesis. Moreover, AI contributes to the elucidation of intricate signaling pathways involved in cancer progression, providing insights into the molecular mechanisms that drive abnormal cellular behaviors.43 The ability of AI to analyze and interpret the complex interactions between genes and proteins enables researchers to unravel the complexities of cancer signaling networks. These applications empower scientists to identify therapeutic targets, predict treatment responses and ultimately advance precision medicine approaches for cancer diagnosis and treatment.
The integration of clinical and molecular data has emerged as a pivotal strategy for achieving more accurate cancer diagnoses and enhancing treatment planning. By combining information from patients’ clinical histories with molecular insights derived from genetic and molecular profiling, a more comprehensive understanding of individual cases can be achieved. Ongoing research in this field aims to leverage these integrated datasets to tailor treatment approaches, predict therapeutic responses and identify targeted therapies.44 For instance, ongoing studies explore how combining clinical information, such as patient demographics and treatment responses, with molecular data, including genomic alterations and biomarker expression, can refine prognostic assessments and guide personalized treatment strategies.45,46 This approach represents a promising avenue for advancing precision medicine in cancer care.
There are several challenges in handling diverse and heterogeneous datasets in cancer research. Handling diverse and heterogeneous datasets poses significant challenges in the field of cancer research.47 One primary challenge is the inherent variability among datasets, stemming from differences in data formats, scales and collection methodologies.48 Integrating data from various sources, such as clinical records, genomic information and imaging data, requires addressing discrepancies in data types and structures. This diversity often leads to complexities in data preprocessing and harmonization, as researchers must develop standardized approaches to ensure compatibility across heterogeneous datasets. Another challenge is the scale of data involved; especially in large-scale multi-omics studies.49 Managing and analyzing vast amounts of diverse data necessitate sophisticated computational tools and infrastructure. This includes addressing issues related to data storage, computational resource requirements and the development of robust algorithms capable of handling the intricacies of diverse datasets. Furthermore, data privacy and ethical considerations present challenges in the sharing and collaboration of heterogeneous datasets.50,51 Integrating clinical, genomic and other sensitive information requires rigorous protocols to protect patient privacy while facilitating meaningful research collaborations. Establishing secure data-sharing frameworks and ensuring compliance with privacy regulations are ongoing challenges in harnessing the full potential of diverse datasets in cancer research.
Addressing these challenges requires interdisciplinary collaboration between researchers, data scientists and informaticians. Standardization efforts, the development of interoperable data formats and advancements in computational techniques are essential for overcoming the hurdles associated with handling diverse and heterogeneous datasets in cancer research. Despite these challenges, the integration of diverse datasets holds immense promise for advancing our understanding of cancer biology; improving diagnostic accuracy and tailoring personalized treatment strategies.
Prediction of patient outcomes
The exploration of AI-driven predictive models represents a transformative approach in cancer treatment, specifically in forecasting patient prognosis and predicting treatment responses.52,53 AI techniques, such as machine learning and deep learning, are harnessed to analyze vast and complex datasets encompassing patient demographics, clinical histories, genomic profiles and treatment outcomes. These models aim to discern intricate patterns within the data, enabling the development of prognostic models that can predict the likely course of the disease for individual patients.54 Additionally, AI-driven models are leveraged to forecast how patients may respond to specific treatments, offering valuable insights for personalized therapeutic strategies.
Ongoing research in this domain focuses on refining the accuracy and reliability of these predictive models. For instance, machine learning algorithms may integrate genomic information to identify molecular signatures associated with treatment outcomes. These models not only aid in prognosis but also contribute to the emerging field of precision medicine by guiding clinicians in selecting the most effective and tailored interventions for individual patients. The exploration of AI-driven predictive models in cancer treatment represents a paradigm shift, promising to enhance clinical decision-making, optimize treatment plans and ultimately improve patient outcomes in the dynamic landscape of cancer care. Recently, an AI-based predictive model was presented which showed more than 95% accuracy in predicting the survival times of the patient diagnosed with pancreatic cancer and performed excellently on test data.55 The consideration of the role of AI in identifying potential biomarkers marks a significant advancement in predicting the efficacy of cancer treatments. AI, particularly machine learning algorithms, plays a crucial role in sifting through vast and intricate datasets comprising genomic, proteomic and clinical information.56 By discerning patterns and relationships within this data, AI-driven models can pinpoint potential biomarkers that may serve as indicators of treatment response. The strength of AI lies in its ability to analyse complex biological data comprehensively, considering the interplay of numerous variables. For example, AI can identify subtle genomic variations or protein expression patterns associated with positive treatment outcomes.57,58 This has the potential to revolutionize the field of cancer treatment by providing clinicians with predictive biomarkers, enabling them to tailor therapies to individual patients.
Ongoing research in this realm focuses on refining AI algorithms to enhance the accuracy and robustness of biomarker predictions.59 Additionally, AI contributes to uncovering novel and previously unrecognized biomarkers, expanding our understanding of the molecular landscape of cancer and paving the way for more targeted and effective treatment strategies.60,61 The consideration of AI in identifying potential biomarkers not only streamlines the development of personalized treatment plans but also holds promise for improving overall treatment efficacy and patient outcomes in the realm of cancer care.
The integration of Electronic Health Records (EHR) and real- world data stands at the forefront of personalized outcome predictions in cancer treatment.62 By amalgamating comprehensive patient data from EHR systems with real-world insights, this approach harnesses a wealth of information encompassing clinical histories, treatment regimens and patient outcomes.63 Utilizing advanced analytics, including machine learning, this integrated data facilitates the development of personalized predictive models for cancer treatment outcomes.64 Through the fusion of EHR and real-world data, clinicians can gain a holistic understanding of individual patient profiles. This includes factors such as demographic information, pre-existing conditions, treatment responses and potential side effects. By analyzing this amalgamated data, predictive models can identify patterns and correlations that contribute to more accurate and individualized predictions regarding treatment efficacy and patient outcomes. Potential pitfalls such as selection bias in sample choice, vague definitions of variables, challenges in implementation, irregular measurement frequency of variables, subjective allocation of treatments and the risk of model overfitting are factors that present limitations in the study.65 Addressing all these issues, ongoing research endeavours in this field focus on optimizing the integration process, refining predictive algorithms and expanding the scope of data sources. The ultimate goal is to empower clinicians with actionable insights, allowing for the tailoring of cancer treatment strategies to the unique characteristics and needs of each patient. The integration of EHR and real-world data thus represents a promising frontier in advancing personalized medicine, enhancing treatment decision-making and ultimately improving the effectiveness of cancer care.
Drug discovery and development
In drug discovery, AI-driven approaches expedite the identification of potential therapeutic compounds by analyzing vast datasets to predict their biological activities and potential efficacy against specific cancer targets.66 These models significantly streamline the early stages of drug development, reducing time and costs. Various machine learning and deep learning algorithms have been integrated into numerous facets of drug discovery procedures, including but not limited to peptide synthesis, structure-based virtual screening, ligand-based virtual screening, toxicity prediction, drug monitoring and release, pharmacophore modeling, quantitative structureactivity relationship, drug repositioning, polypharmacology and physiochemical activity analysis.8,67 Target identification, a critical phase in drug development, benefits from AI’s ability to analyze diverse biological data,68,69 including genomics, proteomics and clinical information.70 AI algorithms can identify novel molecular targets associated with cancer progression, offering valuable insights for developing targeted therapies.71 This accelerates the process of pinpointing specific proteins or pathways implicated in cancer, paving the way for more precise and effective interventions. The exploration of AI-driven algorithms has significantly transformed the landscape of cancer treatment, specifically in predicting drug responses and identifying novel therapeutic targets.53,72 AI leverages advanced machine learning and data analytics to analyze complex datasets encompassing genomics, proteomics and clinical information. In predicting drug responses, AI algorithms scrutinize these datasets to discern patterns that correlate with the effectiveness of specific treatments. This enables the development of predictive models capable of anticipating how individual patients will respond to particular drugs, facilitating the customization of treatment plans for enhanced efficacy. Moreover, AI plays a pivotal role in identifying novel therapeutic targets for cancer treatment. By comprehensively analyzing diverse biological data, including genomic and proteomic profiles, AI algorithms can unveil previously unrecognized molecular targets associated with cancer progression. This innovative approach accelerates the discovery of potential targets, providing valuable insights for the development of targeted therapies. The ability of AI to sift through vast datasets and discern complex relationships positions it as a powerful tool in uncovering new avenues for therapeutic intervention.
Drug repurposing, another area where AI excels, involves identifying existing drugs with untapped potential for cancer treatment.73 AI analyzes comprehensive datasets, linking drug profiles with known cancer-related pathways to uncover novel therapeutic applications.74 This approach not only expedites the drug development process but also maximizes the utility of existing pharmaceuticals. The overarching impact of AI in these areas is reflected in its ability to enhance the efficiency of drug discovery pipelines, reduce costs and promote personalized medicine by tailoring treatments to specific molecular profiles. As AI technologies continue to evolve, their applications in drug discovery, target identification and drug repurposing for cancer hold immense promise for advancing therapeutic interventions and improving patient outcomes. In a recent study, the researchers employed a variety of complementary approaches to analyze a dataset comprising 2766 FDA-approved drugs designed for multiple therapeutic target classes.75 Their investigation led to the identification of 27,371 off-target interactions involving 2013 protein targets and they uncovered 150,620 structurally similar compounds in relation to the drugs in the dataset. The drugs targeting G Protein-Coupled Receptors (GPCRs), enzymes and kinases exhibited the highest number of predicted interactions, with 10,648, 4081 and 3678 interactions, respectively. Significantly, 63% (17,283) of the off-target interactions were confirmed in vitro. Noteworthy findings include approximately 4000 interactions with an IC50 of <100 nM for 1105 FDA-approved drugs and 1661 interactions with an IC50 of <10 nM for 696 FDA-approved drugs. The confirmation of numerous predicted interactions, coupled with an exploration of tissue-specific expression patterns in human and animal tissues, provides valuable insights into potential drug repurposing for novel therapeutic applications. As AI-driven algorithms continue to evolve, their applications in predicting drug responses and identifying therapeutic targets hold immense promise for advancing precision medicine in cancer treatment. This exploration not only enhances our understanding of individualized treatment approaches but also contributes to the development of more effective and targeted therapies, ultimately improving patient outcomes in the realm of cancer care.
Evaluation of the impact of AI on clinical decision-making and patient care
Artificial Intelligence has significantly reshaped the landscape of cancer care by influencing various aspects of clinical decision-making and patient management.76,77 AI-driven diagnostic tools enhance the accuracy of cancer detection and prognosis, providing rapid and precise assessments.78 The personalized treatment approach facilitated by AI, based on genetic and molecular insights, optimizes treatment plans, ensuring better efficacy with minimized adverse effects. AI-driven clinical decision support systems assist healthcare providers in choosing optimal treatment strategies, accelerating drug discovery and streamlining workflows. Moreover, AI contributes to continuous patient monitoring and resource optimization in healthcare institutions, fostering more efficient and personalized cancer care delivery. As AI continues to evolve, challenges such as data privacy and seamless integration into healthcare systems are being addressed.79,80 The ongoing impact of AI in cancer care promises to revolutionize clinical practices, providing more precise, personalized and efficient interventions for the benefit of cancer patients. The translation of Artificial Intelligence research into clinical practice presents a dual landscape of challenges and opportunities.81 Overcoming hurdles related to data privacy, interoperability, regulatory compliance, ethical considerations and user acceptance is critical for the successful integration of AI technologies into healthcare settings. On the flip side, AI brings promising opportunities such as enhanced diagnostics, personalized medicine, workflow optimization, continuous learning and cost savings. Navigating this dynamic landscape requires collaborative efforts among researchers, healthcare professionals, regulators and technology developers, ensuring a balanced and responsible implementation that maximizes the benefits of AI in improving patient care and healthcare efficiency.
Integration of AI into Clinical Practice
Rapid advancements in AI have revolutionized the healthcare by integrating it into clinical practice with the potential to improve patient care and quality of life. Numerous clinical trials are underway to assess the efficacy of AI in cancer diagnosis and treatment.82 For example; researchers are utilizing multimodal deep learning algorithms to analyze medical imaging data for early detection of prostate cancer therapy. Preliminary results indicate a notable improvement in accuracy and speed compared to traditional diagnostic methods.83 Another study utilized machine learning models based on blood profile data to assist physicians in selecting intensive care metastasis breast cancer patients to enhance the overall survival outcome.84 Similarly, several studies are exploring the use of AI-driven predictive models to enhance treatment recommendations based on genetic profiles, showcasing the potential of AI to tailor therapies to individual patients.85
AI has demonstrated a transformative impact on clinical decision-making. In several researches, AI algorithms analyzed patient records and medical literature to recommend personalized treatment plans for cancer patients, considering genetic markers, treatment responses and outcomes.86–88 This approach not only improved the accuracy of treatment decisions but also expedited the process, allowing for more timely interventions. Furthermore, AI-powered decision support systems have shown promise in predicting treatment responses, helping clinicians optimize therapeutic strategies and minimize adverse effects.89,90 Despite the remarkable advancements, the integration of AI into clinical practice poses several challenges. Interpretability of AI models, ethical considerations and data security issues demand careful attention.91,92 Additionally, the successful translation of AI research into routine clinical workflows requires collaboration between researchers, clinicians and regulatory bodies.93 However, these challenges present opportunities for interdisciplinary collaboration, further research and the establishment of robust ethical guidelines to ensure the responsible and effective use of AI in healthcare.94
The integration of AI into clinical practice, especially in the realm of cancer diagnosis and treatment, represents a groundbreaking paradigm shift.95 Ongoing clinical trials showcase the tangible benefits of AI, while its impact on decision-making and patient care is becoming increasingly evident. As we navigate the challenges associated with this integration, it is imperative to recognize the immense potential of AI in revolutionizing healthcare practices and improving patient outcomes. Continued research, ethical considerations and collaborative efforts will pave the way for a future where AI becomes an indispensable tool in the clinician’s arsenal, ultimately enhancing the quality of care provided to patients.
CONCLUSION AND FUTURE PROSPECT
Despite the reported successes of AI in the field of cancer imaging, there are several limitations and challenges that need to be addressed before widespread clinical adoption becomes feasible. An additional constraint involves the interpretability of AI, including the ability to understand the reasoning behind specific outcomes and the ability to predict potential failures. While current research has primarily prioritized enhancing performance over achieving explainability and transparency, ongoing efforts are dedicated to advancing the interpretability of AI. The advantages of instilling trust and transparency in AI systems will vary depending on their performance, enabling the identification of shortcomings when AI falls below human standards. Consequently, this approach transforms AI with superhuman capabilities into a valuable learning resource.
The exploration of emerging trends and future directions in AI-driven cancer research reveals a dynamic landscape poised for transformative advancements. One prominent trend is the increasing integration of multi-modal data, combining genomics, imaging and clinical information. This holistic approach enhances the understanding of cancer biology, paving the way for more comprehensive and personalized treatment strategies. Another trend is the rise of explainable AI, addressing the “black box” nature of complex algorithms.96 Ensuring transparency and interpretability in AI models is becoming a priority, allowing clinicians and researchers to trust and understand the decisions made by these systems, ultimately facilitating their adoption in clinical practice. The advent of federated learning is also gaining prominence, enabling collaborative model training across different institutions without sharing raw patient data. This decentralized approach maintains privacy while leveraging the collective knowledge from diverse datasets to enhance the robustness of AI models.
Future directions in AI-driven cancer research include the refinement of predictive models for treatment responses, the discovery of novel biomarkers through advanced data mining techniques and the development of AI-powered tools for real-time monitoring and early detection. Moreover, AI is anticipated to play a pivotal role in identifying rare subpopulations of cells within tumors, contributing to our understanding of tumor heterogeneity and guiding more targeted therapies. Additionally, the application of AI in drug discovery, including the repurposing of existing drugs and the design of novel compounds, is expected to accelerate the development of innovative and effective cancer treatments. As AI technologies continue to evolve, collaborations between clinicians, data scientists and industry stakeholders will be crucial in shaping the future of cancer research. The ongoing synergy between technology and healthcare expertise holds the promise of unlocking new dimensions in cancer diagnosis, treatment and prevention, ultimately improving patient outcomes and advancing the field of oncology. The integration of AI into precision medicine and personalized cancer care holds immense potential for reshaping the landscape of oncology. Speculation about this integration revolves around several key areas that could significantly impact how we approach the diagnosis and treatment of cancer. One key aspect is the refinement of patient stratification through AI-driven analysis of diverse datasets. By assimilating genomics, clinical and imaging data, AI can identify intricate patterns that contribute to more accurate subtyping of cancers. This finer granularity in patient classification allows for the tailoring of treatment strategies based on individual molecular profiles, paving the way for more precise and effective interventions.
Furthermore, AI is expected to revolutionize treatment selection by predicting responses to specific therapies. Advanced algorithms can analyze large datasets to identify molecular signatures associated with drug responsiveness. This predictive capability enables clinicians to make informed decisions about the most suitable treatment options for individual patients, optimizing therapeutic outcomes. The role of AI in biomarker discovery is another speculative frontier. By sifting through vast datasets, AI algorithms may uncover novel biomarkers indicative of disease progression, treatment efficacy, or potential resistance. This not only enhances our understanding of cancer biology but also contributes to the identification of new targets for therapeutic interventions. Additionally, the integration of AI is anticipated to streamline clinical trial design and recruitment. AI-driven tools can identify eligible patients based on specific molecular criteria, expediting the development and testing of novel therapies. This accelerates the translation of scientific discoveries into clinical applications, bringing innovative treatments to patients more efficiently. While these speculations highlight the promising future of AI in precision medicine and personalized cancer care, challenges such as data privacy, regulatory considerations and the need for interdisciplinary collaboration must be addressed. Nevertheless, the envisioned integration of AI technologies has the potential to usher in a new era of tailored and data-driven approaches to cancer diagnosis and treatment, ultimately improving outcomes for individual patients.
ABBREVIATIONS
AI | Artificial Intelligence |
---|---|
ML | Machine Learning |
DL | Deep Learning |
NLP | Natural Language Processing |
LLMs | Large Language Models |
PO | Precision oncology |
CNNs | Convolutional Neural Networks |
EHR | Electronic Health Records |
GPCRs | G protein-coupled receptors |
References
- Panesar A. Book. 2019:1-368. [CrossRef]
- Shah R, Chircu AJIIIS. IoT and AI in healthcare: A systematic literature review. Issues in Information Systems. 2018;19(3):33-41. [CrossRef] | [Google Scholar]
- Wirries A, Geiger F, Oberkircher L, Jabari SJD. An Evolution Gaining Momentum-The Growing Role of Artificial Intelligence in the Diagnosis and Treatment of Spinal Diseases. Diagnostics. 2022;12(4):836 [PubMed] | [CrossRef] | [Google Scholar]
- Alowais SA, Alghamdi SS, Alsuhebany N, Alqahtani T, Alshaya AI, Almohareb SN, et al. Revolutionizing healthcare: the role of artificial intelligence in clinical practice. BMC Medical Education. 2023;23(1):689 [PubMed] | [CrossRef] | [Google Scholar]
- Rebolledo-Mendez JD, Vaishnav R. Artificial intelligence in precision oncology: The way forward. Published by Scientific Scholar on behalf of International Journal of Molecular and Immuno Oncology. 2022;7(3):77-81. [CrossRef] | [Google Scholar]
- Secinaro S, Calandra D, Secinaro A, Muthurangu V, Biancone PJBMI, Making D, et al. The role of artificial intelligence in healthcare: a structured literature review. BMC Medical Informatics and Decision Making. 2021;21(1):125 [PubMed] | [CrossRef] | [Google Scholar]
- Chen M, Decary M. Artificial intelligence in healthcare: An essential guide for health leaders. Healthcare Management Forum. 2020;33(1):10-18. [PubMed] | [CrossRef] | [Google Scholar]
- Gupta R, Srivastava D, Sahu M, Tiwari S, Ambasta RK, Kumar P, et al. Artificial intelligence to deep learning: machine intelligence approach for drug discovery. 2021;25:1315-60. [PubMed] | [CrossRef] | [Google Scholar]
- Mak KK, Pichika MR. Artificial intelligence in drug development: present status and future prospects. Drug Discovery Today. 2019;24(3):773-80. [PubMed] | [CrossRef] | [Google Scholar]
- Farghali H, Canová NK, Arora M. The potential applications of artificial intelligence in drug discovery and development. Physilogy Research. 2021;70(Suppl4):S715-22. [PubMed] | [CrossRef] | [Google Scholar]
- Tadiboina SN. The use of AI in advanced medical imaging. Journal of Positive School Psychology. 2022;6(11):1939-46. [PubMed] | [CrossRef] | [Google Scholar]
- Kouser S, Aggarwal A. Revolutionizing Healthcare: An AI-Powered X-ray Analysis App for Fast and Accurate Disease Detection. International Journal of Sustainable Development Through AI. 2023;2(1):1-23. [PubMed] | [CrossRef] | [Google Scholar]
- Bokolo AJ. Application of telemedicine and eHealth technology for clinical services in response to COVID-19 pandemic. Health and Technology. 2021;11(2):359-66. [PubMed] | [CrossRef] | [Google Scholar]
- Sussman L, Garcia-Robledo JE, Ordóñez-Reyes C, Forero Y, Mosquera AF, Ruíz-Patiño A, et al. Integration of artificial intelligence and precision oncology in Latin America. Frontiers in Medical Technology. 2022;4:1007822 [PubMed] | [CrossRef] | [Google Scholar]
- Melnekoff DT, Laganà A. Single-cell sequencing technologies in precision oncology. Advances in Experimental Medicine and Biology. 2022;1361:269-82. [PubMed] | [CrossRef] | [Google Scholar]
- Gonzalez-Kozlova EE. Molecular profiling of liquid biopsies for precision oncology. Advances in Experimental Medicine and Biology. 2022;1361:235-47. [PubMed] | [CrossRef] | [Google Scholar]
- Dlamini Z, Skepu A, Kim N, Mkhabele M, Khanyile R, Molefi T, et al. AI and precision oncology in clinical cancer genomics: From prevention to targeted cancer therapies-an outcomes-based patient care. Informatics in Medicine Unlocked. 2022;31:100965 [CrossRef] | [Google Scholar]
- Fitzgerald RC, Antoniou AC, Fruk L, Rosenfeld N. The future of early cancer detection. Nature Medicine. 2022;28(4):666-77. [PubMed] | [CrossRef] | [Google Scholar]
- Andrei P, Battuello P, Grasso G, Rovera E, Tesio N, Bardelli A, et al. Integrated approaches for precision oncology in colorectal cancer: The more you know, the better. Seminars in Cancer Biology. 2022;84:199-213. [PubMed] | [CrossRef] | [Google Scholar]
- Ranschaert ER, Morozov S, Algra PR. Artificial intelligence in medical imaging: opportunities, applications and risks. Book Springer. 2019:1-373. [CrossRef] | [Google Scholar]
- Panayides AS, Amini A, Filipovic ND, Sharma A, Tsaftaris SA, Young A, et al. AI in medical imaging informatics: current challenges and future directions. IEEE Journal of Biomedical and Health Informatics. 2020;24(7):1837-57. [PubMed] | [CrossRef] | [Google Scholar]
- Koh D-M, Papanikolaou N, Bick U, Illing R, Kahn CE, Kalpathi-Cramer J, et al. Prior. Artificial intelligence and machine learning in cancer imaging. Communications Medicine. 2022;2(1):133 [PubMed] | [CrossRef] | [Google Scholar]
- Bi WL, Hosny A, Schabath MB, Giger ML, Birkbak NJ, Mehrtash A, et al. Artificial intelligence in cancer imaging: clinical challenges and applications. CA: A Cancer Journal for Clinicians. 2019;69(2):127-57. [PubMed] | [CrossRef] | [Google Scholar]
- Nazir M, Shakil S, Khurshid K. Role of deep learning in brain tumor detection and classification (2015 to 2020): A review. Computerized Medical Imaging and Graphics. 2021;91:101940 [PubMed] | [CrossRef] | [Google Scholar]
- Zhao L, Jia K. Multiscale CNNs for brain tumor segmentation and diagnosis. Computational and Mathematical Methods in Medicine. 2016;2016:8356294 [PubMed] | [CrossRef] | [Google Scholar]
- Yang R, Yu Y. Artificial convolutional neural network in object detection and semantic segmentation for medical imaging analysis. Frontiers in Oncology. 2021;11:638182 [PubMed] | [CrossRef] | [Google Scholar]
- Khairandish MO, Sharma M, Jain V, Chatterjee JM, Jhanjhi NJ. A hybrid CNN-SVM threshold segmentation approach for tumor detection and classification of MRI brain images. Innovation and Research in BioMedical engineering. 2022;43(4):290-9. [PubMed] | [CrossRef] | [Google Scholar]
- Mallick PK, Ryu SH, Satapathy SK, Mishra S, Nguyen GN, Tiwari P, et al. Brain MRI image classification for cancer detection using deep wavelet autoencoder-based deep neural network. IEEE Access. 2019;7:46278-87. [CrossRef] | [Google Scholar]
- Anand L, Maurya M, Seetha J, Nagaraju D, Ravuri A, Vidhya R, et al. An intelligent approach to segment the liver cancer using Machine Learning Method. 2023 [CrossRef] | [Google Scholar]
- Zadeh HG, Fayazi A, Binazir B, Yargholi M. Breast cancer diagnosis based on feature extraction using dynamic models of thermal imaging and deep autoencoder neural networks. Journal of Testing and Evaluation. 2020;49(3):1516-32. [CrossRef] | [Google Scholar]
- Alzubaidi L, Fadhel MA, Al-Shamma O, Zhang J, Santamaría J, Duan Y, et al. Towards a better understanding of transfer learning for medical imaging: a case study. Applied Sciences. 2020;10(13):4523 [CrossRef] | [Google Scholar]
- Alzubaidi L, Al-Amidie M, Al-Asadi A, Humaidi AJ, Al-Shamma O, Fadhel MA, et al. Novel transfer learning approach for medical imaging with limited labeled data. Cancers. 2021;13(7):1590 [PubMed] | [CrossRef] | [Google Scholar]
- Ayana G, Dese K, Choe SW. Transfer learning in breast cancer diagnoses via ultrasound imaging. Cancers. 2021;13(4):738 [PubMed] | [CrossRef] | [Google Scholar]
- Zhou SK, Greenspan H, Davatzikos C, Duncan JS, Van Ginneken B, Madabhushi A, et al. A review of deep learning in medical imaging: Imaging traits, technology trends, case studies with progress highlights and future promises. Proceedings of the IEEE. 2021;109(5):820-38. [PubMed] | [CrossRef] | [Google Scholar]
- Bera K, Braman N, Gupta A, Velcheti V, Madabhushi A. Predicting cancer outcomes with radiomics and artificial intelligence in radiology. Nature Reviews Clinical Oncology. 2022;19(2):132-46. [PubMed] | [CrossRef] | [Google Scholar]
- de Margerie-Mellon C, Chassagnon GJD, Imaging I. Artificial intelligence: A critical review of applications for lung nodule and lung cancer. Diagnostic and Interventional Imaging. 2023;104(1):11-7. [PubMed] | [CrossRef] | [Google Scholar]
- Jones CM, Buchlak QD, Oakden-Rayner L, Milne M, Seah J, Esmaili N, et al. Chest radiographs and machine learning-Past, present and future. Journal of Medical Imaging and Radiation Oncology. 2021;65(5):538-44. [PubMed] | [CrossRef] | [Google Scholar]
- Moxley-Wyles B, Colling R, Verrill C. Artificial intelligence in pathology: an overview. Diagnostic Histopathology. 2020;26(11):513-20. [CrossRef] | [Google Scholar]
- Lång K, Dustler M, Dahlblom V, Åkesson Aandersson I, Zackrisson S. Identifying normal mammograms in a large screening population using artificial intelligence. European Radiology. 2021;31(3):1687-92. [CrossRef] | [Google Scholar]
- Sechopoulos I, Teuwen J, Mann R. Artificial intelligence for breast cancer detection in mammography and digital breast tomosynthesis: State of the art. in Seminars in cancer biology. 2021;72:214-25. [PubMed] | [CrossRef] | [Google Scholar]
- Dlamini Z, Francies FZ, Hull R, Marima R. Artificial intelligence (AI) and big data in cancer and precision oncology. Computational and Structural Biotechnology Journal. 2020;18:2300-11. [PubMed] | [CrossRef] | [Google Scholar]
- Vadapalli S, Abdelhalim H, Zeeshan S, Ahmed Z. Artificial intelligence and machine learning approaches using gene expression and variant data for personalized medicine. Briefings in Bioinformatics. 2022;23(5):bbac191 [PubMed] | [CrossRef] | [Google Scholar]
- Almansour NM. Triple-negative breast cancer: a brief review about epidemiology, risk factors, signaling pathways, treatment and role of artificial intelligence. Frontiers in Molecular Biosciences. 2022;9:836417 [PubMed] | [CrossRef] | [Google Scholar]
- Cifci D, Foersch S, Kather JN. Artificial intelligence to identify genetic alterations in conventional histopathology. Journal of Pathology. 2022;257(4):430-44. [PubMed] | [CrossRef] | [Google Scholar]
- Paksoy N. and Yağin FH. Artificial intelligence-based colon cancer prediction by identifying genomic biomarkers. International Medical Journal. 2022;4(2):196-202. [CrossRef] | [Google Scholar]
- Volovat S-R, Augustin I, Zob D, Boboc D, Amurariti F, Volovat C, et al. Use of Personalized Biomarkers in Metastatic Colorectal Cancer and the Impact of AI. Cancers. 2022;14(19):4834 [PubMed] | [CrossRef] | [Google Scholar]
- Cirillo D, Núñez-Carpintero I, Valencia A. Artificial intelligence in cancer research: learning at different levels of data granularity. Molecular Oncology. 2021;15(4):817-29. [PubMed] | [CrossRef] | [Google Scholar]
- Noor AM, Holmberg L, Gillett C, Grigoriadis A. Big Data: the challenge for small research groups in the era of cancer genomics. British Journal of Cancer. 2015;113(10):1405-12. [PubMed] | [CrossRef] | [Google Scholar]
- Herrmann M, Probst P, Hornung R, Jurinovic V, Boulesteix AL. Large-scale benchmark study of survival prediction methods using multi-omics data. Briefings in Bioinformatics. 2021;22(3):bbaa167 [PubMed] | [CrossRef] | [Google Scholar]
- Mittelstadt BD, Floridi LJ. The ethics of big data: current and foreseeable issues in biomedical contexts. Science and Engineering Ethics. 2016(2):45-80. [PubMed] | [CrossRef] | [Google Scholar]
- Bonomi L, Huang Y, Ohno-Machado L. Privacy challenges and research opportunities for genomic data sharing. Nature Genetics. 2020;52(7):646-54. [PubMed] | [CrossRef] | [Google Scholar]
- Workman P, Antolin AA, Al-Lazikani B. Transforming cancer drug discovery with Big Data and AI. Expert Opinion on Drug Discovery. 2019;14(11):1089-95. [PubMed] | [CrossRef] | [Google Scholar]
- Tippur A. AI-Powered Precision Oncology: Computational Insights Redefining Therapeutic Landscapes. Special Edition: Junior Commentary. 2023;3(S1):1-10. [PubMed] | [CrossRef] | [Google Scholar]
- Corti C, Cobanaj M, Dee EC, Criscitiello C, Tolaney SM, Celi LA, et al. Artificial intelligence in cancer research and precision medicine: Applications, limitations and priorities to drive transformation in the delivery of equitable and unbiased care. Cancer Treatment Reviews. 2023;112:102498 [PubMed] | [CrossRef] | [Google Scholar]
- Chakraborty A, Tsokos CP. An AI-driven Predictive Model for Pancreatic Cancer Patients Using Extreme Gradient Boosting. Journal of Statistical Theory and Applications. 2023;22(4):262-82. [CrossRef] | [Google Scholar]
- Xu J, Yang P, Xue S, Sharma B, Sanchez-Martin M, Wang F, et al. Translating cancer genomics into precision medicine with artificial intelligence: applications, challenges and future perspectives. Human Genetics. 2019;138(2):109-24. [PubMed] | [CrossRef] | [Google Scholar]
- Walter W, Pohlkamp C, Meggendorfer M, Nadarajah N, Kern W, Haferlach C, et al. Artificial intelligence in hematological diagnostics: Game changer or gadget?. Blood Reviews. 2023;58:101019 [PubMed] | [CrossRef] | [Google Scholar]
- Lee M. Recent advances in generative adversarial networks for gene expression data: a comprehensive review. Mathematics. 2023;11(14):3055 [CrossRef] | [Google Scholar]
- Danishuddin Khan S, Kim JJ. From cancer big data to treatment: Artificial intelligence in cancer research. The Journal of Gene Medicine. 2024;26(1):e3629 [PubMed] | [CrossRef] | [Google Scholar]
- Waldstein SM, Seeböck P, Donner R, Sadeghipour A, Bogunović H, Osborne A, et al. Unbiased identification of novel subclinical imaging biomarkers using unsupervised deep learning. Scientific Reports. 2020;10(1):12954 [PubMed] | [CrossRef] | [Google Scholar]
- Mäkelä K, Mäyränpää MI, Sihvo H-K, Bergman P, Sutinen E, Ollila H, et al. Artificial intelligence identifies inflammation and confirms fibroblast foci as prognostic tissue biomarkers in idiopathic pulmonary fibrosis. Human Pathology. 2021;107:58-68. [PubMed] | [CrossRef] | [Google Scholar]
- Rinderknecht MD, Klopfenstein Y. Predicting critical state after COVID-19 diagnosis: model development using a large US electronic health record dataset. 2021;4(1):113 [PubMed] | [CrossRef] | [Google Scholar]
- Matsumoto K, Nohara Y, Sakaguchi M, Takayama Y, Fukushige S, Soejima H, et al. Temporal Generalizability of Machine Learning Models for Predicting Postoperative Delirium Using Electronic Health Record Data: Model Development and Validation Study. JMIR Perioperative Medicine. 2023;6(1):e50895 [PubMed] | [CrossRef] | [Google Scholar]
- Francis AP, Khan SA, Fuloria S, Fuloria NK, Meenakshi DU. Big Data and Precision Oncology in Healthcare, in Big Data in Oncology: Impact, Challenges and Risk Assessment. River Publishers. 2023:47-74. [PubMed] | [CrossRef] | [Google Scholar]
- Sauer CM, Chen LC, Hyland SL, Girbes A, Elbers P, Celi L, et al. Leveraging electronic health records for data science: common pitfalls and how to avoid them. 2022;4(12):e893-8. [PubMed] | [CrossRef] | [Google Scholar]
- David L, Thakkar A, Mercado R, Engkvist O. Molecular representations in AI-driven drug discovery: a review and practical guide. Journal of Cheminformatics. 2020;12(1):1-22. [PubMed] | [CrossRef] | [Google Scholar]
- Jiménez-Luna J, Grisoni F, Weskamp N, Schneider G. Artificial intelligence in drug discovery: recent advances and future perspectives. Expert Opinion on Drug Discovery. 2021;16(9):949-59. [PubMed] | [CrossRef] | [Google Scholar]
- Vamathevan J, Clark D, Czodrowski P, Dunham I, Ferran E, Lee G, et al. Applications of machine learning in drug discovery and development. 2019;18(6):463-77. [PubMed] | [CrossRef] | [Google Scholar]
- Paul D, Sanap G, Shenoy S, Kalyane D, Kalia K, Tekade R, et al. Artificial intelligence in drug discovery and development. Drug Discov Today. 2021;26(1):80 [PubMed] | [CrossRef] | [Google Scholar]
- Pun FW, Ozerov IV, Zhavoronkov A. AI-powered therapeutic target discovery. Trends in Pharmacological Sciences. 2023;44(9):561-72. [PubMed] | [CrossRef] | [Google Scholar]
- You Y, Lai X, Pan Y, Zheng H, Vera J, Liu S, et al. Artificial intelligence in cancer target identification and drug discovery. Signal Transduction and Targeted Therapy. 2022;7(1):156 [PubMed] | [CrossRef] | [Google Scholar]
- Arigbede O, Amusa T, Buxbaum S. Exploring the Use of Artificial Intelligence and Robotics in Prostate Cancer Management. Cureus. 2023;15(9):e46021 [PubMed] | [CrossRef] | [Google Scholar]
- Zheng P, Wang S, Wang X, Zeng X. Artificial Intelligence in Bioinformatics and Drug Repurposing: Methods and Applications. Frontiers in Genetics. 2022;13(Issue):870795 [PubMed] | [CrossRef] | [Google Scholar]
- Partin A, Brettin TS, Zhu Y, Narykov O, Clyde A, Overbeek J, et al. Deep learning methods for drug response prediction in cancer: predominant and emerging trends. Frontiers in Medicine. 2023;10:1086097 [PubMed] | [CrossRef] | [Google Scholar]
- Rao M, McDuffie E, Sachs C. Artificial Intelligence/Machine Learning-Driven Small Molecule Repurposing via Off-Target Prediction and Transcriptomics. 2023;11(10):875 [PubMed] | [CrossRef] | [Google Scholar]
- Sardar P, Abbott JD, Kundu A, Aronow HD, Granada JF, Giri J, et al. Impact of artificial intelligence on interventional cardiology: from decision-making aid to advanced interventional procedure assistance. JACC: Cardiovascular Interventions. 2019;12(14):1293-303. [CrossRef] | [Google Scholar]
- Harish V, Morgado F, Stern AD, Das S. Artificial intelligence and clinical decision making: the new nature of medical uncertainty. Academic Medicine. 2021;96(1):31-6. [PubMed] | [CrossRef] | [Google Scholar]
- Christie JR, Lang P, Zelko LM, Palma DA, Abdelrazek M, Mattonen S, et al. Artificial intelligence in lung cancer: bridging the gap between computational power and clinical decision-making. Canadian Association of Radiologists Journal. 2021;72(1):86-97. [CrossRef] | [Google Scholar]
- Thapa C, Camtepe S. Precision health data: Requirements, challenges and existing techniques for data security and privacy. Computers in Biology and Medicine. 2021;129:104130 2020;104130 [PubMed] | [CrossRef] | [Google Scholar]
- Marcu LG, Boyd C. Current issues regarding artificial intelligence in cancer and health care. Implications for medical physicists and biomedical engineers. 2019;9:375-81. [CrossRef] | [Google Scholar]
- Aldoseri A, Al-Khalifa KN, Hamouda AM. Re-Thinking Data Strategy and Integration for Artificial Intelligence: Concepts, Opportunities and Challenges. Applied Sciences. 2023;13(12):7082 [CrossRef] | [Google Scholar]
- Askin S, Burkhalter D, Calado G, El Dakrouni S. Artificial Intelligence Applied to clinical trials: opportunities and challenges. Health and Technology. 2023;13(2):203-13. [PubMed] | [CrossRef] | [Google Scholar]
- Esteva A, Feng J, Van der Wal D, Huang SC, Simko JP, DeVries S, et al. Prostate cancer therapy personalization via multi-modal deep learning on randomized phase III clinical trials. npj Digital Medicine-Nature. 2022;5(1):71 [PubMed] | [CrossRef] | [Google Scholar]
- Botlagunta M, Botlagunta MD, Myneni MB, Lakshmi D, Nayyar A, Gullapalli JS, et al. Classification and diagnostic prediction of breast cancer metastasis on clinical data using machine learning algorithms. Scientific Reports. 2023;13(1):485 [PubMed] | [CrossRef] | [Google Scholar]
- Shao D, Dai Y, Li N, Cao X, Zhao W, Cheng L, Rong Z, Huang L, Wang Y, Zhao J, et al. Artificial intelligence in clinical research of cancers. 2022;23(1):bbab523 [PubMed] | [CrossRef] | [Google Scholar]
- Liao J, Li X, Gan Y, Han S, Rong P, Wang W, Li W, et al. Artificial intelligence assists precision medicine in cancer treatment. Frontiers in Oncology. 2023;12:998222 [PubMed] | [CrossRef] | [Google Scholar]
- Torrente M, Sousa PA, Hernández R, Blanco M, Calvo V, Collazo A, et al. An artificial intelligence-based tool for data analysis and prognosis in cancer patients: Results from the Clarify study. Cancers. 2022;14(16):4041 [PubMed] | [CrossRef] | [Google Scholar]
- Yang X, Mu D, Peng H, Li H, Wang Y, Wang P, et al. Research and application of artificial intelligence based on electronic health records of patients with cancer: systematic review. JMIR Medical Informatics. 2022;10(4):e33799 [PubMed] | [CrossRef] | [Google Scholar]
- Molefi T, Marima R, Demetriou D, Basera A, Dlamini Z. Employing AI-Powered Decision Support Systems in Recommending the Most Effective Therapeutic Approaches for Individual Cancer Patients: Maximising Therapeutic Efficacy, in Artificial Intelligence and Precision Oncology: Bridging Cancer Research and Clinical Decision Support. 2023:259-75. [CrossRef] | [Google Scholar]
- Zhang Z, Wei X. Artificial intelligence-assisted selection and efficacy prediction of antineoplastic strategies for precision cancer therapy. Seminars in Cancer Biology. 2023;90:57-72. [PubMed] | [CrossRef] | [Google Scholar]
- John-Mathews J-M. Some critical and ethical perspectives on the empirical turn of AI interpretability. Technological Forecasting and Social Change. 2022;174:121209 [CrossRef] | [Google Scholar]
- Lisboa P, Saralajew S, Vellido A, Fernández-Domenech R, Villmann TJN. The coming of age of interpretable and explainable machine learning models. Conference: ESANN 2021 – European Symposium on Artificial Neural Networks, Computational Intelligence and Machine Learning. 2023;535:25-39. [CrossRef] | [Google Scholar]
- El Naqa I, Karolak A, Luo Y, Folio L, Tarhini AA, Rollison D, Parodi KJO, et al. Translation of AI into oncology clinical practice. Oncogenes. 2023;42(42):3089-97. [PubMed] | [CrossRef] | [Google Scholar]
- Bernstam EV, Shireman PK, Meric-Bernstam FN, Zozus M, Jiang X, Brimhall BB, et al. Artificial intelligence in clinical and translational science: Successes, challenges and opportunities. Clinical and Translational Science. 2022;15(2):309-21. [PubMed] | [CrossRef] | [Google Scholar]
- Popescu ER, Geantă M, Brand A. Mapping of clinical research on artificial intelligence in the treatment of cancer and the challenges and opportunities underpinning its integration in the European Union health sector. European Journal of Public Health. 2022;32(3):443-9. [PubMed] | [CrossRef] | [Google Scholar]
- Claus C, Ferrara-Koller C, Klein C. Monoclonal antibodies. 2023 [PubMed] | [CrossRef] | [Google Scholar]