ABSTRACT
Aim
A Scientometric study examines the publication’s quantitative and qualitative trends in a particular research area during the year 2023.
Materials and Methods
The present study investigates 333 ChatGPT research outputs of world published 2023, indexed in the Web of Science Core Collection database software, including bibliometric Biblioshiny and VOSviewer, have been used for data Analysis. Further, we have analysed the top 15 authors, institutions, countries and journals were analysed with the various bibliometric indicators.
Results
Annals of Biomedical Engineering journal has the highest number of publications 8.982% in the world. Duke University, USA has the maximum number of publications 1.80%, The authors who registered the highest number of publications were Anonymous, He YB And Wu HY (USA), most productive publisher were identified from Springer Nature published 20.606% publications.
Conclusion
This study suggests that the number of publications on ChatGPT have remained stable over the year and maximum research emerged from the development countries. The quality of the study is entirely depending on the input information imported from the Web of Science (WoS) database.
INTRODUCTION
ChatGPT is a powerful language model developed by OpenAI based on the GPT-3.5 architecture, designed to generate human-like text responses in a conversational manner. With its advanced machine learning algorithms, ChatGPT can understand natural language input and provide accurate and meaningful responses, making it a valuable tool for a wide range of applications, from chatbots and customer service to virtual assistants and content creation. The development of ChatGPT is a result of years of research in the field of artificial intelligence and natural language processing. OpenAI is one of the leading research institutions in this field, dedicated to developing AI systems that can improve our lives and solve some of the world’s most pressing problems. With ChatGPT, OpenAI aims to create a more natural and intuitive way for humans to interact with computers and machines, allowing for more efficient and effective communication and problem-solving.1
ChatGPT is trained on a massive amount of data, consisting of billions of words from various sources, including books, articles, and websites. The training data is carefully curated to ensure that the model can learn the nuances of language and understand the context in which words are used. With such vast amounts of data and powerful computing resources, ChatGPT can generate responses that are not only grammatically correct but also semantically meaningful and contextually relevant. One of the most impressive features of ChatGPT is its ability to adapt to new situations and learn from experience. As users interact with the system, ChatGPT can learn from their inputs and adjust its responses to provide more accurate and helpful information. This means that over time, the system can become more personalized and tailored to individual users’ needs, leading to a more efficient and satisfying experience.1,3
ChatGPT has a wide range of potential applications in various fields, including customer service, education, healthcare, and entertainment. For example, businesses can use ChatGPT to provide 24/7 customer support, allowing customers to quickly get answers to their questions and resolve any issues they may have. In education, ChatGPT can assist students in learning and answering questions, providing a more personalized and interactive learning experience. In healthcare, ChatGPT can provide patients with information about their medical conditions and treatments, helping to improve their understanding and adherence to their healthcare plans. Finally, in entertainment, ChatGPT can be used to generate creative writing, music, and even art, providing a new way for artists to express themselves and create new forms of content.1,5
In summary, ChatGPT is a powerful language model that has the potential to revolutionize the way we interact with machines and computers. Its advanced machine learning algorithms and ability to adapt to new situations make it a valuable tool for a wide range of applications, from customer service to education and entertainment. As ChatGPT continues to evolve and improve, we can expect to see it play an increasingly important role in our daily lives, helping us to communicate more effectively and efficiently with the world around us.
Analysing the impact of ChatGPT using scientometrics is an important research area that aims to evaluate the influence of ChatGPT on various fields. Scientometrics is the study of quantitative measures used to analyse scientific and research activities, such as citations, publications, and collaborations. By applying scientometric methods to ChatGPT, researchers can assess the extent to which ChatGPT has influenced different domains and how it has contributed to advancing research in those areas. The use of scientometrics can help to identify the most impactful applications of ChatGPT, the most relevant research directions, and potential future areas of development. Moreover, it can also provide insights into the development of other language models and the evolution of natural language processing as a whole.
REVIEW OF LITERATURE
Few Scientometric studies have been published on this ‘ChatGPT’ research. Among them, Kirtania’s study6 emphasizes the benefits that using ChatGPT can bring to researchers, including data visualization, data analysis, and data interpretation. This suggests that ChatGPT can assist researchers in handling and understanding large amounts of data, potentially enhancing their productivity and efficiency in data-driven tasks.
Characterization of ChatGPT as a large language model based on neural networks aligns with the general understanding of ChatGPT as a powerful AI system that learns from vast amounts of human-generated text and generates intelligent responses. This highlights the underlying technology and methodology employed in ChatGPT’s development.7
Khosravi et al.’s8 analysis of the field of chatbots demonstrates the growing attention and increasing number of publications in this area. Although their study does not specifically focus on ChatGPT, it suggests a rising interest and recognition of chatbots, which can include models like ChatGPT, in various domains.
Another paper discussed regarding the foresight of AI, particularly deep learning, being used in a variety of applications aligns with the broader expectations and potential applications of AI technologies, including systems like ChatGPT. This implies that ChatGPT and similar language models can find utility across different user groups, from specialty doctors to paramedics.9
Li et al.’s study,10 while not directly related to ChatGPT, provides a quantitative summary and review of research in the field of urban microclimate. While this study may not offer specific details about ChatGPT or its impact, it demonstrates the application of scientometric methods to analyze a specific research domain.
Overall, while these studies do not directly focus on the impact of ChatGPT itself, they provide valuable insights into related areas and highlight the broader context in which ChatGPT operates. Further scientometric studies specifically targeting the impact of ChatGPT could provide more specific and comprehensive analyses of its influence in various fields.
MATERIALS AND METHODS
Bibliometrics refers to the method of applying mathematical statistics to quantitatively analyse the temporal and spatial distribution properties of scientific documents in a specific field, which can realize the scientific transformation of documents from data to knowledge.11 In bibliometrics, there are two main procedures: performance analysis and science mapping. Performance analysis is based on bibliographic data to assess the impact of groups of scientific actors (countries, institutions, and researchers) and their activities. Science mapping aims to show the knowledge structure, dynamic evolution, and trends in the research field, which can provide spatial representation through physical proximity and relative position to show the relationships between disciplines, fields, papers, or authors.12 The most commonly used analytical methods of bibliometrics are documents co-citation and co-word analysis. Documents co-citation refers to mapping the knowledge structure of the research field through the commonly cited paired documents. Co-word analysis is a kind of content-analysis technology, which directly deals with the term set shared by documents and maps relevant documents through the interaction of key terms. The results of co-citation or co-word analysis can be used for a variety of purposes, such as identifying current research hotspots and frontiers and analysing the evolution and trend of knowledge structure.1,13
The VOSviewer software developed by Leiden University in the Netherlands can realize the mining of literature authors, journals, countries, and other information through bibliometrics, and can also carry out visual analysis by constructing citation networks and co-occurrence networks. This software has advantages in the accuracy of information mining, network density, and cluster visualization. In this paper, VOSviewer software was used to mine the information of authors, journals, and institutions of health technology literature, as well as to analyse the map of the core author group, key points of published journals, and the cooperative network of institutions.
Our search revealed that there were a total 333 articles in Web of Science database on ChatGPT were exported in MS-Excel for further analysis for the year of Publications, affiliations, type and source of documents, funding sources, International Collaborative Publications (ICPs), leading organizations and authors prominent journals and significant subject keywords.
RESULTS
From the Web of Science (WoS) database 333 publications were found on the Topic “ChatGPT” publications during the last year 2023 (June 18, 2023).
The Table 1 provides a summary of the data, presenting key information related to a specific topic. The data covers a timespan of the year 2023 and includes findings from 200 different sources, such as journals and books, among others. A total of 333 documents were analysed, and on average, each document has been cited 2.303 times, indicating the level of impact and relevance of the research. Moreover, these documents contain a substantial number of references, totalling 5,976. Examining the content of the documents, there are 170 identified Keywords Plus (ID), which serve as additional descriptive terms or phrases associated with the research. Additionally, the authors provided 561 Author’s Keywords (DE), showcasing their own chosen keywords that summarize the main themes and concepts of their work.
Considering the authors involved, a significant number of 932 contributors are associated with the documents. Among them, 86 authors have authored single-authored documents, highlighting their independent research contributions. Collaboration among authors is also evident, with 99 of the documents being co-authored. On average, there are approximately 3.31 co-authors per document, indicating a cooperative approach to conducting research. Furthermore, the data indicate that international co-authorships accounted for 24.62% of the collaborations, suggesting a global perspective and the exchange of knowledge across different regions and institutions. The Table 1 also provides insights into the types of documents included. The majority of the documents fall into the categories of articles (119) and editorial material (105), indicating the presence of research papers and publications with expert commentary. Additionally, there are 85 letters, 14 reviews, 9 news items, and 1 book review, illustrating a diverse range of document types within the dataset (Table 1).
Geographical distribution
Among global research on “ChatGPT” 59 countries participated. The USA took the lead by contributing the largest number of publications 33.234% (n=111) with 208 citations, to be followed by China 11.976% (n=40), with 87 citations, India 7.784% (n=26) with 34 citations, England 7.784% (n=25) with 78 citations, Australia 6.587% (n=22) with 42 citations, Germany 5.389% (n=18) with 45 citations, Italy 5.09% (n=16) with 58 citations, Switzerland 4.192% (n=14) with 54 citations, Netherlands 3.593% (n=12) with 87 citations, France 2.695% (n=9) with 32 citations, aSpain 2.695% (n=8) with 25 citations, Austria and Sweden published 2.096% (n=7) publications with 16,15 citations, and Scotland and South Africa published 1.497% (n=5) with 38 and 28 citations. The top 15 countries, institutions, and authors with the most publications are listed in Table 2.
Collaborative Linkages among top 15 Countries
The Top 15 most productive countries collaborated with each other ChatGPT research but the scale of their collaboration measured in terms of collaborative linkages varied from 13 to 67. The top countries with the most collaborative linkages count are: USA (67 linages), China (32 linkages) and Switzerland (30 linkages). On the other hand, Australia, Scotland (13 linkages), Spain (16 linkages) accounted for the lowest collaborative linkages count. The country co-authorship network of ChatGPT, built by the VOS viewer, is presented in Figure 1.
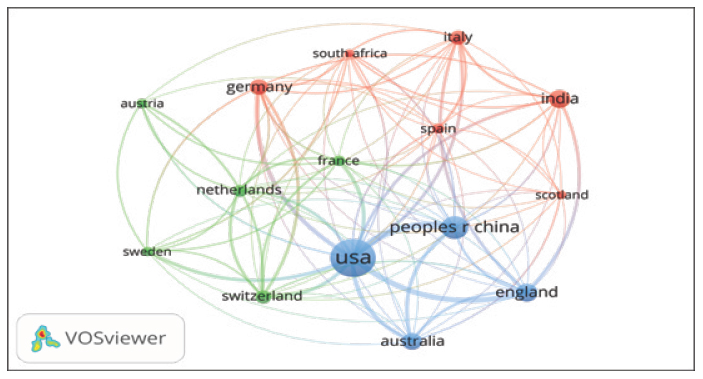
Figure 1:
Countries collaborations Network Map of top 15 Countries
Broad subject areas
Using Web of Science (WoS) database classification, the publications on “ChatGPT” were broadly classified under five subjects. Surgery (with 10.81% share) contributed the most publications, followed by Engineering Biomedical (11.21% share, Education Educational research (6.30% share), Medicine General Internal (5.40% share), Computer Science and Information systems (5.40% share) etc., (Table 3).
Most productive and impactful organisations
In all a total 609 research organisations were found to have participated in ChatGPT research in the year of 2023. The top 15 organisations contributed 1 to 16 papers and these together contributed 69 papers which received 181 citations, accounting more than 100% share each in global publications and citations. On further analysis, it was observed that 14 organisations contributed more than the average publication productivity (4.6%) of all 15 organisations: Duke University, USA, Tianjin Med University, China, University of Illinois, USA (6 papers and 1.80% share), In terms of least productive 9 organisations contributed 4 papers each. The average publications contributed by the top 15 organisations, only 3 organisations contributed more than these average publications productivity.
The top 6 organisation contributes In Terms of Most Collaborative Linkages Were: Duke University, USA, Tianjin Med University, China, Beijing Sport University, China, Zhengzhou University, China, University of Illinois, USA, Drexel University, USA (16, 16, 14, 14, 11 and 11 linkages). The three top organizations in terms of least collaborative linkages were: St Louis University, Spain, University of Texas Medical Branch, USA, Weill Cornell Graduate School of Medical Sciences, USA, University of Queensland, Australia, University of Southern Denmark, Denmark (2, 2, 2, 1, and 1 linkages).
The top organisations collaboration networks are shown in Figures 2 and 3. All 15 organisations were divided into 5 cluster. Cluster1 (Green, 3 organisations) includes the Duke University, USA, Tianjin Med University, China and University of Illinois, USA, Cluster 2 (Red, 5 organisations) includes Drexel University, USA, Chinese Academy Science, China, Zhengzhou University, China, etc., (Table 4).
Top 15 most productive authors
The authors are affiliated with various universities and institutions in different countries, including China, the USA, Slovakia, and Australia. The 971 authors participated in 333 global papers in ChatGPT research within the year of 2023: 4 authors contributed 1-5 publications each, only one author contributed 5-6 publications. Among 15 top authors, the dominant participation comes from USA authors, followed by 5 from China, Bratislava 2 authors and one author from Australia. The individual research output of the top 15 authors varied from 3 to 6 publications per author and together contributed 56 publications and 112 citations, which present 3.73% and 7.46% share of global publications and citations in ChatGPT research.
Wu, Haiyang from Anhui University of Chinese Medicine in China has 6 publications, 13 citations, a Citation per paper of 2.167, and Total link Strength of 21, Cheng, Kunming from Zhengzhou University in China has 5 publications, 9 citations, Citation per paper of 1.800, and a Total link Strength of 19, He, Yongbin from Beijing Sport University in China has 5 publications, 13 citations, Citation per paper of 2.600, and a Total link Strength of 19, Camacho, Justin M. from Drexel University in the USA has 4 publications, 8 citations, Citation per paper of 2.000, and Total link Strength of 16, Gu, Shuqin from Duke University in the USA has 4 publications, 12 citations, Citation per paper of 3.000, and Total link Strength of 16 etc., (Table 5).
Table 6 provides a comprehensive overview of various journals that have published articles related to ChatGPT. Among the listed journals, several notable publications stand out. The Annals of Biomedical Engineering leads the list with 30 articles published on ChatGPT, resulting in a moderate TC of 38. These articles have received an average of 1.267 citations each, indicating a decent level of interest and impact within the field. The journal’s TLS score of 12 reflects its contributions to the research community.
Description | Results |
---|---|
Main information about data | |
Timespan | 2023 |
Sources (Journals, Books, etc.,) | 200 |
Documents | 333 |
Average citations per doc | 2.303 |
References | 5976 |
Document Contents | |
Keywords Plus (ID) | 170 |
Author’s Keywords (DE) | 561 |
Authors | |
Authors | 932 |
Authors of single-authored docs | 86 |
Authors Collaboration | |
Single-authored docs | 99 |
Co-Authors per Doc | 3.31 |
International co-authorships % | 24.62 |
Document Types | |
Article | 119 |
Editorial material | 105 |
Letter | 85 |
Review | 14 |
News item | 9 |
Book review | 1 |
Summary of the data
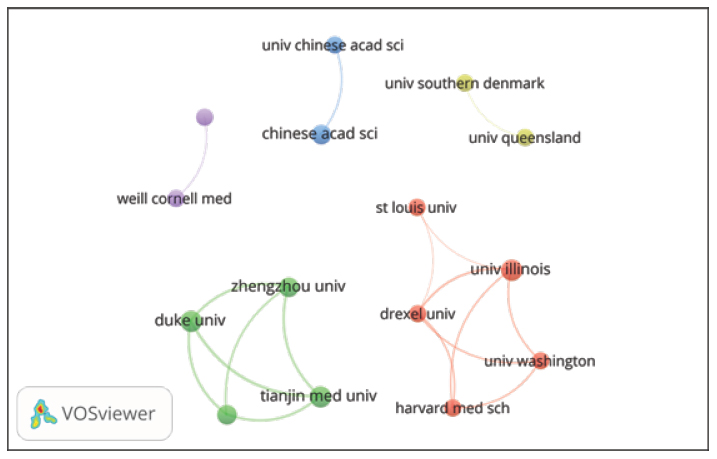
Figure 2:
Top 15 Organisations collaborations network map
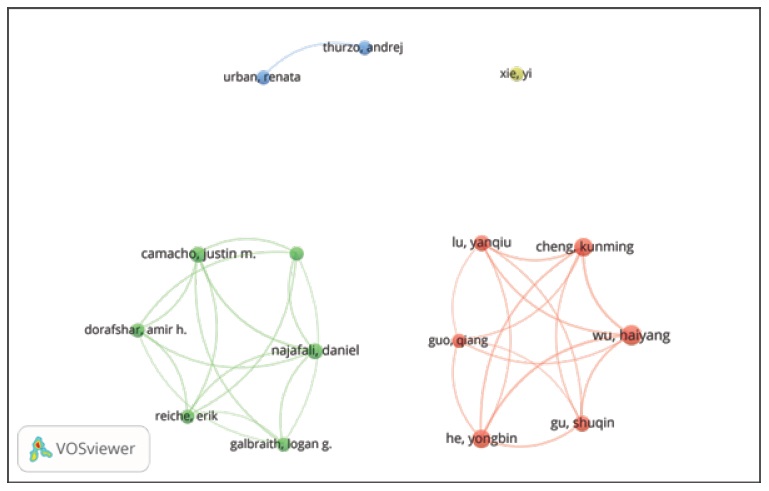
Figure 3:
Top 15 Authors collaborations network map
Nature, a highly regarded scientific journal, has also delved into ChatGPT with 9 published articles. These papers have garnered significant attention, accumulating a high TC of 244. With an impressive CPP of 27.111, these articles have been widely cited, emphasizing their importance in advancing the understanding of ChatGPT. Nature’s substantial TLS of 20 underscores its prominent position in the scientific community.
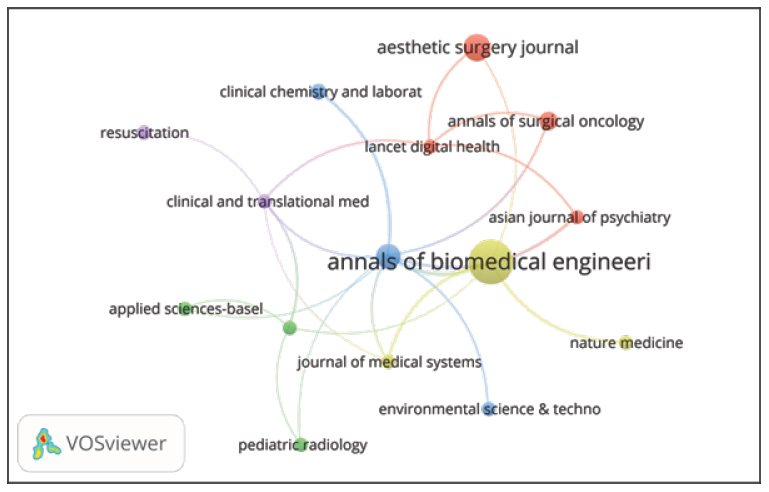
Figure 4:
Top 15 Journal Co-citations network map
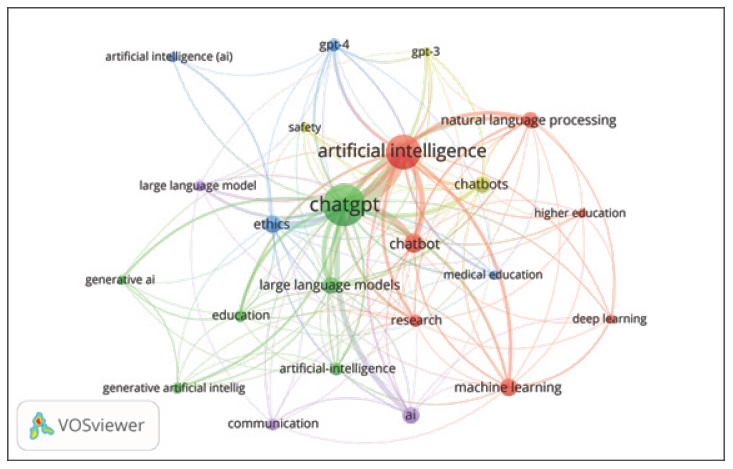
Figure 5:
Top 15 selected keywords Co-occurrence network
Other journals, such as Aesthetic Surgery Journal, Clinical Chemistry and Laboratory Medicine, and Journal of Medical Systems, have contributed with a smaller number of publications. However, they have made notable impacts within their respective domains. For instance, the Aesthetic Surgery Journal’s 11 articles have achieved a TC of 20, indicating recognition within the field. The Clinical Chemistry and Laboratory Medicine journal, with 4 articles, has achieved a reasonable CPP of 0.750, suggesting that its publications have attracted attention and contributed to the advancement of knowledge. It is important to note that the remaining journals listed in the Table 6 have also played a role in the dissemination of ChatGPT-related research. Each journal has contributed to the field to varying degrees, as indicated by their respective TC, CPP, and TLS values. Collectively, these publications provide valuable insights into the development and Applications of ChatGPT, contributing to the ongoing exploration and understanding of this technology (Figure 4 and Table 6).
Sl. No. | Country | TP | TC | CPP | HI | TLS | %TP |
---|---|---|---|---|---|---|---|
1 | USA | 111 | 208 | 1.874 | 7 | 67 | 33.234 |
2 | China | 40 | 87 | 2.175 | 4 | 32 | 11.976 |
3 | India | 26 | 34 | 1.308 | 3 | 24 | 7.784 |
4 | England | 25 | 78 | 3.12 | 4 | 33 | 7.485 |
5 | Australia | 22 | 42 | 1.909 | 4 | 26 | 6.587 |
6 | Germany | 18 | 45 | 2.5 | 3 | 27 | 5.389 |
7 | Italy | 16 | 58 | 3.625 | 3 | 25 | 5.09 |
8 | Switzerland | 14 | 59 | 4.214 | 3 | 30 | 4.192 |
9 | Netherlands | 12 | 87 | 7.25 | 4 | 25 | 3.593 |
10 | France | 9 | 32 | 3.556 | 1 | 26 | 2.695 |
11 | Spain | 8 | 25 | 3.125 | 2 | 16 | 2.695 |
12 | Austria | 7 | 16 | 2.286 | 2 | 13 | 2.096 |
13 | Sweden | 7 | 15 | 2.143 | 3 | 20 | 2.096 |
14 | Scotland | 5 | 38 | 7.6 | 3 | 13 | 1.497 |
15 | South Africa | 5 | 28 | 5.6 | 2 | 19 | 1.497 |
325 | 852 | 52.285 |
Top 15 most productive Countries in ChatGPT publications
Sl. No. | Broad Subject | TP | TC | CPP | %TP |
---|---|---|---|---|---|
1 | Surgery | 36 | 41 | 1.14 | 10.81 |
2 | Engineering Biomedical | 34 | 40 | 1.18 | 11.21 |
3 | Education Educational Research | 21 | 36 | 1.71 | 6.30 |
4 | Medicine general Internal | 18 | 36 | 2.11 | 5.40 |
5 | Computer Science Information systems | 18 | 88 | 4,89 | 5.40 |
Global Total | 333 | 880 | 6.14 |
Top 15 most productive Countries in ChatGPT publications
Keywords analysis
Table 7 provides a breakdown of keywords that have frequently occurred in ChatGPT publications. The data includes the keyword itself, and the number of times it appeared. The most prominent keyword in ChatGPT publications is “ChatGPT” itself, which occurs 103 times and has a TLS of 181. This indicates that ChatGPT is a central focus of the research, with significant attention given to this specific topic. The keyword “artificial intelligence” follows closely, appearing 71 times with a TLS of 154. This reflects the strong association between ChatGPT and the broader field of artificial intelligence. Additionally, “chatbot” is another keyword that frequently appears, with 21 occurrences and a TLS of 54, indicating the relevance of chatbot technologies within the context of ChatGPT. Ethics and machine learning emerge as important themes, both occurring 18 times, with respective TLS scores of 43 and 32. These keywords highlight the ethical considerations and the role of machine learning algorithms in the development and implementation of ChatGPT.
Other noteworthy keywords include “AI” (17 occurrences, TLS 33), “large language models” (17 occurrences, TLS 39), and “chatbots” (16 occurrences, TLS 37). These keywords underline the broader concepts and technologies surrounding ChatGPT. Furthermore, natural language processing (15 occurrences, TLS 37), research (10 occurrences, TLS 29), and GPT-4 (9 occurrences, TLS 24) are relevant keywords in the ChatGPT publications, indicating the focus on language processing techniques, the importance of research, and the exploration of future iterations of the GPT model (Figure 5, Table 7).
No | Name of the Organisations | TP | TC | CPP | TLS | %TP |
---|---|---|---|---|---|---|
Top 15 most productive organisations | ||||||
1 | Duke University, USA. | 6 | 13 | 2.167 | 16 | 1.80 |
2 | Tianjin Med University, China. | 6 | 13 | 2.167 | 16 | 1.80 |
3 | University of Illinois, USA. | 6 | 8 | 1.333 | 11 | 1.80 |
4 | Beijing Sport University, China. | 5 | 13 | 2.6 | 14 | 1.50 |
5 | Chinese Academy Science, China. | 5 | 20 | 4 | 3 | 1.50 |
6 | Zhengzhou University, China. | 5 | 9 | 1.8 | 14 | 1.50 |
7 | Drexel University, USA. | 4 | 8 | 2 | 11 | 1.20 |
8 | Harvard Med School, USA. | 4 | 9 | 2.25 | 9 | 1.20 |
9 | St Louis University, Spain. | 4 | 11 | 2.75 | 2 | 1.20 |
10 | University of the Chinese Academy of Sciences, China. | 4 | 22 | 5.5 | 3 | 1.20 |
11 | University of Queensland, Australia. | 4 | 1 | 0.25 | 1 | 1.20 |
12 | University of Southern Denmark, Denmark. | 4 | 2 | 0.5 | 1 | 1.20 |
13 | The University of Texas Medical Branch, USA. | 4 | 3 | 0.75 | 2 | 1.20 |
14 | University of Washington, USA. | 4 | 8 | 2 | 9 | 1.20 |
15 | Weill Cornell Graduate School of Medical Sciences, USA. | 4 | 41 | 10.25 | 2 | 1.20 |
Top 15 most Impactful organisations | ||||||
1 | Weill Cornell Graduate School of Medical Sciences, USA. | 4 | 41 | 10.25 | 2 | 12.31 |
2 | University of the Chinese Academy of Sciences, China. | 4 | 22 | 5.5 | 3 | 6.60 |
3 | Chinese Academy Science, China. | 5 | 20 | 4 | 3 | 6.00 |
4 | Beijing Sport University, China. | 5 | 13 | 2.6 | 14 | 3.90 |
5 | Duke University, USA. | 6 | 13 | 2.167 | 16 | 3.90 |
6 | Tianjin Med University, China. | 6 | 13 | 2.167 | 16 | 3.90 |
7 | St Louis University, Spain. | 4 | 11 | 2.75 | 2 | 3.30 |
8 | Harvard Med School, USA. | 4 | 9 | 2.25 | 9 | 2.70 |
9 | Zhengzhou University, China. | 5 | 9 | 1.8 | 14 | 2.70 |
10 | Drexel University, USA. | 4 | 8 | 2 | 11 | 2.40 |
11 | University of Washington, USA. | 4 | 8 | 2 | 9 | 2.40 |
12 | University of Illinois, USA. | 6 | 8 | 1.333 | 11 | 2.40 |
13 | The University of Texas Medical Branch, USA. | 4 | 3 | 0.75 | 2 | 0.90 |
14 | University of Southern Denmark, Denmark. | 4 | 2 | 0.5 | 1 | 0.60 |
15 | University of Queensland, Australia. | 4 | 1 | 0.25 | 1 | 0.30 |
Institutions in ChatGPT publications
Sl. No. | Author | Affiliation | TP | TC | CPP | TLS |
---|---|---|---|---|---|---|
1 | Wu, Haiyang | Anhui University of Chinese Medicine, China. | 6 | 13 | 2.167 | 21 |
2 | Cheng, Kunming | Zhengzhou University, China. | 5 | 9 | 1.800 | 19 |
3 | He, Yongbin | Beijing Sport University, China. | 5 | 13 | 2.600 | 19 |
4 | Camacho, Justin M. | Drexel University, USA. | 4 | 8 | 2.000 | 16 |
5 | Gu, Shuqin | Duke University. | 4 | 12 | 3.000 | 16 |
6 | Lu, Yanqiu | Zhengzhou University, China. | 4 | 8 | 2.000 | 16 |
7 | Najafali, Daniel | Yale University, USA. | 4 | 8 | 2.000 | 16 |
8 | Dorafshar, Amir H. | Rush University, USA. | 3 | 8 | 2.667 | 14 |
9 | Galbraith, Logan G. | Northeast Ohio Medical University, USA. | 3 | 3 | 1.000 | 12 |
10 | Guo, Qiang | Tianjin Medical University, China. | 3 | 5 | 1.667 | 13 |
11 | Morrison, Shane D. | University of Washington, USA. | 3 | 8 | 2.667 | 14 |
12 | Reiche, Erik | Harvard Medical School, USA. | 3 | 8 | 2.667 | 14 |
13 | Thurzo, Andrej | Comenius University, Bratislava. | 3 | 4 | 1.333 | 3 |
14 | Urban, Renata | Comenius University, Bratislava. | 3 | 4 | 1.333 | 3 |
15 | Xie, Yi | Peninsula Health, Australia. | 3 | 1 | 0.333 | 0 |
Authors in ChatGPT publications
Various other keywords such as “communication,” “education,” “generative artificial intelligence,” “deep learning,” “safety,” and others are also represented in the publications. These keywords reflect the diverse range of topics and applications related to ChatGPT, including communication, educational contexts, safety considerations, and the advancement of generative artificial intelligence.
DISCUSSION AND CONCLUSION
Analyzing the impact of ChatGPT using scientometrics can provide valuable insights into the reach and influence of this language model. Scientometrics is a field that employs quantitative methods to study scientific publications, citations, and other related data to evaluate the impact of research and technological advancements.14 By applying scientometric analysis to ChatGPT, we can assess its significance in terms of scholarly output, citations, and broader societal impact.
One aspect of the scientometric analysis is examining scholarly publications related to ChatGPT. This includes research papers, conference presentations, and other academic works that discuss the development, applications, and evaluations of ChatGPT. By tracking the number of publications and their distribution across different disciplines, we can gauge the level of interest and engagement from the research community.1,15
Citation analysis is another key component of scientometrics. It involves examining the citations received by ChatGPT-related publications and assessing the impact it has had on subsequent research. Higher citation counts indicate that the work is influential and has made significant contributions to the field. By analyzing the citation patterns, we can identify the most impactful publications and the areas of research that have been most influenced by ChatGPT.15
Furthermore, scientometrics can also help evaluate the societal impact of ChatGPT. This includes analyzing media coverage, public engagement, and policy discussions related to ChatGPT. By examining news articles, blog posts, and social media discussions, we can understand the public perception and discourse surrounding ChatGPT. Additionally, analyzing policy documents and guidelines can shed light on the regulatory and ethical considerations associated with deploying ChatGPT in various domains.
By conducting a scientometric analysis on ChatGPT, we can draw several conclusions. Firstly, we can assess the level of research interest and productivity in the field of language models and Natural Language Processing (NLP) that have been spurred by ChatGPT. Secondly, we can identify the most influential publications and researchers in the field, as well as the areas of research that have been most impacted by ChatGPT. Lastly, we can gain insights into the societal implications of ChatGPT, such as its potential applications, concerns, and policy considerations.
Sl. No. | Author | Affiliation | TP | TC | CPP |
---|---|---|---|---|---|
1 | Annals Of Biomedical Engineering | 30 | 38 | 1.267 | 12 |
2 | Aesthetic Surgery Journal | 11 | 20 | 1.818 | 3 |
3 | Nature | 9 | 244 | 27.111 | 20 |
4 | Annals Of Surgical Oncology | 5 | 1 | 0.200 | 4 |
5 | Clinical Chemistry and Laboratory Medicine | 4 | 3 | 0.750 | 3 |
6 | Applied Sciences-Basel | 3 | 0 | 0.000 | 2 |
7 | Asian Journal of Psychiatry | 3 | 0 | 0.000 | 5 |
8 | Clinical And Translational Medicine | 3 | 2 | 0.667 | 8 |
9 | Environmental Science and Technology | 3 | 3 | 1.000 | 2 |
10 | Journal Of MedicalSystems | 3 | 11 | 3.667 | 6 |
11 | Lancet Digital Health | 3 | 52 | 17.333 | 8 |
12 | Nature Medicine | 3 | 10 | 3.333 | 3 |
13 | Pediatric Radiology | 3 | 1 | 0.333 | 2 |
14 | Radiology | 3 | 77 | 25.667 | 5 |
15 | Resuscitation | 3 | 11 | 3.667 | 1 |
Journals in ChatGPT publications
Sl. No. | Keyword | Occurrences | TLS |
---|---|---|---|
1 | ChatGPT | 103 | 181 |
2 | Artificial intelligence | 71 | 154 |
3 | Chatbot | 21 | 54 |
4 | Ethics | 18 | 43 |
5 | Machine learning | 18 | 32 |
6 | AI | 17 | 33 |
7 | Large language models | 17 | 39 |
8 | Chatbots | 16 | 37 |
9 | Natural language processing | 15 | 37 |
10 | Research | 10 | 29 |
11 | GPT-4 | 9 | 24 |
12 | Communication | 8 | 10 |
13 | Education | 8 | 25 |
14 | Generative artificial intelligence | 7 | 15 |
15 | Large language model | 7 | 17 |
16 | Deep learning | 5 | 13 |
17 | Generative ai | 5 | 13 |
18 | GPT-3 | 5 | 19 |
19 | Higher education | 5 | 11 |
20 | Medical education | 5 | 15 |
21 | Safety | 5 | 13 |
Keywords occurred in ChatGPT publications
It is important to note that scientometric analysis is not without limitations. It relies on the availability and accuracy of data sources, and it may not capture all forms of impact, such as non-academic or non-traditional outputs. Nonetheless, by employing scientometric methods, we can gain a better understanding of the impact and significance of ChatGPT in the scientific community and beyond.
ACKNOWLEDGEMENT
The authors are thankful to Author(s) for providing financial assistance to compile this work.
ABBREVIATIONS
AI: | Artificial Intelligence |
---|---|
CPP: | Citation Per Paper |
GPT: | Generative Pre-trained Transformer |
HI: | h-Index |
ICPs: | International Collaborative Publications |
MS-Excel: | Microsoft Excel |
NLP: | Natural Language Processing |
TC: | Total Citations |
TLS: | Total link strength |
TP: | Total Publications |
UK: | United Kingdom |
USA: | United States of America |
USA: | United States of America |
VOS: | Visualization of Similarities |
WoS: | Web of Science. |
References
- Brown TB, Mann B, Ryder N, Subbiah M, Kaplan J, Dhariwal P, et al. Language models are few-shot learners. arXiv preprint arXiv. 2020 [Google Scholar]
- Radford A, Wu J, Child R, Luan D, Amodei D, Sutskever I, et al. Language models are unsupervised multitask learners. OpenAI blog. 2019;2019(8):9 [Google Scholar]
- Brown TB, Bao P, Mann B, Gao N, Fisch A, Shazeer N, et al. Unsupervised cross-lingual representation learning at scale. arXiv preprint arXiv. 2020 [Google Scholar]
- Liu Y, Ott M, Goyal N, Du J, Joshi M, Chen D, et al. RoBERTa: A robustly optimized BERT pretraining approach. arXiv preprint arXiv. 2019 [Google Scholar]
- Young T, Hazarika D, Poria S, Cambria E. Recent trends in deep learning based natural language processing [Review Article]. IEEE Comp Intell Mag. 2018;2018(3):55-75. [CrossRef] | [Google Scholar]
- Kirtania DK. ChatGPT as a tool for bibliometrics Analysis: interview with ChatGPT Three Questions and the Answers by ChatGPT. [CrossRef] | [Google Scholar]
- Prathap G. Chats with ChatGPT on h-type indices. Researchgate.Net. 2023:1-10. [CrossRef] | [Google Scholar]
- Khosravi H, Shafie MR, Hajiabadi M, Raihan AS, Ahmed I. Chatbots and ChatGPT: A bibliometric analysis and systematic review of publications in Web of Science and Scopus databases. [CrossRef] | [Google Scholar]
- Nagarkar S. ChatGPT: impact of an artificial author on bibliometrics. Indian J Med Ethics. 2023;2023(2):93-4. [PubMed] | [CrossRef] | [Google Scholar]
- Li J, Mao Y, Ouyang J, Zheng S. A review of urban microclimate research based on CiteSpace and VOSviewer analysis. Int J Environ Res Public Health. 2022;2022(8):4741 [PubMed] | [CrossRef] | [Google Scholar]
- Cobo MJ, López-Herrera AG, Herrera-Viedma E, Herrera F. An approach for detecting, quantifying, and visualizing the evolution of a research field: A practical application to the Fuzzy Sets Theory field. J Inf. 2011;2011(1):146-66. [CrossRef] | [Google Scholar]
- Small H. Co-citation in the scientific literature: a new measure of the relationship between two documents. J Am Soc Inf Sci. 1973;1973(4):265-9. [CrossRef] | [Google Scholar]
- Mora L, Deakin M, Reid A. Combining co-citation clustering and text-based analysis to reveal the main development paths of smart cities. Technol Forecasting Soc Change. 2019;142:56-69. [CrossRef] | [Google Scholar]
- Gao T, Chen X, Lin Y, Sun X. Dialogue generation with latent variable models: A survey. arXiv preprint arXiv. 2020 [CrossRef] | [Google Scholar]
- Holtzman A, Buys J, Du X, Forbes M, Bosselut A, Choi E, et al. The curious case of neural text degeneration. arXiv preprint arXiv. 2020 [CrossRef] | [Google Scholar]