ABSTRACT
ABSTRACT
Introduction:
The objective of the in silico study was to evaluate GRIN2B gene using bioinformatics tools so as to discover the probable effect of mutations of this gene as well as its protein-protein interactions with the pathobiology of depression.
Materials and Methods:
In silico analysis of SNPs of GRIN2B gene was conducted using its accession IDs and their FASTA amino acid sequences obtained from NCBI. SIFT, Polyphen-2, CADD score, MetaLR and mutation assessor were the bioinformatics softwares utilized for the study. Protein–protein interaction was assessed by string database.
Results:
Analysis of SNPs of GRIN2B gene by SIFT revealed 56.42% mutations were tolerated and 43.58% were damaging. Polyphen-2 analysis showed 46.36% SNPs were deleterious and 53.64% were benign mutations. On metaLR analysis, 7.93% of the SNPs found to be dangerous mutations whereas 92.07% of them were tolerated. CADD scores presented 2.67% damaging and 97.32% tolerant mutations of GRIN2B gene. A total of 2.46%, 39.72%, 27.3% and 30.51% of the mutations were found to be high, low, medium and neutral as per the mutation assessor tool. According to a string database analysis, there were 11 nodes, 37 edges (instead of the expected 14 edges), an average node degree of 6.73, and an average local clustering coefficient of 0.79 and protein protein interaction enrichment p-value was 1.018e-07.
Conclusion:
The results propose that GRIN2B gene mutations that are deleterious and its interactions as obtained by the bioinformatics softwares may have a crucial role the pathogenesis of depression.
INTRODUCTION
Depression is a psychiatric disease marked by a pathologically low mood (hypothymia), negative self-esteem, and unfavourable outlook on one’s present and future. The word “depression” comes from the Latin word depression, which means “gloominess, oppression”.1 In other words, with a complex etiopathogenesis based on a wide range of factors that may function at different levels, such as psychological, biochemical, genetic, and social features, major depressive disorder is a challenging and varied illness.
An ion channel and glutamate receptor found in neurons is called the N-methyl-D-aspartate receptor (often referred to as the NMDA receptor or NMDAR). The NMDA receptors are composed of a common NMDA1 sub-unit and one of the four NMDA2 sub-units (2A, 2B, 2C, or 2D), linked in an undetermined ratio to create the receptor complex.
The NMDAR-NR2B (GRIN2B) gene has 13 exons and is 419 kb in size. It is localised at 12p12. Expression of this gene is in the cerebral cortex, basal ganglia, and hippocampus.2 It was linked to alcohol consumption habits, Alzheimer’s disease, schizophrenia risk, and obsessive-compulsive disorder susceptibility.3 A silent mutation caused by the GRIN2B polymorphism C2664T in exon 13, rs1806201, results in the substitution of the codon ACT for ACC, which code for threonine. Silent mutations do not change the functional aspects of proteins, although they are not necessarily evolutionary neutral. Codons being less stringent at the third position minimizes the chances of mutations. Splicing or transcriptional regulation may be impacted by silent alterations as well.
The neuronal N-methyl-D-aspartate receptor (NMDAR) is important in the pathophysiology of schizophrenia, bipolar disorder, and depression, according to Mundo et al and Weickert et al.4–5
A SNP (Single Nucleotide Polymorphism) is a single nucleotide mutation that alters the A, T, C, or G sequence in DNA. About 90% of human genetic diversity is accounted for by SNPs. SNPs with densities ranging from 100 to 300 bases apart are present in the 3 billion-base human genome.6 SNPs have an impact on both the coding and non-coding parts of the genome. SNPs can alter how drugs interact with the body, have no effect on cell function, or cause disease, among other outcomes. Non-synonymous SNPs (nsSNPs), which cause amino acid substitutions in protein products, account for nearly half of all known genetic variants associated with human inherited diseases. This makes them particularly significant.7 However, transcription factor binding, gene expression, and splicing can all be affected by non-coding and coding synonymous SNPs (nsSNPs).8–9
SNPs should be recognized because they elicit particular traits. As a result, SNP detection is crucial. Because it requires evaluating thousands of SNPs in potential genes, this is a challenging endeavor.10 Choosing which SNPs to include in a study examining the significance of SNPs in disease is always a challenging decision. Bioinformatics prediction algorithms may be used to isolate functional and neutral SNPs under these conditions. It can also provide an explanation for mutations’ structural aspects. Simply put, bioinformatics strategies arrange SNPs in order of their functional importance.11–12
When conducting in silico genetic analysis, bioinformatics methods eliminate the need to screen a large number of individuals in order to locate genetic disease associations with sufficient statistical significance. As a result, these techniques enable SNP preselection.10 Before using wet experimental methods, it is very convenient to be able to distinguish disease-associated SNPs from neutral SNPs. When subsequent independent studies fail to identify the disease state, in silico analysis is helpful.11 Consequently, independent evidence of the SNP function that has been discovered through the use of predictive algorithms can be used in conjunction with additional resources to distinguish between true positives and false positives.
The relationship between the GRIN2B gene and depression may be established through in silico analysis. The goal of the proposed study was to look at all GRIN2B SNPs that are caused by missense mutations that are linked to depression. The mutation’s structural foundation is also examined in this investigation. These bioinformatics tools are merely tools for putting SNPs in order of importance to their functions. For silico genetic analysis, bioinformatics software has the potential to study gene-disease associations at a statistically significant level without having to study a significant number of people. In a nutshell, these methods can be used for SNP preselection.
The objective of the in silico study was to evaluate GRIN2B gene using bioinformatics softwares, sorting the intolerant from tolerant (SIFT), Polyphen-2, CADD, metaLR, mutation assessor and protein-protein interactions (PPIs) by string database so as to identify the probable deleterious effect of mutations as well as protein-protein interactions of these genes in the pathobiology of depression.
MATERIALS AND METHODS
Evaluation of the Functional Impact of Coding nsSNPs Using a Sorting Intolerant from Tolerant (SIFT) Sequence Homology Tool
SIFT can be accessed at sift.jcvi.org.13 Query sequences are analyzed and various alignment information are used to predict harmful substitutions at each point of the query sequence. This involves, for a given protein sequence, first searching for related sequences, then selecting closely related sequences that may have similar functions, and then multiplexing these selected sequences. It is a multi-step process that obtains alignments and finally computes the normalized probabilities of all potential permutations. Intolerant or damaging substitutions are predicted to occur when the normalised probability is less than 0.05; tolerated substitutions are predicted to occur when the normalised probability is more than 0.05.14
By letting the method run with its default options (UniProt- TrEMBL 39.6 database, average sequence conservation of 3.00, sequences with >90% match to the query sequence), homologous sequences were found for the study. The capacity to remove remains unchanged). The SIFT method assesses if changes in amino acids have an impact on how proteins function. It operates by taking use of the sequence homology between related genes and domains and the physico-chemical properties of amino acid residues. The total number of non-intronic missense mutations, rs numbers, and SNP locations on the GRIN2B chromosome were recorded in an analysis-friendly format using the Sort the Intolerant from Tolerant online software (SIFT).We used the FASTA amino acid sequence from the NCBI protein accession ID of GRIN2B as a query sequence to analyze the filtered nsSNPs from the dbSNP database.
Assessment of nsSNPs using polyphen
The technique called PolyPhen-2 (Polymorphism Phenotyping v2) makes use of elementary physical and comparative principles to forecast the effects of amino acid alterations on the structure and function of human proteins.
Both the SIFT and Polyphen tools use amino acid sequences obtained by the protein accession ID of the GRIN2B gene.
Combined Annotation Dependent Depletion (CADD)
CADD is a technique for evaluating how harmful insertion-deletion variations and single nucleotide variants are to the human genome.
Despite the availability of numerous alternative annotation and scoring systems, most annotations tend to focus on a specific information category (such as conservation) and/or have a narrow range of use. As a result, a widely useful statistic that impartially incorporates several pieces of information is required. By comparing variations that survived natural selection with simulated mutations, the Combined Annotation Dependent Depletion (CADD) system unifies several annotations into one metric.
C-scores rank causal variations inside specific genome sequences and correlated with regulatory effects measured experimentally, pathogenicity of both coding and non-coding variants, and allelic diversity.
In Genome-Wide association studies (GWAS), complex trait-associated variant C-scores were significantly higher than corresponding control C-scores and correlated with study sample size, indicating greater precision in larger GWAS.
MetaLR
In order to predict the virulence of missense variants, MetaLR employs logistic regression to combine allele frequency data and nine independent variant hazard scores. Variants are categorized as “harmful” or “acceptable.” Additionally, it receives a score of 0 to 1, with variants with higher scores being more likely to be harmful.
Mutation assessor
Mutation assessment software predicts the functional impact of amino acid substitutions in proteins based on the evolutionary conservation of affected amino acids in protein homologues.
Protein protein interaction was carried out using string database, enrichment was done by GO enrichment.
RESULTS
A total of 934 nsSNPs were filtered and analysed. SIFT analysis of the GRIN2B gene’s SNPs found that 43.58% of the mutations were harmful while 56.42% were tolerable. According to Polyphen-2 study, 53.64% of SNPs were benign mutations whereas 46.36% of them were harmful. On the basis of the metaLR analysis, 7.93% of the SNPs were determined to have harmful mutations, whereas 92.07% of them were tolerated. The GRIN2B gene showed 2.67% harmful and 97.32% tolerant alterations according to CADD scores. According to the mutation assessor tool, a total of 2.46%, 39.72%, 27.3%, and 30.51% of the mutations were determined to be high, low, medium, and neutral. Results of effect prediction is displayed in Table 1.
SIFT | Polyphen-2 | CADD | MetaLR | Mutation Assessor | |||||
---|---|---|---|---|---|---|---|---|---|
Deleterious low | 30 | Benign | 501 | Deleterious | 25 | Damaging | 74 | High | 23 |
Deleterious | 377 | Low | 371 | ||||||
Tolerated low | 57 | Possibly damaging | 433 | Benign | 909 | Tolerated | 860 | Medium | 255 |
Tolerated | 470 | Neutral | 285 |
On string database analysis, number of nodes were 11, edges 37 (versus expected number of edges 14), average node degree 6.73, average local clustering coefficient 0.79 and protein protein interaction enrichment p value was 1.018e-07 (Figure 1). Proteins are the nodes of the network. The hypothesised functional relationships are represented by the edges. An edge was drawn in evidence mode with seven lines of varying colours; lines indicate the presence of the seven various categories of evidence that were considered in anticipating the relationships as follows (Figure 1).
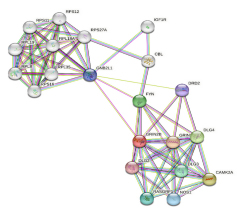
Figure 1:
Protein-protein interaction by string database.
Red line – indicates the presence of fusion evidence
- Green line – neighbourhood evidence
- Blue line – cooccurrence evidence
- Purple line – experimental evidence
- Yellow line – text mining evidence
- Light blue line – database evidence
- Black line – co-expression evidence.
Enrichment processes biological, cellular or molecular were statistically insignificant. Only interactions with a confidence score greater than the lowest needed interaction score are involved in the predicted network. More engagement but higher false positives are associated with lower scores. The possibility of a predicted association between two proteins of the metabolic pathway in the database actually exists is represented by the confidence score. Following are the bounds of confidence:
- Low confidence – 0.15 (or better),
- Medium confidence – 0.4
- High confidence – 0.7
- Highest confidence – 0.9
Interaction results by string database are given in Table 2.
Nodel | Node 2 | Neighb ourhood On chrom osome | Gene fusion | Phylogen etic Co-occurence | Homology | Co-expression | Experimental interaction | Database annotated | Automated textmining | Combined score |
---|---|---|---|---|---|---|---|---|---|---|
CAMK2A | GRIN1 | 0 | 0 | 0 | 0 | 0.378 | 0.631 | 0.5 | 0.625 | 0.951 |
CAMK2A | DLG3 | 0 | 0 | 0 | 0 | 0.062 | 0.299 | 0.5 | 0.354 | 0.759 |
CAMK2A | DLG2 | 0 | 0 | 0 | 0 | 0.231 | 0.241 | 0.5 | 0.397 | 0.8 |
CAMK2A | RASGRF1 | 0 | 0 | 0 | 0 | 0.239 | 0 | 0.5 | 0.226 | 0.68 |
CAMK2A | NOS1 | 0 | 0 | 0 | 0 | 0.096 | 0.213 | 0.8 | 0.051 | 0.846 |
CAMK2A | DLG4 | 0 | 0 | 0 | 0 | 0.17 | 0.247 | 0.5 | 0.583 | 0.852 |
CAMK2A | GRIN2B | 0 | 0 | 0 | 0 | 0.231 | 0.991 | 0.5 | 0.904 | 0.999 |
DLG2 | FYN | 0 | 0 | 0 | 0 | 0.062 | 0.07 | 0 | 0.704 | 0.72 |
DLG2 | GRIN1 | 0 | 0 | 0 | 0 | 0.231 | 0.426 | 0.5 | 0.707 | 0.926 |
DLG2 | DLG3 | 0 | 0 | 0 | 0.965 | 0.083 | 0.182 | 0.8 | 0.9 | 0.842 |
DLG2 | NOS1 | 0 | 0 | 0 | 0.541 | 0.083 | 0.491 | 0 | 0.54 | 0.63 |
DLG2 | RASGRF1 | 0 | 0 | 0 | 0 | 0.158 | 0 | 0.5 | 0.279 | 0.67 |
DLG2 | DLG4 | 0 | 0 | 0 | 0.967 | 0.118 | 0.501 | 0.3 | 0.982 | 0.676 |
DLG2 | GRIN2B | 0 | 0 | 0 | 0 | 0.16 | 0.723 | 0.5 | 0.909 | 0.988 |
DLG3 | FYN | 0 | 0 | 0 | 0 | 0 | 0.07 | 0 | 0.416 | 0.434 |
DLG3 | GRIN1 | 0 | 0 | 0 | 0 | 0.096 | 0.426 | 0.5 | 0.815 | 0.945 |
DLG3 | RASGRF1 | 0 | 0 | 0 | 0 | 0.062 | 0 | 0.5 | 0.262 | 0.623 |
DLG3 | DLG4 | 0 | 0 | 0 | 0.965 | 0.063 | 0.598 | 0.8 | 0.975 | 0.92 |
DLG3 | GRIN2B | 0 | 0 | 0 | 0 | 0.062 | 0.807 | 0.5 | 0.989 | 0.998 |
DLG4 | FYN | 0 | 0 | 0 | 0 | 0.089 | 0.501 | 0 | 0.99 | 0.995 |
DLG4 | DRD2 | 0 | 0 | 0 | 0 | 0.09 | 0.182 | 0 | 0.502 | 0.597 |
DLG4 | GRIN1 | 0 | 0 | 0 | 0 | 0.23 | 0.99 | 0.8 | 0.966 | 0.999 |
DLG4 | RASGRF1 | 0 | 0 | 0 | 0 | 0.12 | 0 | 0.5 | 0.256 | 0.644 |
DLG4 | GRIN2B | 0 | 0 | 0 | 0 | 0.091 | 0.992 | 0.8 | 0.99 | 0.999 |
DLG4 | NOS1 | 0 | 0 | 0 | 0 | 0.063 | 0.698 | 0.9 | 0.989 | 0.999 |
DRD2 | GNB2L1 | 0 | 0 | 0 | 0 | 0 | 0 | 0 | 0.447 | 0.447 |
DRD2 | GRIN1 | 0 | 0 | 0 | 0 | 0.121 | 0.078 | 0 | 0.767 | 0.794 |
DRD2 | GRIN2B | 0 | 0 | 0 | 0 | 0.063 | 0.057 | 0 | 0.987 | 0.988 |
FYN | GRIN1 | 0 | 0 | 0 | 0 | 0.062 | 0.4 | 0.5 | 0.725 | 0.912 |
FYN | GNB2L1 | 0 | 0 | 0 | 0 | 0 | 0.496 | 0 | 0.987 | 0.993 |
FYN | GRIN2B | 0 | 0 | 0 | 0 | 0.062 | 0.685 | 0.9 | 0.951 | 0.998 |
GNB2L1 | GRIN2B | 0 | 0 | 0 | 0 | 0 | 0.281 | 0 | 0.985 | 0.989 |
GRIN1 | RASGRF1 | 0 | 0 | 0 | 0 | 0.276 | 0 | 0.5 | 0.426 | 0.774 |
GRIN1 | NOS1 | 0 | 0 | 0 | 0 | 0.063 | 0.394 | 0.8 | 0.819 | 0.976 |
GRIN1 | GRIN2B | 0 | 0 | 0 | 0.697 | 0.269 | 0.992 | 0.9 | 0.993 | 0.999 |
GRIN2B | RASGRF1 | 0 | 0 | 0 | 0 | 0.13 | 0.27 | 0.5 | 0.988 | 0.995 |
GRIN2B | NOS1 | 0 | 0 | 0 | 0 | 0.096 | 0.393 | 0.8 | 0.943 | 0.993 |
The SIFT scale runs from 0 to 1. Single nucleotide polymorphisms with a SIFT score of lower than or equal to 0.05 are deemed harmful, whereas those with a value higher than that are deemed tolerable. The optimal range for the median information is between 2.75 and 3.5. This is used to gauge the diversity of the prediction sequences. An indicator indicating the prediction was based on closely similar sequences is a number larger than 3.25. The number of sequences at a certain place is known as the number of sequences at prediction. SIFT software picks sequences spontaneously, however if the alteration occurs at the start or termination of the protein, there might only be a small number of sequences represented there, as this column demonstrates.
The PolyPhen tool also looked at the SNPs. Scores between 0.0-0.15 indicate a benign mutation, 0.15 and 1.0 indicate potential damage, and 0.85 and 1.0 indicate certain, expected damage.
DISCUSSION
Computational studies on the functional effects of SNPs in this gene have not yet been carried out, despite numerous studies demonstrating a link between SNPs in this gene and a variety of diseases. To determine whether amino acid changes affect protein function, SIFT technology uses sequence homology and the physico-chemical properties of amino acid residues between related genes and domains throughout evolution. SIFT has an estimated “false positive” and “false negative” error rate of 20% and 31%, respectively. In benchmark studies using amino acid substitutions believed to have a significant negative effect on the mutant protein’s residual activity as a test set, SIFT has demonstrated approximately 80% success. However SIFT and polyphen are of great hep in predicting the effects of mutation on protein function and the need to assess the gene polymorphisms by wet lab procedures.
CADD Scores below 30 are regarded as “likely benign,” whereas scores above 30 are considered “likely harmful.” The 0.1% of the most harmful potential substitutions in the human genome are projected to be those variants with scores > 30.
MetaLR displays the likelihood, which can be any of “neutral,””low,””medium,” or “high,” as well as the rank score, which ranges from 0 -1, with greater scores indicating variations that are more expected to have negative effects.
The mutation assessor scale ranges from 0 to 1, with higher ratings indicating more detrimental potential.
There is evidence to suggest that NMDAR signalling may have a role in the aetiology of emotional disorders. Bipolar disorder and major depressive disorder, according to Coyle and Javitt et al., are linked to altered levels of central excitation neurotransmitters.15–16 According to Kristiansen et al., hypothesis, patients with mood disorders have decreased NMDAR expression, distribution, and function.17 According to a research by Ibrahim et al, patients may benefit therapeutically from NMDAR modulators.18 Antidepressants and mood stabilisers have been shown to improve NMDAR function, according to studies by Berman and colleagues. And Methew and colleagues.19–20 Their preliminary results support the idea that GRIN2B functions as a genetic predictor of treatment resistant depression in people with major depressive disorder.21 In addition to conferring vulnerability to it.
Protein-protein interactions (PPIs) are crucial for predicting target protein function and drug-like properties of molecules. Cell-to-cell interactions, metabolic and developmental processes regulation are some of the biological events controlled by protein-protein interactions (PPIs).22 Finding knowledge about protein-protein interactions aids in the selection of therapeutic targets.23 Families of enzymes, transcription factors, and intrinsically disordered proteins, among others, have been shown in studies to be proteins with more connections (hubs).24–25 PPIs, however, have a wider regulatory scope and more complex processes involved. One must recognise different interactions and ascertain the effects of the interactions in order to more fully comprehend their significance in the cell. In silico approach is one of the methods to analyse PPIs. The “functional association,” or link between two proteins that both collectively contribute to a particular biological function, is the fundamental interaction unit in STRING On analysis, there were insignificant gene co-expression, co-occurrence, fusions for GRIN2B gene. Results of string database analysis are represented in Table 2. Along with p53 gene, other genes interacting with it may also have a role in the pathobiology of depression. Thus genes involved in NMDAR signalling may be important genetic regulators of human physiology, and consequently influence mood disorders.
CONCLUSION
The current in silico analysis, intended to discover the role of GRIN2B gene polymorphisms-their interactions in the pathobiology of depression suggests strongly that deleterious effects of mutations of this gene as well as protein-protein interactions influence the pathobiology of depression. The bioinformatics study findings may be useful in exploring therapeutic targets for depression.
Cite this article: Adiga U, Adiga S, Rai T, Desy TM, Honnalli NM. Bioinformatics Approach to Evaluate GRIN2B Gene in Depression -An in silico Study. Int. J. Pharm. Investigation. 2023;13(4):852-7.
ABBREVIATIONS
GRIN2B | Glutamate Ionotropic Receptor NMDA Type Sub-unit 2B |
---|---|
NMDA | N-methyl-D-aspartate receptor |
NsSNPs: | Nonsynonymous SNPs |
SIFT: | sorting the intolerant from tolerant |
CADD: | Combined Annotation Dependent Depletion |
GWAS: | Genome-Wide Association Studies. |
References
- Smulevich. Depression in general practice. 2000
- Schito AM, Pizzuti A, Di Maria E, Schenone A, Ratti A, Defferrari R, et al. mRNA distribution in adult human brain of GRIN2B, a N-methyl-D-aspartate (NMDA) receptor sub-unit. Neurosci Lett. 1997;239(1):49-53. [PubMed] | [CrossRef] | [Google Scholar]
- Li D, He L. Association study between the NMDA receptor 2B sub-unit gene (GRIN2B) and schizophrenia: A HuGE review and meta-analysis. Genet Med. 2007;9(1):4-8. [PubMed] | [CrossRef] | [Google Scholar]
- Mundo E, Tharmalingham S, Neves-Pereira M, Dalton EJ, Macciardi F, Parikh SV, et al. Evidence that the N-methyl-D-aspartate sub-unit 1 receptor gene (GRIN1) confers susceptibility to bipolar disorder. Mol Psychiatry. 2003;8(2):241-5. [PubMed] | [CrossRef] | [Google Scholar]
- Weickert CS, Fung SJ, Catts VS, Schofield PR, Allen KM, Moore LT, et al. Molecular evidence of N-methyl-D-aspartate receptor hypofunction in schizophrenia. Mol Psychiatry. 2013;18(11):1185-92. [PubMed] | [CrossRef] | [Google Scholar]
- Lee JE, Choi JH, Lee JH, Lee MG. Gene SNPs and mutations in clinical genetic testing: haplotype-based testing and analysis. Mutat Res. 2005;573(1-2):195-204. [PubMed] | [CrossRef] | [Google Scholar]
- Krawczak M, Ball EV, Fenton I, Stenson PD, Abeysinghe S, Thomas N, et al. Human gene mutation database—a biomedical information and research resource. Hum Mutat. 2000;15(1):45-51. [PubMed] | [CrossRef] | [Google Scholar]
- Prokunina L, Alarcón-Riquelme ME. Regulatory SNPs in complex diseases: their identification and functional validation. Expert Rev Mol Med. 2004;6(10):1-15. [PubMed] | [CrossRef] | [Google Scholar]
- Stenson PD, Mort M, Ball EV, Howells K, Phillips AD, Thomas NS, et al. The human gene mutation database: 2008 update. Genome Med. 2009;1(1):13 [PubMed] | [CrossRef] | [Google Scholar]
- Ramensky V, Bork P, Sunyaev S. Human non-synonymous SNPs: server and survey. Nucleic Acids Res. 2002;30(17):3894-900. [PubMed] | [CrossRef] | [Google Scholar]
- Emahazion T, Feuk L, Jobs M, Sawyer SL, Fredman D, St Clair D, et al. SNP association studies in Alzheimer’s disease highlight problems for complex disease analysis. Trends Genet. 2001;17(7):407-13. [PubMed] | [CrossRef] | [Google Scholar]
- Schork NJ, Fallin D, Lanchbury JS. Single nucleotide polymorphisms and the future of genetic epidemiology. Clin Genet. 2000;58(4):250-64. [PubMed] | [CrossRef] | [Google Scholar]
- Ng PC, Henikoff S. SIFT: Predicting amino acid changes that affect protein function. Nucleic Acids Res. 2003;31(13):3812-4. [PubMed] | [CrossRef] | [Google Scholar]
- Ng PC, Henikoff S. Predicting the effects of amino acid substitutions on protein function. Annu Rev Genomics Hum Genet. 2006;7:61-80. [PubMed] | [CrossRef] | [Google Scholar]
- Coyle JT. The glutamatergic dysfunction hypothesis for schizophrenia. Harv Rev Psychiatry. 1996;3(5):241-53. [PubMed] | [CrossRef] | [Google Scholar]
- Javitt DC, Zukin SR. Recent advances in the phencyclidine model of schizophrenia. Am J Psychiatry. 1991;148(10):1301-8. [PubMed] | [CrossRef] | [Google Scholar]
- Kristiansen LV, Huerta I, Beneyto M, Meador-Woodruff JH. NMDA receptors and schizophrenia. Curr Opinionin Pharmacol. 2007;Array(1):48-55. [PubMed] | [CrossRef] | [Google Scholar]
- Ibrahim L, Diaz Granados N, Jolkovsky L, Brutsche N, Luckenbaugh DA, Herring WJ, et al. Arandomized, placebo-controlled, crossover pilot trial of the oral selective NR2Bantagonist MK 0657 in patients with treatment – resistant major depressive disorder. J Clin Psychopharmacol. 2012;32(4):551-7. [PubMed] | [CrossRef] | [Google Scholar]
- Berman RM, Cappiello A, Anand A, Oren DA, Heninger GR, Charney DS, et al. Antidepressant effects of ketamine in depressed patients. Biol Psychiatry. 2000;47(4):351-4. [PubMed] | [CrossRef] | [Google Scholar]
- Mathew SJ, Murrough JW, aan het Rot MA, Collins KA, Reich DL, Charney DS, et al. Riluzole for relapse prevention following intravenous ketamine in treatment-resistant depression:a pilot randomized,placebo-controlled continuation trial. Int J Neuropsychopharmacol. 2010;13(1):71-82. [PubMed] | [CrossRef] | [Google Scholar]
- Zhang C, Li Z, Wu Z, Chen J, Wang Z, Peng D, et al. A study of N-methyl-D-aspartate receptoer gene (GRIN2B) variants as predictors of treatment resistant major depression. Psychopharmacology. 2014;231(4):685-93. [PubMed] | [CrossRef] | [Google Scholar]
- Braun P, Gingras AC. History of protein-protein interactions: from egg-white to complex networks. Proteomics. 2012;12(10):1478-98. [PubMed] | [CrossRef] | [Google Scholar]
- Pedamallu CS, Posfai J. Open source tool for prediction of genome wide protein-protein interaction network based on ortholog information. Source Code Biol Med. 2010;5 [PubMed] | [CrossRef] | [Google Scholar]
- Dunker AK, Cortese MS, Romero P, Iakoucheva LM, Uversky VN. Flexible nets: the roles of intrinsic disorder in protein interaction networks. FEBS Journal. 2005;272(20):5129-48. [PubMed] | [CrossRef] | [Google Scholar]
- Sarmady M, Dampier W, Tozeren A. HIV protein sequence hotspots for crosstalk with host hub proteins. PLOS ONE. 2011;6(8) [PubMed] | [CrossRef] | [Google Scholar]