ABSTRACT
Background
Cardiovascular diseases comprehend a array of situations that disturb the heart and blood vessels, making them a principal cause of morbidity and mortality worldwide. Dyslipidemia, an imbalance in lipids, such as cholesterol, Low-Density Lipoprotein Cholesterol (LDL-C), triglycerides and High-Density Lipoprotein (HDL), is a significant risk factor for cardiovascular disease and contributes to numerous deaths annually. The development of cholesterol-degrading drugs, known as statins, began in the mid-1970s and their effects on intracellular processes have been well documented.
Materials and Methods
Fluvastatin, a statin widely used in cardiovascular disease, inhibits hydroxymethyl glutaryl-coenzyme A, a vital enzyme in cholesterol biosynthesis. This study aimed to discover new therapeutic drugs to prevent cardiovascular diseases by deriving new compounds from fluvastatin.
Results
The investigation involved several processes including derivative generation, molecular docking, ADME analysis, PASS prediction, rodent toxicity assessment and identification of possible adverse effects. After evaluating a vast dataset of 194 derivatives, compounds that met stringent criteria across all parameters were selected.
Conclusion
Two promising compounds from this filtered set were further assessed through Molecular Dynamics (MD) simulation and Principal Component Analysis (PCA), along with the control.
INTRODUCTION
Cardiovascular Diseases (CVDs), a broad term encompassing conditions affecting the heart and blood vessels, pose a significant global health threat, leading to millions of deaths worldwide. CVDs are categorized as noncommunicable diseases, contributing to a decline in overall life expectancy (Sadeghiet al., 2017). The global prevalence of CVD is on the rise and key risk factors include obesity, hypertension, dyslipidemia and Diabetes Mellitus (DM) (Mathers and Loncar, 2006). Unhealthy lifestyles, physical inactivity, smoking, alcohol consumption, aging, and a family history of cardiovascular diseases are major contributors to the risk of CVD (Despréset al., 2008; Martirosyanet al., 2007; Ordovas, 2006). Dyslipidemia, characterized by abnormal blood lipid levels, is a key modifiable risk factor for CVD. It involves elevated Total Cholesterol (TC), Low-Density Lipoprotein Cholesterol (LDL-C), and Triglycerides (TG) levels, along with decreased High-Density Lipoprotein Cholesterol (HDL-C) concentration (Wuet al., 2018; Klopet al., 2013; Guzzaloniet al., 2000). Dyslipidemia significantly influences the development and progression of atherosclerosis, a condition associated with stroke, heart failure, myocardial infarction and kidney failure. Factors such as elevated LDL cholesterol, low HDL cholesterol, and high triglyceride concentrations are connected with an increased cardiovascular risk (O’Mearaet al., 2004; Joshiet al., 2014; Brownet al., 2000; Hadaeghet al., 2006).
As individuals age, the prevalence of dyslipidemia tends to rise (Hedayatniaet al., 2020). The primary diagnostic method for dyslipidemia is a fasting lipid panel that includes measurements of total cholesterol, LDL, HDL, and triglycerides. The age at which dyslipidemia screening should commence remains a matter of debate. Furthermore, genetic factors can contribute to dyslipidemia, with familial hypercholesterolemia as a notable example. Despite advancements in cardiovascular medicine,
CVDs remains a growing public health concern, particularly in developing and economically challenged nations. Individuals at moderate to high risk of developing cardiovascular diseases are often prescribed statins, with atorvastatin being a commonly used medication among various statins (Grundy and Stone, 2019; Andersonet al., 2016). The rising prevalence of cardiovascular illnesses such as heart attack, angina and artery disease has contributed significantly to the increased use of this class of medications (Mihaylovaet al., 2012; Tayloret al., 2013). Some studies have reported that statins reduce both cholesterol levels and CVD risk with no side effects. Unlike various individuals from the statin group, atorvastatin has proven to be a functional compound and does not require any attention to activate.
HMG-CoA reductase plays a major role in cholesterol regulation. HMG-CoA reductase was the first enzyme to be involved in the mevalonate pathway, which is considered to be responsible for the rate-limiting step of cholesterol production. Statins competitively inhibited HMG-CoA reductase. Fluvastatin, an HMG-CoA reductase inhibitor treated as an inhibiting drug compound was discovered at about the same time as atorvastatin with greater efficacy (Sikorski, 2007). In individuals who grew up, fluvastatin was prescribed from person to person with hypercholesterolemia and mixed dyslipidemia to diminish cholesterol levels in the bloodstream. In particular, this medication lowers low-density lipoprotein cholesterol and triglycerides while extending high-density lipoproteins. From every aspect of view, the inhibitory activity of HMG-CoA enzyme has been proven to reduce cholesterol synthesis, resulting in an increase in the liver’s absorption of low-density lipids via LDL receptor modification. More explicitly, statin prescriptions were proven to seriously repress HMG-CoA reductase (Moghadasian, 1999), which catalyzes the conversion of HMG-CoA to mevalonic acid (Liao and Laufs, 2005). The impact of dyslipidemia can shift from one individual to another and geographical area, depending on age, illness, climate, dietary routine, and lifestyle factors. This study demonstrated that the synergistic action of dyslipidemia and other CVD risk factors in cardiac patients accelerates the progression of atherosclerosis, cardiovascular morbidity, and death. Recognizing the probable cause of dyslipidemia in patients with CVD is pivotal in dealing with the illness and decreasing further complexities. Therefore, the study aimed to identify fluvastatin derivatives to prevent CVDs with minimal side effects, utilizing the advancement of bioinformatics tools and databases to focus on derivatives as new pharmaceutical alternatives for better and safer control of cardiovascular disorders. The analysis was based on distinct parameters, including pharmacokinetics, drug-likeliness features, toxicity profiling assessment, molecular interactions and molecular dynamics. The protein chosen for the study was HMG-CoA reductase with atorvastatin (PDB ID 1HWK). Using these leveraging bioinformatics techniques, we were able to thoroughly assess research contributing to gaining an effective compound (Mihaylovaet al., 2012; Tayloret al., 2013; Sikorski, 2007; Moghadasian, 1999; Liao and Laufs, 2005).
MATERIALS AND METHODS
Retrieval and preparation of target protein
The target protein, 1HWK, with a resolution of 2.22 Angstroms, was gained from the Protein Data Bank in PDB format, which was sourced from rcsb.org (Domínguezet al., 2021). Afterwards, the macromolecule was prepared, which involved the removal of water particles and the formation of hydrogen bonds, using the BIOVIA Discovery Studio Visualizer 2021 (Singhet al., 2021).
Bioisosteric substitution and preparation of ligands database
Retrieving the parent compound in standard SMILE format was the starting point. To generate the primary molecule, the authoritative SMILE ID was inputted into the web-based tool MolOpt (Modeeet al., 2023). Subsequently, the bio isosteric substitution technique was applied, in which the functional groups of molecules were exchanged to enhance their potency and efficacy (Dick and Cocklin, 2020). The data mining (fast) replacement technique provided rules for drawing derivatives. The server automatically generated derivatives with SMILE IDs, which were then converted into the PDB format using the ChemDB chemoinformatic gateway for further analysis (Langdonet al., 2010).
Virtual Screening
Molecular docking procedures were conducted using DockThor, a widely recognized and versatile docking web server (Santoset al., 2020), to evaluate the binding affinity of the acquired ligand analogs in comparison to the control. The proteins and analogs were carefully uploaded to ensure a smooth execution. The binding pocket was defined and other parameters, such as docking runs and evaluations per docking, remained unchanged. For individual runs, the server provides the best pose based on binding energies and clustering probability. The results were retrieved and saved in an Excel file for in-depth analysis. Biovia Discovery Studio was used to identify the most favorable compound interactions. The DockThor score, similar to delta G, was used to measure the binding affinity and rank different ligand molecules (Santoset al., 2020; De Magalhãeset al., 2014).
Original Ligand Name | Coded Ligand Name | Binding Affinity (kcal/mol) |
---|---|---|
control.pdb | ligand_e787dfc97e | -7.04 |
mol_000.pdb | ligand_6f4099b31b | -6.92 |
mol_001.pdb | ligand_ef3441670f | -6.89 |
mol_002.pdb | ligand_068ab1 | -7.00 |
mol_003.pdb | ligand_46a0627 | -8.00 |
mol_004.pdb | ligand_a1096914f4 | -7.52 |
mol_005.pdb | ligand_a61ae1016 | -7.37 |
mol_008.pdb | ligand_62c5696a2a | -7.83 |
mol_012.pdb | ligand_bbd5403f04 | -7.75 |
mol_014.pdb | ligand_f1ef0b | -7.50 |
Evaluation of drug-likeness and pharmacokinetics predictions
In evaluating the pharmacokinetic properties of drugs, ADMET examines various parameters such as surface area, number of hydrogen bond donors and BBB permeation. Profiling is a crucial step in drug development and highlights its importance (Singhet al., 2021; Azzam, 2023). Profiling helps to identify compounds with drug-like properties and favorable pharmacokinetics, indicating their potential as drug candidates. To ensure the accuracy of our results, we employed Swiss ADME (Brenket al., 2008; Mueggeet al., 2001; Dainaet al., 2017; Lipinski, 2004) and pkCSM (Ze-jun et al., 2023; Pireset al., 2015). prediction tools.
In silico Prophecy of Activity Spectra for compounds (PASS)
The PASS prediction tool was utilized to investigate a wide array of biological activities, such as antifungal, antidote and antiviral effects (Dmitrievet al., 2019). (https://www.way2drug.com/passonline/index.php). First, the compound structures were transformed into the SMILES format using the online tool Chemdb. These data were then uploaded to the system to analyze the mass spectrum activities. The values were determined based on the principles of Pa and Pi, which represent the probability of active and inactive molecules, respectively. For a compound to be deemed acceptable, its Pa and Pi values must fall within the range of 0.000-1.00, with Pa being greater than Pi.
Anticipating compound toxicity and side effects
To predict severe acute toxicity and potential secondary effects in rats, researchers have used the online tools GUSAR and ADVER-Pred. GUSAR (http://www.way2drug.com/gusar/) (Lagunin et al., 2011) was employed to predict rat toxicity based on different routes of administration, including intraperitoneal, intravenous, oral and subcutaneous. Meanwhile, ADVER-PRED (http://www.way2drug.com/adverpred) (Ivanovet al., 2018) was utilized to determine potential side effects.
Performing Molecular Dynamics
Molecular Dynamics has emerged as a valuable approach for understanding the atomic-level chemistry of biological macromolecules (Khanet al., 2018). Through rigorous analysis of various trajectories, each parameter was thoroughly examined, leading to refinement of the compounds. The top-hit compounds underwent Molecular Dynamics (MD) simulation studies conducted at 300 K using GROMACS software version 5.1.2 (Stenberg and Stenqvist, 2020). The gmx grep module of the GROMACS package was utilized to extract and compile complexes and topologies. The affected compounds were subjected to a force field CGenFF (CHARMm General Force Field) (Fischeret al., 2015). Subsequently, the TIP3 model was employed to solvate each complex within a cubic box. After applying the boundary conditions, salts were introduced to equilibrate the charges. The framework underwent 25,000,000 steps using the steepest descent algorithm for energy minimization. The temperature was increased while maintaining the stable NVT and NPT conditions. Following the equilibration phase, a mesh was generated using the Ewald method (Grantet al., 2006; Petersen, 1995). The simulation lasted for 10 ns, providing in-depth insights into submolecular dynamics (Yu and Dalby, 2020; Zhanget al.,2020).
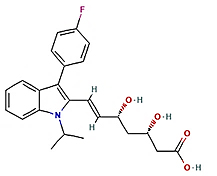
Figure 1:
2D representation of parent compound Fluvastatin.
Compounds | Formula | MW* | RB* | HBA* | HBD* | BBB* | BS* | RO5 | TPSA |
---|---|---|---|---|---|---|---|---|---|
control.pdb | C24H26FNO4 | 411.47 | 8 | 5 | 3 | No | 0.56 | Yes | 82.69 |
mol_000.pdb | C20H19FN2O4 | 370.37 | 7 | 5 | 3 | No | 0.56 | Yes | 91.56 |
mol_001.pdb | C21H22FNO5 | 387.4 | 6 | 6 | 4 | No | 0.56 | Yes | 102.92 |
mol_002.pdb | C21H22FNO3 | 355.4 | 6 | 4 | 2 | Yes | 0.85 | Yes | 62.46 |
mol_003.pdb | C21H22FNO5 | 387.4 | 6 | 6 | 3 | No | 0.56 | Yes | 98.07 |
mol_004.pdb | C21H22FNO5 | 387.4 | 6 | 6 | 4 | No | 0.56 | Yes | 102.92 |
mol_005.pdb | C21H22FNO5 | 387.4 | 10 | 6 | 3 | No | 0.56 | Yes | 103.7 |
mol_008.pdb | C21H22FNO3 | 355.4 | 6 | 4 | 2 | Yes | 0.85 | Yes | 62.46 |
mol_012.pdb | C21H22FNO4 | 371.4 | 6 | 5 | 3 | No | 0.56 | Yes | 82.69 |
mol_014.pdb | C21H22ClFO4 | 392.85 | 8 | 5 | 1 | No | 0.85 | Yes | 63.6 |
Parameters | ANALOGS | |||||||||
---|---|---|---|---|---|---|---|---|---|---|
Control | Mol_000 | Mol_001 | Mol_002 | Mol_003 | Mol_004 | Mol_005 | Mol_008 | Mol_012 | Mol_014 | |
Absorption | ||||||||||
Skin perm. (log mol/l) | -2.735 | -2.734 | -2.735 | -2.735 | -2.735 | -2.735 | -2.735 | -2.773 | -2.735 | -2.734 |
Water solubility (Log Kp) | -3.454 | -4.567 | -2.946 | -4.097 | -3.829 | -3,162 | -2.827 | -5.089 | -3.481 | -5.656 |
Distribution | ||||||||||
CNS perm. | -2.411 | -2.822 | -3.203 | -2.272 | -3.325 | -3.259 | -3.081 | -2.225 | -2.3 | -2.099 |
BBB perm. | -1.194 | -0.871 | -1.435 | 0.209 | -1.015 | -1.353 | -0.959 | -0.342 | -1.165 | -0.453 |
Metabolism | ||||||||||
CYP2D6 subs. | Yes | No | No | No | No | No | No | No | No | No |
CYP3A4 subs. | Yes | No | No | Yes | Yes | No | No | No | No | Yes |
CYP1A2 inh. | No | No | No | Yes | No | No | No | Yes | No | No |
CYP2C19 inh. | No | No | No | No | No | No | No | No | No | No |
CYP2C9 inh. | Yes | No | No | Yes | No | No | No | No | No | No |
CYP2D6 inh. | No | No | No | No | No | No | No | No | No | No |
CYP3A4 inh. | No | No | No | No | No | No | No | No | No | No |
Excretion | ||||||||||
Total clearance | 0.507 | 0.644 | 0.414 | 0.531 | 0.073 | 0.334 | 0.26 | 0.586 | 0.4 | -0.031 |
RenalOCT2 subs. | No | No | No | No | No | No | No | No | No | No |
Toxicity | ||||||||||
AMES | No | No | No | No | No | No | No | No | No | No |
hERG I inhibitor | No | No | No | No | No | No | No | No | No | No |
hERG II inhibitor | No | No | No | No | No | No | No | No | No | No |
Hepatotoxicity | Yes | Yes | Yes | Yes | Yes | Yes | Yes | Yes | Yes | No |
Principal component analysis in protein dynamics
Principal Component Analysis (PCA) offers valuable insights into the overall behavior of a protein during various simulations (Maisuradzeet al.,2009). Specifically, it provides information on a protein’s large-scale average motion and exposes the underlying structures and atomic fluctuations (David and Jacobs, 2014). In the context of the galaxy framework, PCA was used to explore conformational changes induced by complex interactions between proteins and drugs (Khanet al., 2023). The 10 ns trajectory of the targeted protein and selected compounds was carefully analyzed to emphasize the parameters and conformations (Patelet al., 2024).
RESULTS
Ligands library
Fluvastatin, the parent compound, was used by the MolOpt webserver to generate diverse analogs with improved pharmacokinetic profiles. To achieve this, bioisosteric replacement sites were identified, resulting in 194 derivatives. Information for these compounds was obtained from a previous study. CSV file for subsequent molecular docking analysis. Figure 1 illustrates the chemical structure of fluvastatin as shown in a 2D representation.
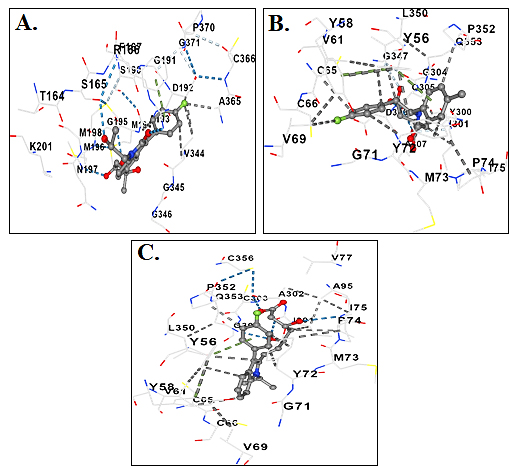
Figure 2:
3-dimensional molecular structures surrounded with Interacting amino acid residues. A: Compound 03 and B: Compound 05 and C: Control.
Compounds | Cardio protectant | Cardiotonic | Cholesterol absorption inhibitor | Cholesterol antagonist | Anti-hyper cholesterolemic | Anti-inflammatory | ||||||
---|---|---|---|---|---|---|---|---|---|---|---|---|
Pa | Pi | Pa | Pi | Pa | Pi | Pa | Pi | Pa | Pi | Pa | Pi | |
control.pdb | 0,286 | 0,094 | 0,223 | 0,143 | 0,038 | 0,038 | NA | NA | 0,248 | 0,080 | 0,283 | 0,084 |
mol_000.pdb | NA | NA | NA | NA | NA | NA | NA | NA | 0,155 | 0,148 | 0,252 | 0,210 |
mol_001.pdb | NA | NA | NA | NA | NA | NA | NA | NA | NA | NA | NA | NA |
mol_002.pdb | 0,204 | 0,183 | NA | NA | NA | NA | 0,215 | 0,197 | 0,215 | 0,102 | 0,409 | 0,091 |
mol_003.pdb | 0,273 | 0,106 | 0,250 | 0,115 | 0,043 | 0,019 | 0,308 | 0,103 | NA | NA | NA | NA |
mol_004.pdb | 0,246 | 0,131 | NA | NA | NA | NA | 0,265 | 0,138 | NA | NA | NA | NA |
mol_005.pdb | NA | NA | NA | NA | NA | NA | 0,267 | 0,136 | 0,395 | 0,038 | NA | NA |
mol_008.pdb | NA | NA | NA | NA | NA | NA | 0,308 | 0,103 | 0,289 | 0,060 | 0,359 | 0,117 |
mol_012.pdb | 0,265 | 0,113 | NA | NA | NA | NA | 0,277 | 0,127 | NA | NA | NA | NA |
mol_014.pdb | NA | NA | NA | NA | NA | NA | NA | NA | 0,392 | 0,039 | 0,473 | 0,065 |
Virtual screening of derivatives
The DockThor web server, a flexible docking tool, was used to determine the binding energies of the 194 derivatives. This method involves assigning unique ligand identities and predicting the binding energy, taking into account additional intermolecular interaction energy, which encompasses the sum of van der Waals and electrostatic energy, van der Waals energy and electrostatic energy. A range of docking scores was recorded for different derivatives, with values ranging from -8.30 to -6.06 kcal/mol. The top nine molecules, exhibiting superior binding energies and interactions, were selected along with the control compound for further evaluation (Supplementary Table 1).
Drug-likeness profiling and ADMET evaluation
The investigation of absorption, desorption, metabolism, excretion and toxicity properties was conducted with great care using the ADMET profile, which is of great importance in drug discovery (Vardhan and Sahoo, 2020). The properties of the chosen compounds were thoroughly assessed to determine their drug-likeness and it was observed that all of them met the ADME criteria and adhered to Lipinski’s rule (Supplementary Table 2). Furthermore, Swiss ADME conducted an extensive examination of the ADME profile (Haneeet al., 2021) Beyond drug-likeness parameters, other molecular properties, including skin permeability, various metabolic parameters, AMES and hepatotoxicity, were uncovered using the pkCSM tool, which is used for toxicity profiling (Chenet al., 2005). All parameters of the selected compounds were predicted and analyzed with precision (Supplementary Table 3).
PASS prediction spectrum analysis
The online tool way2 drug was utilized to examine the selected compounds through PASS prediction. This examination was showed in accordance with the procedures set forth (Filimonovet al., 2014). In their inactive state, the chosen medicinal compounds exhibited lipid-related features, including cardioprotectant, cardiotonic, cholesterol absorption inhibitor, antagonist, anti-hypercholesterolemic and anti-inflammatory properties, which were assessed for their risk of cardiovascular disease (Kimballet al., 2019). The PASS prediction involves determining the likelihood of active and inactive compounds, as outlined (Islamet al., 2022). The primary goal of the PASS prediction was to thoroughly analyze the parameters and ensure that each compound demonstrated a Pa value greater than Pi, as shown in (Supplementary Table 4).
Assessment of acute rat toxicity and side effects
All compounds were subjected to a thorough evaluation of acute toxicity in rats, as shown in (Supplementary Table 5). Acute rat toxicity in rodent models was predicted using the GUSAR database. It is crucial to assess potential adverse side effects in the pursuit of lead candidacy. The Adver-pred web service was employed to analyze the compounds and the outcomes are reported along with the values of Pa and Pi, as presented in (Supplementary Table 6).
This study focused on the comprehensive analysis of mol-003 and mol-005, two compounds that demonstrated non-toxic properties, as well as negative AMES results and no inhibition of hERG channels or hepatotoxicity. These findings indicated that these compounds have minimal side effects. The interaction details and interacting residues of these compounds are provided in (Supplementary Table 7) and Figure 2, respectively.
Molecular Dynamic Simulation
Molecular Dynamics (MD) simulations were executed for a period of 10 ns using the GROMACS setup with the aim of evaluating stability and gaining insights into the identified hits. To comprehensively analyze the stability of the complexes comprising ligands and proteins, several trajectories were incorporated (Tariqueet al., 2017). Plots, including Root Mean Square Deviation (RMSD), Root Mean Square Fluctuation (RMSF), Solvent Accessible Surface Area (SASA), and Radius of gyration (Rg), were generated to assess the stability of ligand-protein interactions (Khanet al., 2022).
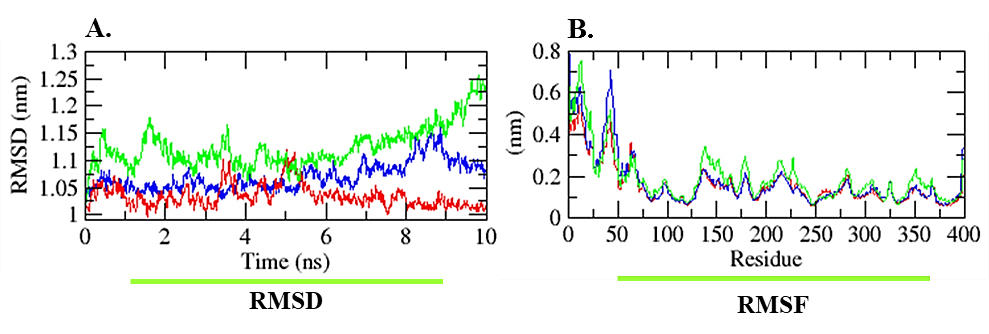
Figure 3:
Time-dependent (A) RMSD and (B) RMSF plots as function of time obtained for compound 03 as red color, blue color represented as compound 05, while the green color represented the control.
Rodent Parameters of Compounds | ||||||||||
---|---|---|---|---|---|---|---|---|---|---|
Control | Mol_000 | Mol_001 | Mol_002 | Mol_003 | Mol_004 | Mol_005 | Mol_008 | Mol_012 | Mol_014 | |
Units/Class | (Rat IP LD50 Log 10) | |||||||||
(mmol/kg) | -0,460 in AD | -0,460 in AD | -0,460 in AD | -0,460 in AD | -0,460 in AD | -0,460 in AD | -0,460 in AD | -0,460 in AD | -0,460 in AD | -0,460 in AD |
(mg/kg) | 147,600 in AD | 147,600 in AD | 147,600 in AD | 147,600 in AD | 147,600 in AD | 147,600 in AD | 147,600 in AD | 147,600 in AD | 147,600 in AD | 147,600 in AD |
CLASS | IV | IV | IV | IV | IV | IV | IV | IV | IV | IV |
(Rat IV LD50 Log 10) | ||||||||||
(mmol/kg) | -0,508 in AD | -0,508 in AD | -0,508 in AD | -0,508 in AD | -0,508 in AD | -0,508 in AD | -0,508 in AD | -0,508 in AD | -0,508 in AD | -0,508 in AD |
(mg/kg) | 132,000 in AD | 132,000 in AD | 132,000 in AD | 132,000 in AD | 132,000 in AD | 132,000 in AD | 132,000 in AD | 132,000 in AD | 132,000 in AD | 132,000 in AD |
CLASS | IV | IV | IV | IV | IV | IV | IV | IV | IV | IV |
Rat oral LD50 Log 10 | ||||||||||
(mmol/kg) | -0,133 in AD | -0,133 in AD | -0,133 in AD | -0,133 in AD | -0,133 in AD | -0,133 in AD | -0,133 in AD | -0,133 in AD | -0,133 in AD | -0,133 in AD |
(mg/kg) | 313,700 in AD | 313,700 in AD | 313,700 in AD | 313,700 in AD | 313,700 in AD | 313,700 in AD | 313,700 in AD | 313,700 in AD | 313,700 in AD | 313,700 in AD |
CLASS | IV | IV | IV | IV | IV | IV | IV | IV | IV | IV |
Rat SC LD50 Log 10 | ||||||||||
(mmol/kg) | -0,542 in AD | -0,542 in AD | -0,542 in AD | -0,542 in AD | -0,542 in AD | -0,542 in AD | -0,542 in AD | -0,542 in AD | -0,542 in AD | -0,542 in AD |
(mg/kg) | 122,200 in AD | 122,200 in AD | 122,200 in AD | 122,200 in AD | 122,200 in AD | 122,200 in AD | 122,200 in AD | 122,200 in AD | 122,200 in AD | 122,200 in AD |
CLASS | III | III | III | III | III | III | III | III | III | III |
Root-Mean-Square Deviation (RMSD) and Fluctuation (RMSF) analysis
To evaluate the reliability of the protein, RMSD and RMSF analyses were conducted over a 10 ns MD trajectory. Lower values indicate greater stability. Specifically, RMSD was analyzed for the protein backbone and ligand, revealing the higher reliability of compound 03 with a value of 1.03 nm, while the other hit showed a value of 1.07 and the control exhibited a value of 1.12 nm (Kausaret al., 2022). RMSF analysis depicted how protein residues fluctuated during ligand binding throughout progression (Ghahremanianet al., 2022). Notably, from the RMS-fluctuation analysis, minimum deviations were observed for compound 03, demonstrating its superior stability. The RMSF values for both compounds were recorded as 0.16 nm and 0.17, respectively, compared to the control’s 0.19. Plots of RMSD and RMSF are revealed in Figure 3.
Quantification of compactness and SASA Determination
The Radius of Gyration (Rg) was determined to evaluate fluctuations in protein compactness during the simulation. This method provides a profile that illustrates the uniformity of the protein throughout the process. The root-mean-square average of the broken distances showed the overall information content of the protein to a greater extent. This study also revealed the size of the macromolecule; with smaller values indicating greater compactness (Figure 4A) (Ahmad Khan and Almarshad, 2023). The Rg values were noted to be 2.48 nm and 2.53 nm for both compounds, compared to the control at 2.57 nm.
A Solvent-Accessible Surface Area (SASA) representation was used to explore the stability and conformational changes after ligand binding. This approach provided in-depth insights from the 10 ns trajectory of all hits, including the control drug. A higher SASA value indicates that the protein structure has expanded, allowing for increased solvent access to a larger surface area (Rathod, 2021). The mean SASA alone does not fully convey the impact of ligand binding on stability, necessitating careful analysis of the trajectory during the simulation. The mean values for compounds 03 and 05 were 26.51 nm² and 26.55 nm², respectively (Figure 4B).
Compounds | Pa | Pi | Side Effects |
---|---|---|---|
Control.pdb | 0.979 | 0.007 | Hepatotoxicity |
0.94 | 0.005 | Nephrotoxicity | |
mol_000.pdb | 0.776 | 0.069 | Hepatotoxicity |
0.576 | 0.032 | Myocardial infarction | |
0.547 | 0.036 | Cardiac failure | |
0.453 | 0.065 | Nephrotoxicity | |
mol_001.pdb | 0.4 | 0.07 | Myocardial infarction |
0.333 | 0.126 | Nephrotoxicity | |
0.289 | 0.184 | Cardiac failure | |
mol_002.pdb | 0.779 | 0.067 | Hepatotoxicity |
0.511 | 0.05 | Cardiac failure | |
0.489 | 0.045 | Myocardial infarction | |
0.444 | 0.068 | Nephrotoxicity | |
mol_003.pdb | 0.394 | 0.088 | Nephrotoxicity |
mol_004.pdb | 0.445 | 0.067 | Nephrotoxicity |
0.29 | 0.224 | Myocardial infarction | |
0.273 | 0.199 | Cardiac failure | |
mol_005.pdb | 0.755 | 0.078 | Hepatotoxicity |
0.284 | 0.175 | Nephrotoxicity | |
mol_008.pdb | 0.752 | 0.079 | Hepatotoxicity |
0.44 | 0.069 | Nephrotoxicity | |
0.412 | 0.099 | Cardiac failure | |
0.373 | 0.087 | Myocardial infarction | |
mol_012.pdb | 0.377 | 0.205 | Arrhythmia |
0.345 | 0.118 | Nephrotoxicity | |
0.33 | 0.322 | Hepatotoxicity | |
0.298 | 0.203 | Myocardial infarction | |
mol_014.pdb | 0.526 | 0.182 | Hepatotoxicity |
0.465 | 0.061 | Nephrotoxicity | |
0.354 | 0.128 | Cardiac failure | |
0.323 | 0.157 | Myocardial infarction | |
0.979 | 0.007 | Hepatotoxicity |
Principal component analysis for stability assessment
The application of Principal Component Analysis (PCA) in this study expected to evaluate and equivalence the trajectories of docked complexes by simplifying the complexity while preserving the maximum variance. The extent of diversity delineated by PC1 for compounds 1 and 2 was remarkably similar, representing 22.1% and 22.6% of that of compound 2, respectively. This finding suggested that the pathways associated with each protein were comparable. By contrast, the variance in the control group was 37.8%. It is widely acknowledged that lower variance proportions in PCA plots indicate greater stability. A visual representation of the plots is shown in Figure 5A-C. The effectiveness of PCA lies in its capacity to produce Principal Components, which are small variables based on variance estimation theory. These components capture the maximum variance in the original data.
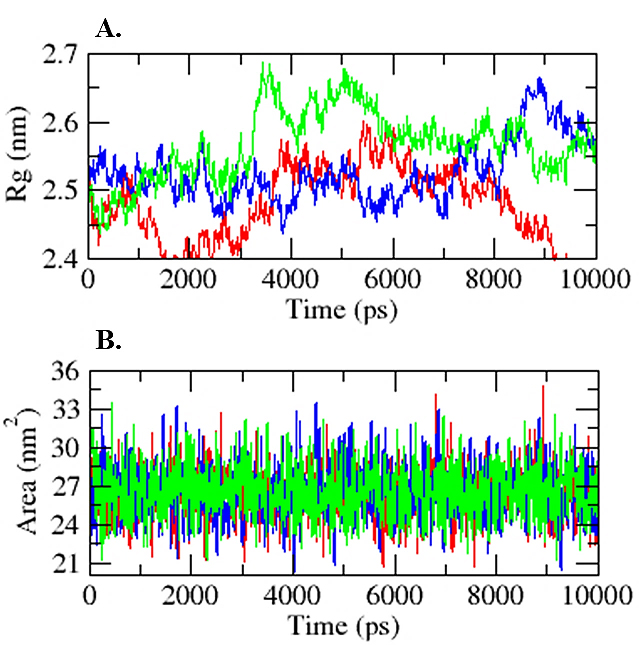
Figure 4:
(A) Time-dependent Radius of Gyration graph. the red line corresponds to Compound 03, the blue line represents Compound 05 and the green line denotes the control. (B) SASA analysis. red color represents compound 03, Blue color represented as compound 05 while the green color represents the control.
Compounds | Interacting Residues |
---|---|
Control | TYR56 TYR58 VAL61 CYS65 CYS66 VAL69 GLY71 TYR72 MET73 PRO74 ILE75 |
VAL77 ALA95 ILE301 ALA302 CYS303 GLY304 LEU350 PRO352 GLN353 | |
03 | LEU51 ARG54 ASP55 TYR56 TYR58 VAL61 CYS66 VAL69 ILE70 GLY71 TYR72 MET73 PRO74 ILE75 VAL77 ALA95 |
TYR300 ILE301 ALA302 CYS303 GLY304 GLN305 ASP306 ALA307 GLY347 LEU350 PRO352 GLN353 CYS356 | |
05 | TYR56 TYR58 VAL61 CYS66 VAL69 GLY71 TYR72 MET73 PRO74 TYR300 ILE301 |
GLY304 GLN305 ALA307 GLY347 LEU350 PRO352 GLN353 |
DISCUSSION
The focus of our study was Cardiovascular Disease (CVD), specifically dyslipidemia. Traditionally, statins have been considered as the primary treatment option, demonstrating efficacy in reducing the risk of major vascular events by lowering LDL-C levels. However, our investigation aimed to enhance efficacy and overcome drug resistance by developing derivatives of the well-known first-line treatment, fluvastatin, using the web-based server MolOpt. The resulting dataset underwent molecular docking for screening and the screened compounds, detailed in (Supplementary Table ), were subjected to further analyses.
The drug-likeness and ADME profiles, as outlined in (Supplementary Tables 2 and 3), provided comprehensive information. Notably, all compounds adhered to the Rule of Five (RO5), while compounds 02 and 08 exhibited Blood-Brain Barrier (BBB) penetration. For pharmacological impact, the PASS prediction indicated broad-ranging properties, with compounds 03 and 05 standing out for having more features than the rest. Subsequent evaluations using the GUSAR and ADVER-PRED databases have provided insights into acute rodent toxicity and adverse effects. This additional filtration reinforced the prominence of compounds 03 and 05, which demonstrated minimal side effects compared to the other compounds, including the control.
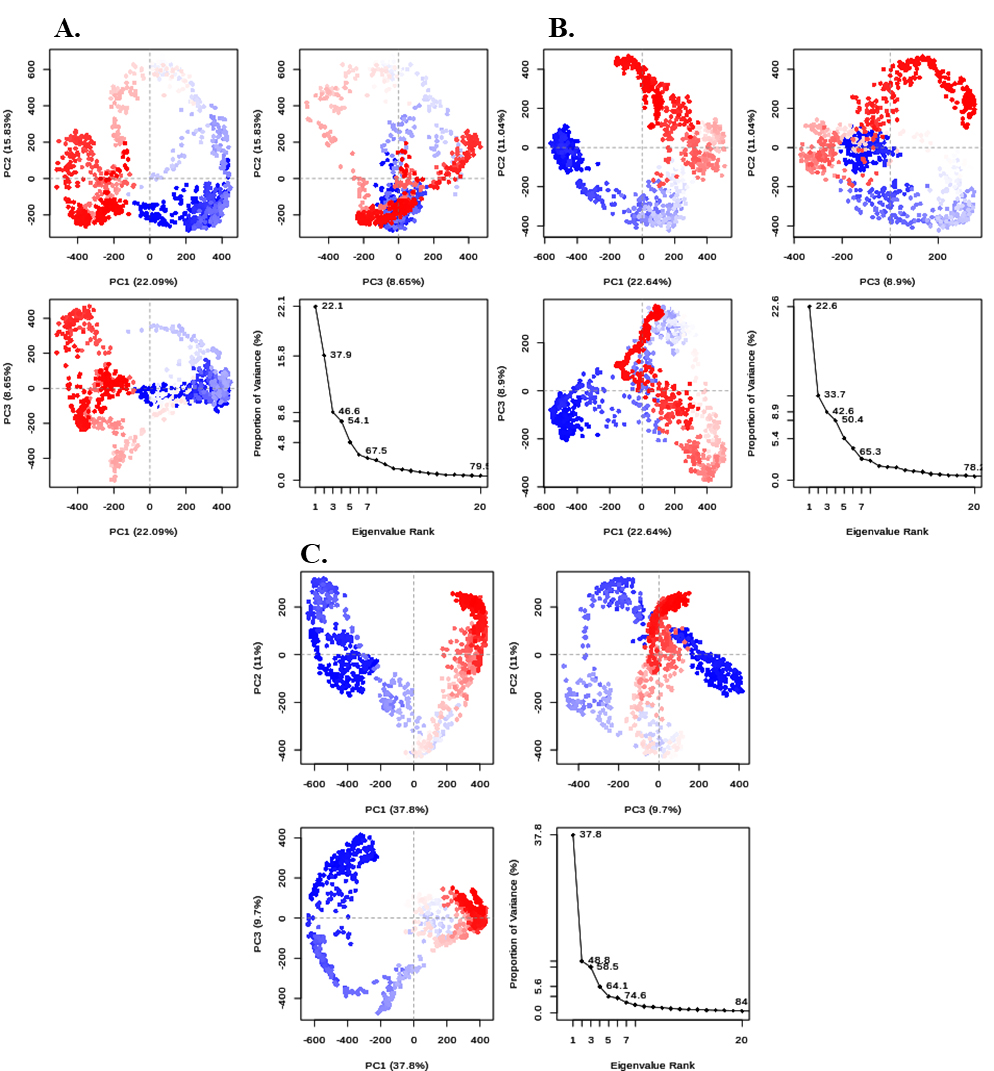
Figure 5:
(A) Graphic illustrations of the plot mol-003. (B) Plot for mol-005. (C) Plot of Control.
The refined selection of compounds, identified as promising candidates, underwent molecular dynamics simulation and principal component investigation for further scrutiny. Simulation studies encompassing root-mean-square deviations, root-mean-square fluctuations, solvent-accessible surface area, and Radius of Gyration revealed that both compounds, mol_03 and mol_05, outperformed the control compound, fluvastatin. Mol_03 exhibited superior stability, with an RMSD of 1.03 nm, which was lower than that of the parent compound and other derivatives. Fluctuation analysis indicated ranges of 0.16 nm and 0.17 nm for mol_03 and mol_05, respectively, compared to the parent compound’s value of 0.19 nm, as illustrated in Figure 3. The radius of gyration and Solvent-Accessible Surface Area (SASA) further supported this assessment, as shown in Figure 4A-B.
Additionally, principal component studies provided supporting evidence by showing lower variance for mol_03 and mol_05 compared with the control, as revealed in Figure 5A, B and C. Collectively, these findings suggest that mol_03 and mol_05 exhibit favorable characteristics in terms of stability and dynamics, making them hopeful candidates for additional investigation in cardiovascular disease treatment.
CONCLUSION
The development of novel drugs to address severe cardiac diseases has become a pressing concern that has garnered significant attention. In light of these challenges, our investigation highlights the promising potential of fluvastatin derivatives or analogs that exhibit anti-hypercholesterolemia, anti-cardiac effects and cholesterol inhibition, particularly in the context of diagnosing dyslipidemia within the spectrum of Cardiovascular Diseases (CVDs). Of all the molecules assessed, mol_03 emerged as the most promising candidate. This compound exhibited remarkable binding affinity, along with a lack of toxicity and only one negligible side effect, as evidenced by various parameters. To further explore this potential, MD simulation studies and Principal Component Analysis (PCA) were conducted. The results of the MD studies indicated lower fluctuations, whereas PCA served as a supporting pillar, reinforcing the robustness of the outcomes. In conclusion, mol_03, a derivative of fluvastatin, is a promising candidate for further development and optimization in the field of drug discovery. However, it is essential to emphasize the need for wet-lab verification to validate and solidify these findings before considering practical applications.
Cite this article:
Mahzari A, Khalifa EH, Zaeri AA, Alyahyawi HE, Alwanian WM, et al. Mechanistic Insight and Inhibitory Potential of Fluvastatin-Derivatives against Dyslipidemia. Int. J. Pharm. Investigation. 2025;15(2):10-8.
ACKNOWLEDGEMENT
We would like to sincerely thank the Department of Laboratory Medicine, Faculty of Applied Medical Sciences, Al-Baha University, Al-Baha, Kingdom of Saudi Arabia, for their invaluable support and resources provided throughout this study.
ABBREVIATIONS
LDL-C | Low-density lipoprotein cholesterol |
---|---|
HDL | High-density lipoprotein |
MD | Molecular dynamics |
PCA | Principal component analysis |
CVDs | Cardiovascular diseases |
DM | Diabetes mellitus |
TC | Total cholesterol |
RMSD | Root Mean Square Deviation |
RMSF | Root Mean Square Fluctuation |
SASA | Solvent Accessible Surface Area |
Rg | Radius of Gyration |
BBB | Blood-brain barrier |
References
- Ahmad Khan M. K., Almarshad F.. (2023) Computational approaches for lead discovery against SARS-CoV-2 3C-like protease: Virtual screening and Molecular Dynamics studies. Journal of Medicine and Health Studies : 49-62 Google Scholar
- Anderson T. J., Grégoire J., Pearson G. J., Barry A. R., Couture P., Dawes M., Francis G. A., Genest J., Grover S., Gupta M., Hegele R. A., Lau D. C., Leiter L. A., Lonn E., Mancini G. B. J., McPherson R., Ngui D., Poirier P., Sievenpiper J. L., Ward R., et al. (2016) 2016 Canadian Cardiovascular Society guidelines for the management of dyslipidemia for the prevention of cardiovascular disease in the adult. The Canadian Journal of Cardiology 32: 1263-1282 Google Scholar
- Azzam K. A.. (2023) SwissADME and pkCSM Webservers Predictors: An integrated Online Platform for Accurate and Comprehensive Predictions for in silico ADME/T Properties of artemisinin and its Derivatives. Kompleksnoe Ispol′zovanie Mineral′nogo syr′â/ Complex Use of Mineral Resources/Mineraldik Shikisattardy Keshendi Paidalanu 325: 14-21 Google Scholar
- Brenk R., Schipani A., James D., Krasowski A., Gilbert I. H., Frearson J., Wyatt P. G., et al. (2008) Lessons learnt from assembling screening libraries for drug discovery for neglected diseases. ChemMedChem 3: 435-444 Google Scholar
- Brown C. D., Higgins M., Donato K. A., Rohde F. C., Garrison R., Obarzanek E., Ernst N. D., Horan M., et al. (2000) Body mass index and the prevalence of hypertension and dyslipidemia. Obesity Research 8: 605-619 Google Scholar
- Chen J., Swamidass S. J., Dou Y., Bruand J., Baldi P.. (2005) ChemDB: A public database of small molecules and related chemoinformatics resources. Bioinformatics 21: 4133-4139 Google Scholar
- Mihaylova B., Emberson J., Blackwell L., Keech A., Simes J., Barnes E. H., Voysey M., Gray A., Collins R., Baigent C., et al. (2012) The Effects of lowering LDL cholesterol with statin therapy in people at low risk of vascular disease: Meta-analysis of individual data from 27 randomised trials. The Lancet 380: 581-590 Google Scholar
- Daina A., Michielin O., Zoete V.. (2017) SwissADME: A free web tool to evaluate pharmacokinetics, drug-likeness and medicinal chemistry friendliness of small molecules. Scientific Reports 7: 42717 Google Scholar
- David C. C., Jacobs D. J.. (2014) Principal component analysis: A method for determining the essential dynamics of proteins : 193-226 Google Scholar
- De Magalhães C. S., Almeida D. M., Barbosa H. J. C., Dardenne L. E.. (2014) A dynamic niching genetic algorithm strategy for docking highly flexible ligands. Information Sciences 289: 206-224 Google Scholar
- Després J.-P., Lemieux I., Bergeron J., Pibarot P., Mathieu P., Larose E., Rodés-Cabau J., Bertrand O. F., Poirier P., et al. (2008) Abdominal obesity and the metabolic syndrome: Contribution to global cardiometabolic risk. Arteriosclerosis, Thrombosis, and Vascular Biology 28: 1039-1049 Google Scholar
- Dick A., Cocklin S.. (2020) Bioisosteric replacement as a tool in anti-HIV drug design. Pharmaceuticals 13: 36 Google Scholar
- Dmitriev A. V., Filimonov D. A., Rudik A. V., Pogodin P. V., Karasev D. A., Lagunin A. A., Poroikov V. V., et al. (2019) Drug–drug interaction prediction using pass. SAR and QSAR in Environmental Research 30: 655-664 Google Scholar
- Domínguez-Villa F. X., Durán-Iturbide N. A., Ávila-Zárraga J. G.. (2021) Synthesis, molecular docking and in silico ADME/Tox profiling studies of new 1-aryl-5- (3-azidopropyl)indol-4-ones: Potential inhibitors of SARS CoV-2 main protease. Bioorganic Chemistry 106: 104497 Google Scholar
- Filimonov D. A., Lagunin A. A., Gloriozova T. A., Rudik A. V., Druzhilovskii D. S., Pogodin P. V., Poroikov V. V., et al. (2014) Prediction of the biological activity spectra of organic compounds using the pass online web resource. Chemistry of Heterocyclic Compounds (NY) 50: 444-457 Google Scholar
- Fischer N. M., Van Maaren P. J., Ditz J. C., Yildirim A., Van der Spoel D.. (2015) Properties of organic liquids when simulated with long-range Lennard-Jones interactions. Journal of Chemical Theory and Computation 11: 2938-2944 Google Scholar
- Ghahremanian S., Rashidi M. M., Raeisi K., Toghraie D.. (2022) Molecular dynamics simulation approach for discovering potential inhibitors against SARS-CoV-2: A structural review. Journal of Molecular Liquids 354: 118901 Google Scholar
- Grant B. J., Rodrigues A. P. C., ElSawy K. M., McCammon J. A., Caves L. S. D.. (2006) Bio3d: An R package for the comparative analysis of protein structures. Bioinformatics 22: 2695-2696 Google Scholar
- Grundy S. M., Stone N. J.. (2019) American Heart Association/American College of Cardiology multisociety guideline on the management of blood cholesterol. JAMA Cardiology 4: 488-489 Google Scholar
- Guzzaloni G., Grugni G., Minocci A., Moro D., Morabito F.. (2000) Liver steatosis in juvenile obesity: Correlations with lipid profile, hepatic biochemical parameters and glycemic and insulinemic responses to an oral glucose tolerance test. International Journal of Obesity and Related Metabolic Disorders 24: 772-776 Google Scholar
- Hadaegh F., Harati H., Ghanbarian A., Azizi F.. (2006) Association of total cholesterol versus other serum lipid parameters with the short-term prediction of cardiovascular outcomes: Tehran Lipid and glucose Study. European Journal of Cardiovascular Prevention and Rehabilitation 13: 571-577 Google Scholar
- Hanee U., Rahman M., Matin M.. (2021) Synthesis, PASS, in silico ADMET and thermodynamic studies of some galactopyranoside esters. Physical Chemistry Research 9: 591-603 Google Scholar
- Hedayatnia M., Asadi Z., Zare-Feyzabadi R., Yaghooti-Khorasani M., Ghazizadeh H., Ghaffarian-Zirak R., Nosrati-Tirkani A., Mohammadi-Bajgiran M., Rohban M., Sadabadi F., Rahimi H.-R., Ghalandari M., Ghaffari M.-S., Yousefi A., Pouresmaeili E., Besharatlou M.-R., Moohebati M., Ferns G. A., Esmaily H., Ghayour-Mobarhan M., et al. (2020) Dyslipidemia and cardiovascular disease risk among the MASHAD study population. Lipids in Health and Disease 19: 42 Google Scholar
- Islam S., Hosen M. A., Ahmad S., ul Qamar M. T., Dey S., Hasan I., Fujii Y., Ozeki Y., Kawsar S. M. A., et al. (2022) Synthesis, antimicrobial, anticancer activities, PASS prediction, molecular docking, molecular dynamics and pharmacokinetic studies of designed methyl α-D-glucopyranoside esters. Journal of Molecular Structure 1260: 132761 Google Scholar
- Ivanov S. M., Lagunin A. A., Rudik A. V., Filimonov D. A., Poroikov V. V.. (2018) ADVERPred-Web service for prediction of adverse effects of drugs. Journal of Chemical Information and Modeling 58: 8-11 Google Scholar
- Jia Z.-J., Lan X.-W., Lu K., Meng X., Jing W.-J., Jia S.-R., Zhao K., Dai Y.-J., et al. (2023) Synthesis, molecular docking and binding Gibbs free energy calculation of β-nitrostyrene derivatives: Potential inhibitors of SARS-CoV-2 3CL protease. Journal of Molecular Structure 1284: 135409-135409 Google Scholar
- Joshi S. R., Anjana R. M., Deepa M., Pradeepa R., Bhansali A., Dhandania V. K., Joshi P. P., Unnikrishnan R., Nirmal E., Subashini R., Madhu S. V., Rao P. V., Das A. K., Kaur T., Shukla D. K., Mohan V., et al. (2014) Prevalence of dyslipidemia in urban and rural India: The ICMR-INDIAB study. PLOS ONE 9: e96808 Google Scholar
- Kausar M. A., Shahid S., Anwar S., Kuddus M., Khan M. K. A., Alotaibi A. D., Arif J. M., et al. (2022) Identifying natural therapeutics against diabetes via inhibition of dipeptidyl peptidase 4: Molecular docking and MD simulation study. Indian Journal of Pharmaceutical Education and Research 56: s21-s31 Google Scholar
- Khan M. K. A., Ahmad S., Rabbani G., Shahab U., Khan M. S.. (2022) Target-based virtual screening, computational multiscoring docking and molecular dynamics simulation of small molecules as promising drug candidate affecting kinesin-like protein KIFC1. Cell Biochemistry and Function 40: 451-472 Google Scholar
- Khan M. K. A., Alouffi S., Ahmad S.. (2023) Identifying potential inhibitors of C-X-C motif chemokine ligand10 against vitiligo: Structure-based virtual screening, molecular dynamics simulation and principal component analysis. Journal of Biomolecular Structure and Dynamics : 1-18 Google Scholar
- Khan S., Khan F. I., Mohammad T., Khan P., Hasan G. M., Lobb K. A., Islam A., Ahmad F., Imtaiyaz Hassan M., et al. (2018) Exploring molecular insights into the interaction mechanism of cholesterol derivatives with the Mce4A: A combined spectroscopic and molecular dynamic simulation studies. International Journal of Biological Macromolecules 111: 548-560 Google Scholar
- Kimball S. M., Mirhosseini N., Rankin B. D., Fyie K., Guarneri M.. (2019) Retrospective analysis of cardiovascular disease risk parameters in participants of a preventive health and wellness program. Integrative Medicine 18: 78-95 Google Scholar
- Klop B., Elte J. W. F., Cabezas M. C.. (2013) Dyslipidemia in obesity: Mechanisms and potential targets. Nutrients 5: 1218-1240 Google Scholar
- Lagunin A., Zakharov A., Filimonov D., Poroikov V.. (2011) QSAR modelling of rat acute toxicity on the basis of PASS prediction. Molecular Informatics 30: 241-250 Google Scholar
- Langdon S. R., Ertl P., Brown N.. (2010) Bioisosteric replacement and scaffold hopping in lead generation and optimization. Molecular Informatics 29: 366-385 Google Scholar
- Liao J. K., Laufs U.. (2005) Pleiotropic effects of statins. Annual Review of Pharmacology and Toxicology 45: 89-118 Google Scholar
- Lipinski C. A.. (2004) Lead- and drug-like compounds: The rule-of-five revolution. Drug Discovery Today. Technologies 1: 337-341 Google Scholar
- Maisuradze G. G., Liwo A., Scheraga H. A.. (2009) Principal component analysis for protein folding dynamics. Journal of Molecular Biology 385: 312-329 Google Scholar
- Martirosyan D. M., Miroshnichenko L. A., Kulakova S. N., Pogojeva A. V., Zoloedov V. I.. (2007) Amaranth oil application for coronary heart disease and hypertension. Lipids in Health and Disease 6: 1 Google Scholar
- Mathers C. D., Loncar D.. (2006) Projections of global mortality and burden of disease from 2002 to 2030. PLOS Medicine 3: e442 Google Scholar
- Modee R., Mehta S., Laghuvarapu S., Priyakumar U. D.. (2023) MolOpt: Autonomous molecular geometry optimization using multiagent reinforcement learning. The Journal of Physical Chemistry. B 127: 10295-10303 Google Scholar
- Moghadasian M. H.. (1999) Clinical pharmacology of 3-hydroxy-3-methylglutaryl coenzyme A reductase inhibitors. Life Sciences 65: 1329-1337 Google Scholar
- Muegge I., Heald S. L., Brittelli D.. (2001) Simple selection criteria for drug-like chemical matter. Journal of Medicinal Chemistry 44: 1841-1846 Google Scholar
- O’Meara J. G., Kardia S. L. R., Armon J. J., Brown C. A., Boerwinkle E., Turner S. T., et al. (2004) Ethnic and sex differences in the prevalence, treatment and control of dyslipidemia among hypertensive adults in the GENOA study. Archives of Internal Medicine 164: 1313-1318 Google Scholar
- Ordovas J. M.. (2006) Genetic interactions with diet influence the risk of cardiovascular disease. The American Journal of Clinical Nutrition 83: 443S-446S Google Scholar
- Patel A. A., Alothaid H., Mallick A. K., Alalawy A. I., Mirdad R. T., Mirdad M. T., Mirdad M. T., Ahsan M., Tarique M., et al. (2024) Inhibiting cancer progression through targeting HDAC2 with novel ligands: A dynamic insights through virtual screening and simulation. Indian Journal of Pharmaceutical Education and Research 58: 802-813 Google Scholar
- Petersen H. G.. (1995) Accuracy and efficiency of the particle mesh Ewald method. The Journal of Chemical Physics 103: 3668-3679 Google Scholar
- Pires D. E. V., Blundell T. L., Ascher D. B. pkCSM.. (2015) pkCSM: Predicting small-molecule pharmacokinetic and toxicity properties using graph-based signatures. Journal of Medicinal Chemistry 58: 4066-4072 Google Scholar
- Rathod S. B.. (2021) Identification of PICK1 PDZ-Domain antagonists: Pharmacophore-based virtual screening, molecular docking and molecular dynamic simulation analyses. ChemRxiv Google Scholar
- Sadeghi M., Haghdoost A. A., Bahrampour A., Dehghani M.-S.. (2017) Modeling the burden of cardiovascular diseases in Iran from 2005 to 2025: The impact of demographic changes. Iranian Journal of Public Health 46: 506-516 Google Scholar
- Santos K. B., Guedes I. A., Karl A. L. M., Dardenne L. E.. (2020) Highly flexible ligand docking: Benchmarking of the dock Thor program on the LEADS-PEP protein-peptide data set. Journal of Chemical Information and Modeling 60: 667-683 Google Scholar
- Sikorski J. A.. (2007) Atherosclerosis/lipoprotein/cholesterol metabolism. In Comprehensive Medicinal Chemistry, II : 459-494 Google Scholar
- Singh R., Bhardwaj V. K., Sharma J., Das P., Purohit R.. (2021) Discovery and evaluation of aminoarylbenzosuberene molecules as novel checkpoint kinase 1 inhibitor determinants. Genomics 113: 707-715 Google Scholar
- Stenberg S., Stenqvist B.. (2020) An exact Ewald summation method in theory and practice. The Journal of Physical Chemistry. A 124: 3943-3946 Google Scholar
- Tarique M., Ahmad M., Chauhan M., Tuteja R.. (2017) Genome Wide in silico Analysis of the Mismatch Repair Components of Plasmodium falciparum and Their Comparison with Human Host. Frontiers in Microbiology 8: 130 Google Scholar
- Taylor F., Huffman M. D., Macedo A. F., Moore T. H. M., Burke M., Davey Smith G., Ward K., Ebrahim S., et al. (2013) Statins for the primary prevention of cardiovascular disease. The Cochrane Database of Systematic Reviews 2013: CD004816 Google Scholar
- Vardhan S., Sahoo S. K.. (2020) In silico ADMET and molecular docking study on searching potential inhibitors from limonoids and triterpenoids for COVID-19. Computers in Biology and Medicine 124: 103936 Google Scholar
- Wu T.-T., Gao Y., Zheng Y.-Y., Ma Y.-T., Xie X.. (2018) Atherogenic index of plasma (AIP): A novel predictive indicator for the coronary artery disease in postmenopausal women. Lipids in Health and Disease 17: 197 Google Scholar
- Yu H., Dalby P. A.. (2020) A beginner’s guide to molecular dynamics simulations and the identification of cross-correlation networks for enzyme engineering. Methods in Enzymology 643: 15-49 Google Scholar
- Zhang Y., Zhang T.-J., Tu S., Zhang Z.-H., Meng F.-H.. (2020) Identification of novel Src inhibitors: Pharmacophore-based virtual screening, molecular docking and Molecular Dynamics simulations. Molecules 25: 4094 Google Scholar